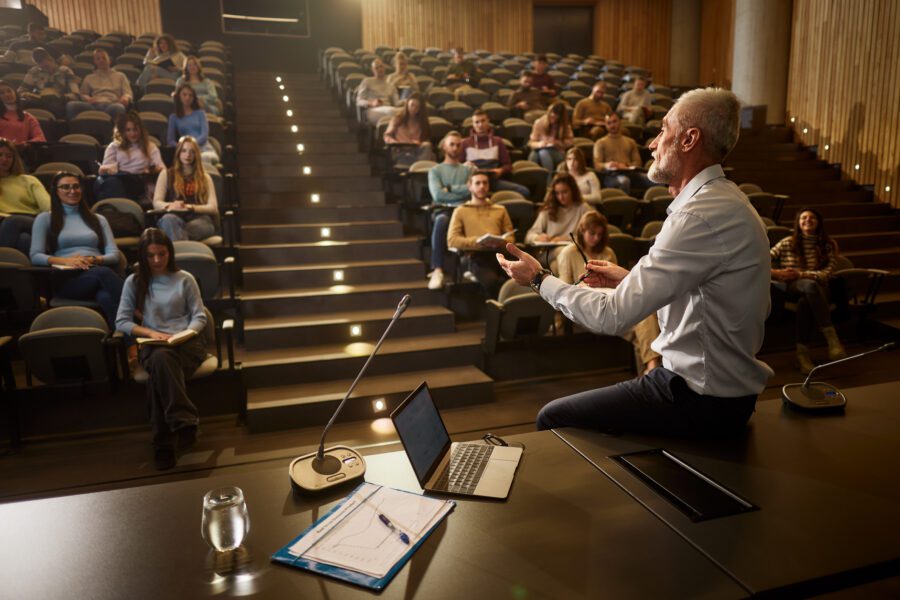
Predicting student performance is crucial for educational institutions aiming to enhance learning outcomes and provide timely support. Anticipating academic challenges and identifying at-risk pupils early allows schools to implement targeted interventions to improve success rates.
Machine learning is crucial in this predictive process. It leverages vast amounts of educational data to uncover patterns and insights traditional analysis methods might miss. This technology can improve student outcomes by making effective data-driven decisions.
How machine learning predicts student performance
Machine learning utilizes various data types to predict student performance, including grades, attendance records, participation metrics, socioeconomic background and behavioral information. Analysis ensures institutions direct resources toward the highest-impact opportunities, improving access and support for a broader range of learners.
The process begins with cleaning and preparing data to eliminate errors and inconsistencies. Developers identify study habits, engagement levels and prior academic performance. Then, they use algorithms — like decision trees and neural networks — to build predictive models. These structures enable them to make informed decisions and enhance student outcomes.
Pros of using machine learning to predict student performance
Machine learning offers numerous advantages when predicting student performance. Here’s how it can significantly improve educational experiences and outcomes.
1. Early intervention
Using machine learning to predict student performance enables educators to identify at-risk students early. This allows schools to provide timely support and allocate resources effectively, addressing issues before they become critical.
Recognizing patterns that indicate potential academic struggles allows machine learning to help educators intervene with personalized strategies and assistance. It improves student outcomes and ensures they receive the attention they need to succeed.
2. Personalized learning
Machine learning tailors educational experiences to individual needs, which creates a personalized environment and boosts student motivation and engagement levels. Analyzing everyone’s strengths, weaknesses and learning preferences means these systems can customize lesson plans and educational content to suit their unique requirements.
This personalized approach makes learning more relevant and accessible and keeps students engaged and motivated. Kids who see institutions designing curriculums specifically for them are more likely to participate actively and take ownership of their educational journey.
3. Improved academic outcomes
Machine learning provides data-driven strategies for boosting student achievement, enabling educators to implement effective interventions based on solid evidence. For instance, pupils who complete their assigned tasks demonstrate a 3.4% increase in persistence rates compared to those who don’t, showcasing the impact of targeted support.
Leveraging these insights allows schools to develop strategies that enhance student performance and ensure educational practices are grounded in empirical data. This approach increases individual success rates and creates a more effective and supporting learning environment for all.
Cons of using machine learning to predict student performance
While machine learning offers benefits in predicting student performance, it also has several challenges that need careful consideration. Understanding these drawbacks ensures the practical and ethical application of using it in education.
1. Dependence on data quality
The quality of machine learning models’ predictions depends on the information they are trained on. Ensuring high data quality is not a one-time activity. Organizations must continuously monitor, measure and improve their practices to maintain accurate and reliable results.
Inaccurate or incomplete information can lead to misleading outcomes, which can negatively impact decision-making processes and the effectiveness of educational interventions. Prioritizing ongoing data quality management allows schools and academic institutions to ensure their machine learning predictions are trustworthy and beneficial for student performance.
2. Data privacy concerns
Using machine learning to predict student performance carries the risk of sensitive student data being mishandled or exposed. As cyberattacks become more robust, sophisticated and complex to detect, the potential for breaches increases significantly. This reality necessitates stringent protection measures to safeguard pupils’ information.
Educational institutions must implement advanced security protocols and continuously update their defenses to protect against these evolving threats. This ensures the safety of student data and the integrity of their machine learning systems.
3. Bias and fairness issues
The potential for machine learning models to reflect and amplify existing biases is a significant concern in education. In 2023, 63% of U.S. adults expressed worries about AI bias and discrimination, highlighting the importance of addressing these issues.
Ensuring fairness and equity in predictions presents substantial challenges, as biased data can lead to unjust outcomes that disproportionately affect certain student groups. Educational institutions must be vigilant in auditing and refining their machine learning models to minimize bias and promote a more equitable and inclusive learning environment for all pupils.
Balancing ethics and innovation in education
Emphasizing ethical considerations and human oversight is crucial when integrating machine learning into education. Its role in education evolves as advancements continue, offering new opportunities and challenges. Ensuring institutions use these technologies responsibly requires a balance between automated systems and human judgment.
Educators and policymakers must collaborate to establish guidelines promoting ethical use, guaranteeing that the benefits of machine learning are realized without compromising student welfare.
This is a well-rounded exploration of both the potential and challenges of using machine learning in education. As rightly mentioned, data quality, privacy, and bias are not just technical hurdles—they’re core to how equitable and effective these systems can truly be.
In our experience at Dserve AI, we’ve seen how even slight inconsistencies or gaps in data can skew outcomes and reinforce existing disparities. That’s why we focus on building high-quality, bias-audited, and domain-specific datasets for machine learning applications, including education. The goal isn’t just prediction—it’s trustworthy prediction that leads to meaningful interventions.
It’s also refreshing to see an emphasis on human oversight. Machine learning should support educators, not replace them. Combining strong data with ethical frameworks and professional insight is the only way to make AI truly effective in shaping student success.
Would love to hear how others are handling bias mitigation or student data protection in their ML pipelines.
Machine learning has great potential to predict student outcomes by analyzing key factors like grades, attendance, and engagement. This can help educators personalize learning and provide early interventions. However, challenges such as data privacy, biases in algorithms, and ethical concerns must be carefully addressed. While AI can enhance education, human oversight remains essential to ensure fairness and accuracy in predictions. A balanced approach that combines technology with thoughtful implementation will lead to better academic outcomes.