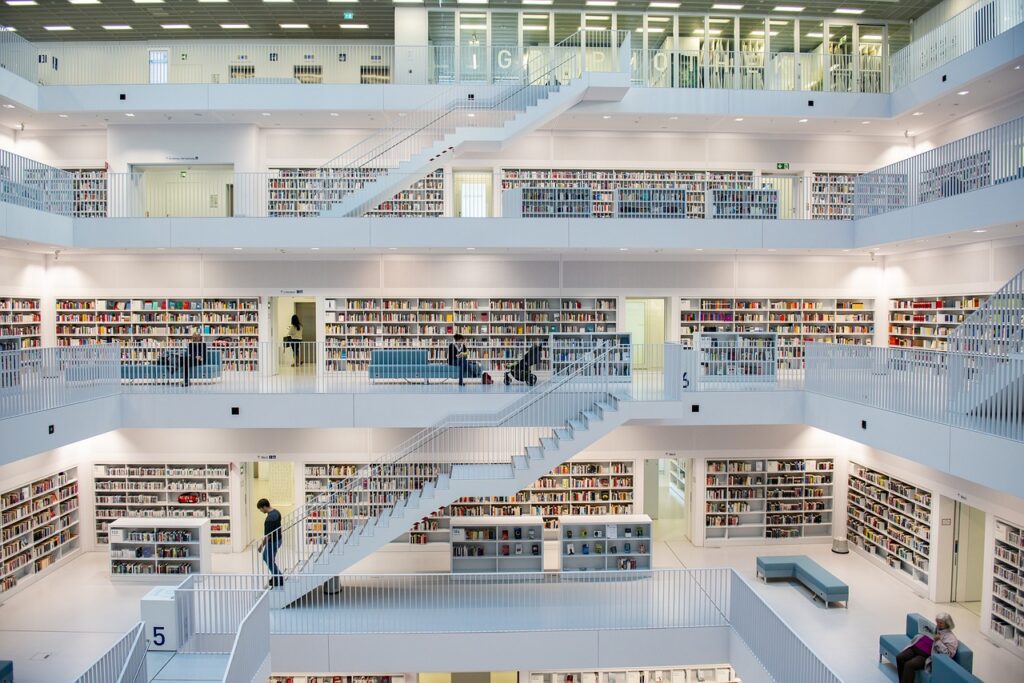
Image by Oli Gotting from Pixabay
While generative AI and the evolution of large language models (LLMs) continue to capture the public’s imagination, it’s worth remembering that the vast majority of business data remains untapped. Even if it were suddenly made available, non-public data wouldn’t be available in a sharable or reusable form.
AI’s value, as I’ve pointed out previously on these DSC pages, is entirely dependent on the quality, quantity, and availability of the right kinds of data inputs. (See https://www.datasciencecentral.com/data-management-implications-of-the-ai-act/, for example.) So regardless of the claims made about how valuable AI is, keep in mind that, if the data isn’t available for it, the AI isn’t going to deliver the value. Period.
The nature of the day-to-day data challenge
If you’re a scientist in the physical, life, or social sciences or a business analyst doing research, the precise detail you need for your research in your niche isn’t often going to be available through a web search or the front end of an LLM. Ironically, scientists know how to collect and manage data better than data scientists do. In any case, teams need dedicated data collectors, stewards, and managers to achieve significant data maturity. (See my previous post on the hiring challenge for more information.)
In that sense, the assertions about artificial general intelligence (AGI) becoming available in 2025 are absurd, because we don’t have the ready data, the data quality, or the level of contextualization that true general AI demands. Most businesses don’t run on scrapings from the public web, and most valid scientific discoveries aren’t made that way either.
The most useful data is difficult to collect. And harnessing the true power of that data requires semantic networks of networks to be built so that machines can be sufficiently context-aware. Otherwise, they’ll be blind to all the various distinctive characteristics that make up each context. The result of the blind spot will be even more ambiguous.
Networks of uniform, logically consistent, actionable meaning and interconnected, disambiguated contexts aren’t easy to build.
Most “knowledge graphs” that do exist and touted via social media don’t deliver knowledge. Instead, they deliver ill-described connections with questionable relevance.
What I’ll talk about next is the most promising effort to date to build a foundation for general, shared intelligence that will benefit businesses most over the long haul. It’s good that the pharma industry in particular has gotten past the denial phase and is investing more in solving the major data problems that have inhibited intelligence innovation.
Without pervasive data maturity and boundary-crossing, shared interoperability, AI can’t be “general”
The AI we do have is still narrow, not general. A big reason for that is that most neural net-based activities don’t take advantage of knowledge representation, the field that spawned the graphs of meaning Google started calling “knowledge graphs” in 2012. To those who haven’t heard, KR lays the groundwork for scaled-out data lifecycle management, addressing the full spectrum of data to knowledge to actionable information.
The statistical machine learning community isn’t versed in KR. Some in the community are hostile to KR if they even are aware of it at all. Some think because it’s been around for decades, that it’s been superseded somehow. But the fact is that neural nets and statistical methods have been around since Minsky and the birth of AI as well.
Both the KR and statistical machine learning tribes have to work together to build general intelligence.
Although you can ask an LLM AI any question and get some sort of answer, the tough questions that business people struggle with every day remain unanswered because of the yawning knowledge gap – the lack of contextualized data that allows boundary-crossing, actionable information necessary for fundamental problem solving and innovation. Ontologies – domain-based graph abstractions of meaning are the only way I know of to scale that sort of contextualization.
Among the industries I follow, the pharmaceutical industry has seemed the most acutely aware of its own data contextualization availability, accessibility, and shareability problem. The various life science industries are increasingly interdependent, and the left hand, more and more, needs to be much more aware of what the right hand is doing. Science in general is trending in this very same direction.
Pharma’s industry-wide, ontology-oriented collaboration model
Thus the reason why AstraZeneca, GSK, Novartis, and Pfizer formed the Pistoia Alliance in 2007. This is not to mention that pharma must work at the molecular level, with all the specificity, tight tolerances, and need to abstract complexity that such a focus implies.
This alliance is known for its focus on findable, accessible, interoperable, and reusable (FAIR) data. Key to the success of this FAIR data initiative is shared ontologies, including for both drug discovery and product data management. The product ontology is called IDMP-O, because it follows in the wake of ISO’s IDMP – Identification of Medicinal Products – standards.
An ontology like this one can be a dynamic relatedness map or uniform, broad-ranging, network-of-networks abstraction across the product space. This map of relatedness is what can allow the scalable coordination of supply networks across organizational boundaries. The logical consistency of good ontologies empowers different manifestations of the network effect, including scaled-out, decentralized reasoning that’s just not possible with today’s centralized LLMs.
With explicit relations that describe what’s being made by whom, how, where, and why across this landscape, different kinds of data efficiencies become possible for the first time for all the primary participants in the pharma ecosystem. Ideally, a shared, real-time visibility can emerge, including ways to troubleshoot production or distribution bottlenecks or reduce compliance costs that haven’t been feasible before. The result will be much less ambiguity.
“Companies generally plan to integrate IDMP data from Regulatory, Manufacturing, Pharmacovigilance, Supply Chain, and Quality functions within the next three years,” according to senior regulatory expert Michael Stam in an article in Pharmaforum. Stam underscored that ownership issues are one of the inhibiting factors slowing adoption.
The ownership issue should not be surprising to anyone who’s tried to get access to someone else’s data inside a large enterprise. It’s one more challenge that will inhibit our ability to create the AGI we really want and need.