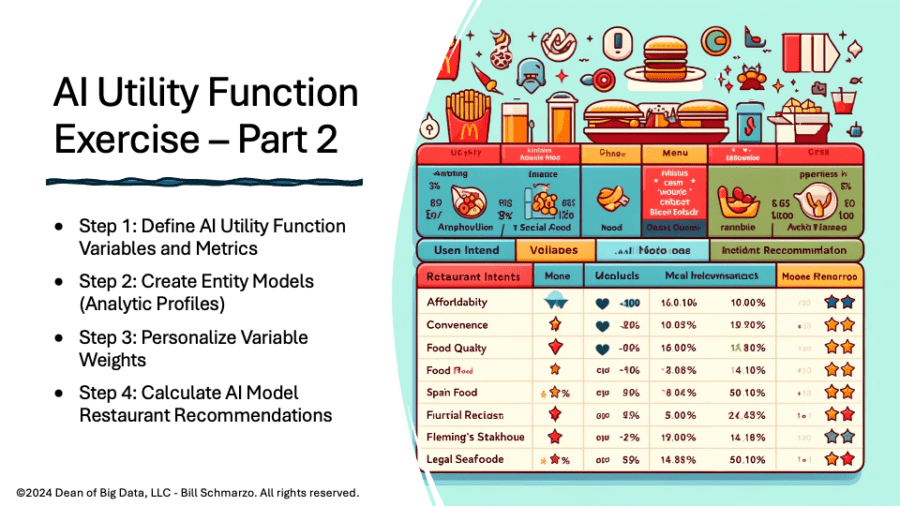
The media, including movies, have made AI conversations confusing and overly emotional. I propose a simple exercise for middle and high school students to provide hands-on training in understanding how an AI model works and the vital role of the AI Utility Function. The exercise will focus on something we do nearly every day: deciding where to dine. We will follow a four-step process to define, create, and execute our “Dining Recommendation” AI Utility Function.
In Part 1 of this two-part blog series, we covered the first two steps:
- In the first step, students were introduced to the foundational concept of defining relevant variables and metrics, such as affordability, food quality, and ambiance. This stage is essential for setting up the criteria the AI will use later to evaluate options. Students learn to think critically about the factors that most influence their decisions, mirroring how AI systems must be programmed to consider various elements in their algorithms.
- In Step 2, we create entity models for each restaurant. Each establishment is scored on a scale of 1 to 100 based on defined variables in this step. This quantification process demonstrates to students how raw data and subjective assessments are standardized for fair comparisons across different options. It clearly illustrates how AI systems process and evaluate diverse inputs.
In Part 2, we will finish the exercise by covering steps 3 and 4. This hands-on exercise aims to clarify AI for students and emphasize the significance of defining AI Utility Functions to guarantee ethical and responsible AI results. By grasping these processes, students can understand how AI models are created to mirror human values and societal norms.
Step 3: Personalize Variable Weights
In step 3, students will explore the significance of variable weighting by assigning weights to each of the ten variables, reflecting their intent and dining preferences. For instance, if students seek a quick, inexpensive meal, they may prioritize affordability, convenience, and value for money. Conversely, the emphasis might shift to ambiance, social media reviews, and menu quality for a date night. Similarly, if food quality is prioritized over cost, a higher weight could be assigned to the food quality variable (Figure 1).
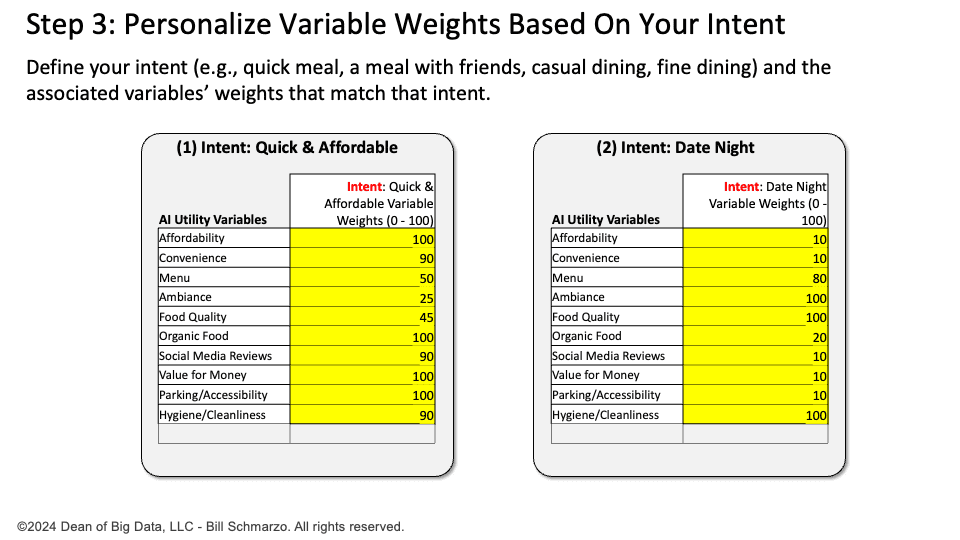
Figure 1: Personalize Variable Weights Based On Your Intent
In this activity, students should be encouraged to experiment with the weights of each of the ten variables to see how that changes the AI model’s recommendations. This will help them understand the concept of weighted decision-making, where all factors are not equally important, and the outcome should reflect their priorities.
Step 4: Calculate AI Model Restaurant Recommendations
In the final step of our AI Utility Function Exercise, we will simulate the decision-making process typical of AI systems by calculating a composite score for each restaurant. Based upon the personalized variable weights associated with my dining intent, we can now calculate each restaurant’s relative score, ranking them based on the student’s individualized criteria.
This calculation involves a straightforward mathematical operation: the scores from Step 2—each restaurant’s performance on the ten variables—are multiplied by the individual weights assigned by the students in Step 3, reflecting their dining preferences. This step demonstrates how AI leverages user preferences in its prioritization process and how students’ variable weights shape AI’s decisions and recommendations. The results of these multiplications are then summed to produce a total score for each restaurant (Figure 2).
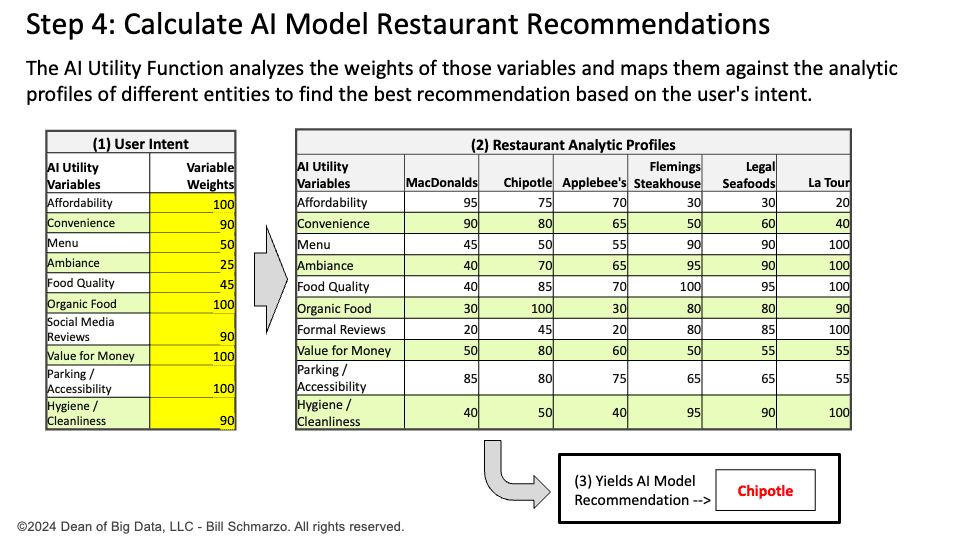
Figure 2: Calculate AI Model Restaurant Recommendations
By performing these calculations within a spreadsheet, students can visually track how each variable’s weight influences the AI model’s recommendation, clearly seeing which restaurant best aligns with their personalized criteria. This approach demystifies the computational aspect of AI utility functions and reinforces the importance of weighting in achieving personalized outcomes.
Note: Moving from handling a few variables and a few restaurants to analyzing tens of thousands of variables across thousands of restaurants dramatically increases computational demands. Machine learning algorithms become essential for efficiently handling these vast datasets and complex variable interactions. Reinforcement Learning (RL) algorithms enable continuous learning and recommendation adjustments to optimize our dining experience in dynamic environments. For example, if unexpected traffic affects accessibility to our highest-prioritized restaurant, the RL-powered AI Utility Function can recalculate and prioritize the next best dining options in real time.
AI Utility Function Exercise Summary – Part 2
This “Becoming an AI Utility Function” exercise helps students understand how AI models make personalized recommendations by using a familiar activity – deciding where to dine. It involves four critical steps to enhance the understanding of how an AI model works to make a recommendation or decision.
- In Step 1, students are introduced to the foundational concept of defining relevant variables and metrics, such as affordability, food quality, and ambiance. This stage is essential for setting up the criteria the AI model will use later to evaluate dining options. Students learn to think critically about the factors that most influence their decisions, mirroring how AI systems must be programmed to consider various elements in their algorithms.
- In Step 2, we create analytic profiles or entity models for each restaurant. Each restaurant is scored on a scale of 1 to 100 based on the variables defined in Step 1. This quantification process demonstrates to students how raw data and subjective assessments are standardized for fair comparisons across different options. It also illustrates how AI systems process and evaluate diverse inputs.
- In step 3, students significantly impact the AI model by personalizing or customizing the variables’ weights based on their specific dining intent and dining experiences. For example, they may be looking for a quick and affordable meal or a more ambient setting for a date night. They can then observe how these weights influence the final recommendations.
- In step 4, the exercise ends with each restaurant’s composite score calculation, revealing which best matches their preferences.
Hopefully, this exercise demystifies the AI’s decision-making process and highlights the importance of brainstorming the variables and weighing them based on their intent and desired outcomes.
In this educational activity, students gained practical experience with the principles of the AI utility function. They learned that the effectiveness of AI’s decision-making processes is greatly influenced by the precise definition and alignment of variables with the user’s intent. This hands-on exploration showcased AI technologies’ capacity to make well-informed, ethical, and personalized decisions in real-life scenarios.