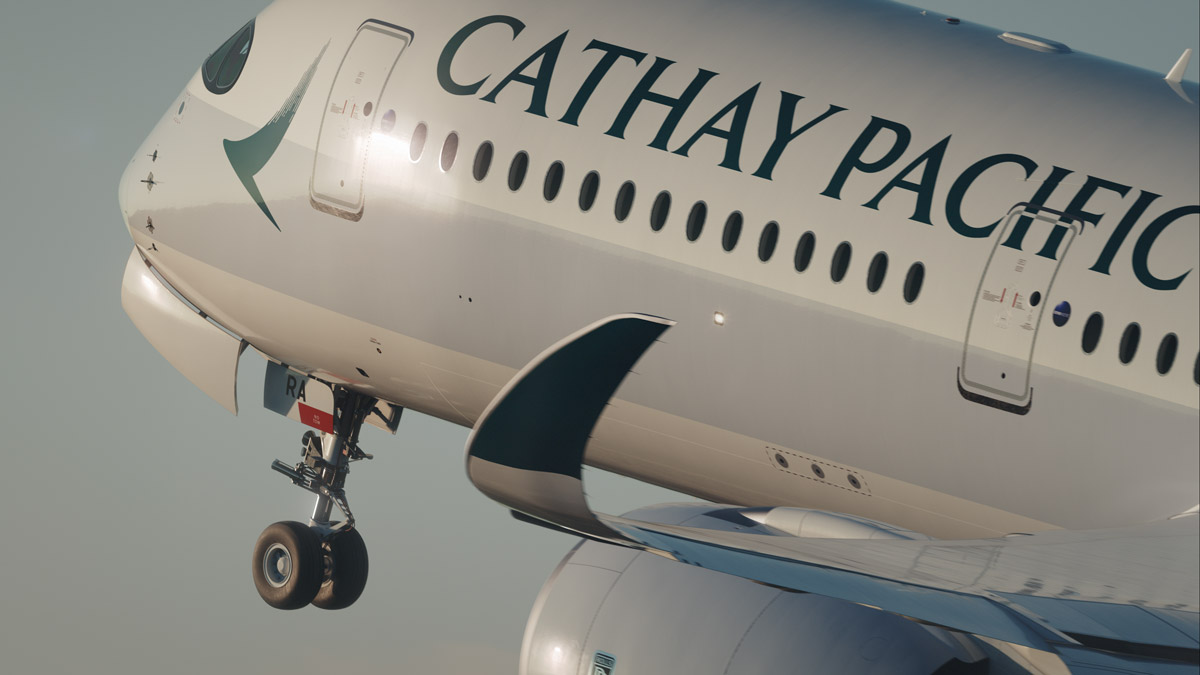
NB: Cathay Pacific
Aircraft fuel is without a doubt the biggest cost for any airline and often receives widespread attention, especially when airlines hedge their bets the wrong way. Cathay Pacific reported a HK$4.49 billion fuel-hedging loss in the first half of 2016, which has hurt the airline’s profitability.
The second biggest expense for an airline is human capital, and researchers from Hong Kong Polytechnic University and University of Nottingham Ningbo China Business School may have found a solution to ease some of Cathays financial woes through an unlikely source – Machine Learning and Data Science.
The researchers say that a “poorly designed airline crew schedule can result in unreliable flight schedules, significantly jeopardizing airline operations and profitability if insufficient crew members are available or other glitches occur. For that reason, managing airline crew scheduling and costs are one of the most crucial topics for airlines because it yields enormous economic benefits and ranks as the second highest expenditure after fuel costs.”
Departure delays can amount to a significant cost for the airline, and one of the often overlooked factors are that of cabin and cockpit crew. In the event of a long delay, it can affect the number of working hours for flight crew, potentially pushing them outside of maximum allowable flight duty period (FDP).
Airlines have built-in redundancy by rostering flight crew on standby, who can be called to operate a flight at short notice. Rostering on standby crew has a direct cost impact for the airline, and the researchers aimed to address in their study. Is it possible to predict how many standby crew need to be rostered on at any one time?
Utilizing Cathay Pacific flight data (April 2015 – March 2016) to develop their improved modeling for crew pairing, they used the data to conduct a series of experiments to get more accurate predictions about when flight arrivals would be delayed given the multiple factors that influence these delays.
Cascading Delay Risk of Airline Workforce Deployments
They used a model reflecting the many nonlinear factors to help define the best crew pairing. “A crew itinerary is called pairing, which is constructed by a set of duties, consisting of many flights, with sit-time and rest period in between as needed, according to the regulations,” the researchers explain. Crew pairing is an incredibly complex process by which airlines connect the legs of a flight so that the flight crew needed for each airplane is properly assigned and ends up where they live, or their crew base. Using such a data-driven approach to predict flight delays can help airlines generate more reliable crew schedules, which in turn can reduce passenger delays.
The researchers used a neural network (NN) approach to predict flight arrival delays to support the crew pairing optimization. NN is a promising approach to capture the nonlinear relationship between
various factors, as flight arrival delays are usually influenced by multiple factors.
Machine learning shows a promising future for airlines and could help solve major problems, but in general, the travel industry has been slow to adopt breakthrough advances in big data.
Cathay Pacific reported on-time performance of 64.7% in 2015.
Researchers Sai Ho Chung, Hoi Lam Ma, Hing Kai Chan. 2016. Cascading Delay Risk of Airline Workforce Deployments with Crew Pairing and Schedule Optimization. Risk Analysis. [In Press]. DOI: 10.1111/risa.12746 and quotes from Society for Risk Analysis.
Originally posted on TravelDataDaily