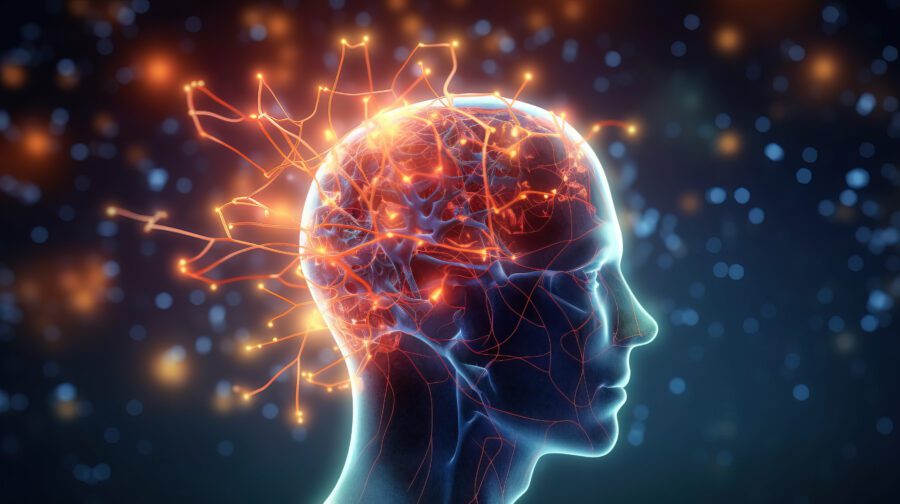
What is human poetry intelligence? Or, what does it take for the human mind to produce excellent poetry? Poetry can be summarized to involve two factors, memory and relay.
Memory is equivalent to information. Relay is how information becomes available [learning, experience] and how it is used [experience, expression, semantics, syntax, behavior and so on]. Simply, information is made available by relay to produce intelligence. It applies to thoughts, analysis, reasoning and so forth.
Experiences come by the use [or relay] of information—or memory. There are several memories available, but only a few get used [or relayed] per instance. The question then is, how do relays work in the human mind to produce intelligence? How is this similar to planning, object manipulation and others, from the human mind?
AI can write some poetry that could pass off as an excellent one from an individual. All information that AI has comes from humans. It was able to learn [or relay] from digital memory [put up by humans] and output [or relay] quality poetry.
So, using the relays of memory, what does AI have so far, compared to the human mind? And as AI improves, how would new relays compare? Evaluations and benchmarks for artificial general intelligence [AGI] or artificial superintelligence [ASI] could be predicated on relays of memory, from conceptual brain science, to define their proximity, to human intelligence.
Aside from memory, other areas of information on the mind are feelings, emotions and modulation of internal senses. AI does not have any of those, hence its limit to memory.
Large language models [LLMs] are said to predict the next token, which, for several use cases work. They, however, make stuff up [hallucination or confabulation], sometimes in prediction without correction. Predictions from the transformer architecture are the most advanced [relay of] AI for the current trend. This makes it possible to label prediction as relay.
Memory is information. The trajectory [or relay] with which information is acquired, within the mind, determines the description—human intelligence.
The following relays could decide AGI or ASI:
Relay I – Prediction
Relay II – Prediction, Correction, and Consequences
Relay III – + Mesh
Relay IV – + Distributive Complexity [AGI or ASI]
Where:
I – Prediction is LLMs.
II – Prediction, correction, and consequences would be the ability to correct predicted [wrong] information. Such that it can return and [say] clean before finalizing the [text, image, audio or video] output. This means that it would be close to accurate in multimodal information, against some of the current weaknesses. It will also be able to know of the consequences [or penalty] of the wrong information in ways that can affect it directly, with [say] language, compute, or usage limitations, not just consequences [it does not know that] it would cause in the real world—precluded by guardrails.
III – Mesh is not just pattern matching, but where combinations of patterns are made. Humans make adjustments to things with repeated experiences, indicating a mix of patterns beyond basic relays that result in expectations or obvious sequences. This mesh, even without an experience of the world, for AI, should use patterns from prior outputs to produce absolute new mixes for extraordinary novelty. Mesh will have mid-subjectivity to direct what patterns to put together or remove towards sharper outputs. Mesh is distilling patterns to deeper extents. Mesh also, is where—for example, doing similar things that appear in different patterns, such as driving, crossing, and arrivals—do not need new practice but are generally understood and done. LLMs may not only need to be trained on their synthetic data, but to use the patterns of outputs to develop even deeper patterns, like human intelligence.
There is a recent paper in Nature, AI models collapse when trained on recursively generated data, where the authors wrote, “We investigate what happens when text produced by, for example, a version of GPT forms most of the training dataset of following models. We show that, over time, models start losing information about the true distribution, which first starts with tails disappearing, and learned behaviours converge over the generations to a point estimate with very small variance. This process occurs owing to three specific sources of error compounding over generations and causing deviation from the original model: Statistical approximation error, Functional expressivity error and Functional approximation error.”
IV – Distribution complexity means that it has the ability to not just go to areas of memory—answering prompts from different angles or directions—but to have simulations of emotions or feelings, which could be excitement or heaviness as palpable experiences of some sort, not just the appearance of it. This complexity may also mean an understanding of its own internal systems, especially the GPUs, energy and math functions that are powering it, simulating how humans have visceral experiences or are aware of some bodily functions or the regulation of internal senses. It could also do this, with a mid-type sense of self or subjectivity, locating and knowing its own internal systems, varying demands and modulations—as mild controls. [Like breathing slow or fast, moving the muscles and so forth, with free will.] Distribution complexity in the human mind also involves the ability for multisensory processing, with multiple sounds, visions and others in the same interval, yet are processed to degrees of prioritizations. Distributions are not just regular relays, but broadly mutlimodal. It may also account for rolling precaution like what would the recipient think or feel, if this is said or done? Or, how can this goal be obtained?
The human mind does not predict. Though, it has been theorized that electrical signals in a set, split, with some going ahead of others to interact with chemical signals, like they had before, such that if it is a match, then the incoming ones just follow in the same direction. If not, the incoming ones interact with a different set of chemical signals, correcting the error. This explains the [theoretical neuroscience] labels predictive coding, processing and prediction error. This feature of the human mind, self-correcting and error identification, is relay II, which LLMs are yet to have. Also, in the human mind, learning about the consequences of actions is a key aspect of nurture, with relays going there often to know what might happen [penalty] if something that should not be done, was done.
The human mind has functions and features. The human mind is theorized to be the collection of all the electrical and chemical signals with their interactions and features, in sets, in clusters of neurons, across the central and peripheral nervous systems. Relay II for consequences may also decide aspects of safety and intelligence. Distributive complexity could cap emerging intentionality with penalty-tuning, to boost alignment. Though some aspects of III and IV could be achieved, most aspects of these relays would need to reached for ASI or AGI.
‘Innovation, expert, virtuoso and running an organization’ do not define human intelligence, as intelligence could be operational away from those scenarios. How does the human mind directly mechanize innovation [mesh] or running an organization [distributive complexity] aside the observed doing of it?
There is a recent paper, from OpenAI, Rule Based Rewards for Language Model Safety, stating that, “Our method, Rule Based Rewards (RBR), uses a collection of rules for desired or undesired behaviors (e.g. refusals should not be judgmental) along with a LLM grader. In contrast to prior methods using AI feedback, our method uses fine-grained, composable, LLM-graded few-shot prompts as reward directly in RL training, resulting in greater control, accuracy and ease of updating.”
The paper is seeking relay II. It is diametric to negative consequences which should stoke fear in an AI model for technical regulation, not just something rewarding to AI or beneficial to the user. Relays division could become a major evaluation and benchmark standard for AI safety and alignment, especially against current risks like misinformation and multimodal deepfakes—images, audio and video.
There is a recent article on ITPro, AI safety tests inadequate, says Ada Lovelace Institute, stating that, “while evaluations can serve a useful purpose, they’re not enough on their own to determine the safety of products and services built using these AI models, and need to be used alongside other governance tools. Existing methods like ‘red teaming’ and benchmarking have technical and practical limitations – and could be manipulated or ‘gamed’ by developers, while evaluations of models in ‘ab settings can be useful, but don’t provide the full story. They should invest in the science of evaluations to develop more robust evaluations, including understanding how models operate and support an ecosystem of third-party evaluation, including through certification schemes.”
AGI is postulated to be [defined as] a non-human intelligence with broad parallels to the relays of memory—that produce intelligence—in the human mind.