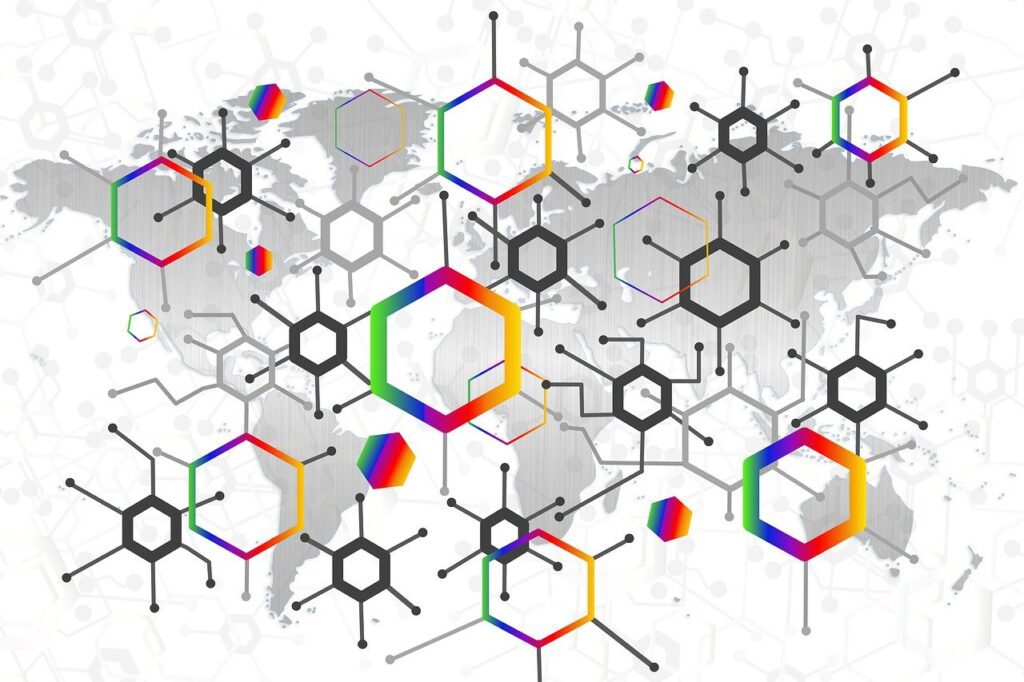
One of the common dilemmas in a typical large enterprise is that multiple groups from different geographies and business units are often spending innovation budget to solve similar problems. The left hand typically doesn’t know what the right hand is doing. But even if an innovation initiative’s leaders are diligent, it might take months to discover what others are doing that’s relevant and align with those other efforts–to the extent alignment is possible when the initiatives are in mid-stream.
What if leadership had end-to-end visibility into the activities of all these different groups and how they’re proceeding to begin with? What if one method was clearly superior to others In terms of its scalability and relevance to both organizational change and data transformation?
Knowledge graphs are clearly superior in making data and content integration easier, as well as to scale integration and contextualization efforts. Many data scientists, engineers, knowledge management professionals, search engine optimization experts and other practitioners know this. Even Gartner in its Impact Radar for 2024 put knowledge graphs in the bullseye with generative AI, the two technologies Gartner decided promised the biggest impact potential this year.
Graph modeling in organizational change scenarios
Besides the clear integration advantages, knowledge graphs can also help with organizational change. They’re highly effective at mapping and dynamically depicting evolving relationships, describing what groups have in common as well as how they differ, depicting process flows and connecting all the interested parties to resources, particularly information resources.
These graphs can become the default means for end-to-end visibility, enhancing leadership’s span of control. And their use in conjunction with simulation modeling and predictive analytics can enable detailed scenario planning.
Guerrilla innovation tech teams often punch above their own weight. Consider how these teams can become more visible and available to top leadership. A given team might be focused on department-level initiatives, where they can make the most immediate impact. But savvy leadership can give such a group follow-on projects, supporting and expanding their reach with more budget, helping to replicate their success across the enterprise.
Leadership can be inspired by knowledge graphs in their whiteboarding tasks. Graph-enabled dashboards and GUIs can encourage collaboration on high-level innovation graph models. These graph-inspired methods are quite comparable to mind mapping, but with additional flexibility and utility beyond simple categorization and hierarchical connection.
How innovation teams will harness the power of a knowledge graph
Innovation teams choosing to use knowledge graphs will start small, addressing pain points that allow quick wins. As they scale out their efforts over time, these teams can help to articulate a future vision that will underscore the scope of meaningful transformation that can take place, including:
- Transparent, dynamic views of the business. Ontologies – the who, what, when, where and why of a subject area and how they’re logically interrelated in graph form–are at the heart of every knowledge graph. The best enterprise ontologies allow a concise, extensible graph model of the business to be built, shared and evolved.
- A circulatory system for business operations. While the main model serves as an upper ontology or highest level of abstraction, domain-specific models articulate how departments or lines of business fulfill their own roles at more specific levels of detail. Other models can be incorporated at lower levels. Spatio-temporal and event models that are also interrelated later on bring the knowledge graph full circle, so it can serve to feed business simulations, AI and analytic systems.
- Living, growing, reusable networks of people, places, things and ideas. Back in the 1960s when punch cards were the norm, the only data was dead data. Today’s ubiquitous compute, networking and storage allows the best engineering teams to design and build once, evolve and reuse everywhere, enlivening the data environment in the process..
- Favored alternatives to legacy, half-dead IT systems. These engineers can be inspired by the examples of the natural world, where networks, interactions and reusability are the norm. Moreover, businesspeople who use the networks in everyday activities, as they become valid alternatives to the application-centric IT networks that have been dominant, will come to depend on living systems.
- A means of broader consensus, security and control. Thousands of databases, each with its own data model, imply pervasive siloing and a lack of transparency. To be valid, governance, risk and compliance efforts need to develop self-describing and self healing information resources based on a unitary, extensible, standards-based graph data model.
- Stakeholders and leaders who want to be involved and data literate. Greater transparency gives stakeholders and leaders a reason to be optimistic about the health of the systems they depend on. Graphs make it possible to bring all sorts of external and internal heterogeneous data sources together, and they can do so virtually, leaving the data in place. Such advantages are part and parcel of a data architecture designed for sustainability and growth.
A system of interacting digital twins and agents for continual innovation
Once an organization commits to semantic graph methods, interoperability at scale and interactive digital twins become feasible goals. The notion of interacting digital twins implies what Wired Co-Founder Kevin Kelly calls a “Mirrorworld”. In this context, envisioning a future for the business becomes addictive.