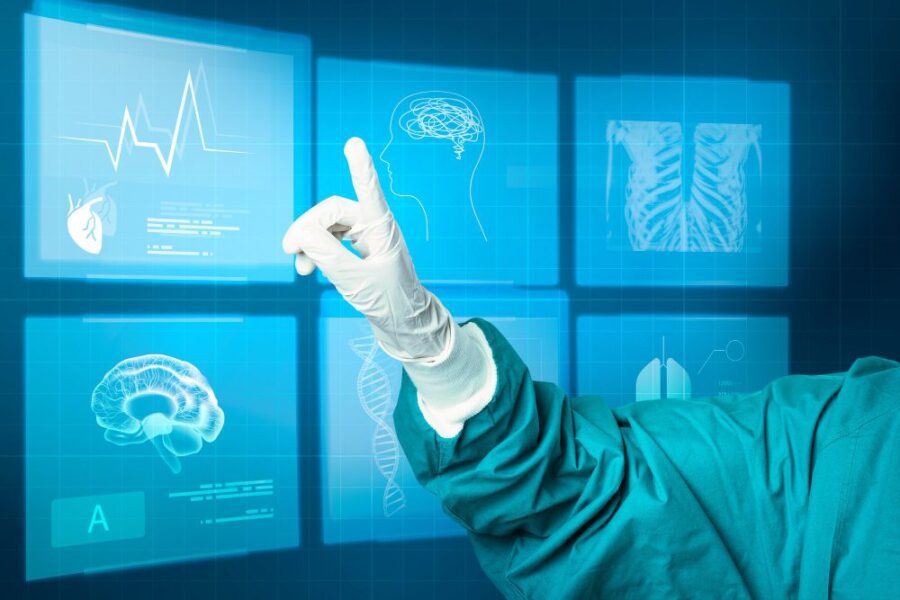
Innovation is increasingly driven by data. As technology advances and alters human behavior, industries collect a growing quantity of information. This data is valuable once we are able to extract actionable, meaningful insights from it – insights that can accelerate better outcomes while remaining equitable and inclusive of the populations we serve, allowing us to work more efficiently.
There is significant interest in predictive analytics, which employs a variety of statistical techniques, including predictive and data modeling, artificial intelligence (AI), machine learning, and deep learning, to analyze data and predict future, unknowable outcomes. It is prevalent in numerous industries, including business management, retail, travel, and sports, as well as science, healthcare, and pharma.
Predictive analytics can help clinicians predict and prevent events, improving patient outcomes. Targeted therapies like precision cancer treatment and cost waste identification improve systems. This blog will examine how predictive analytics and AI affect healthcare.
Predictive analytics uses current and historical data to predict outcomes. Data analysis can identify behavior associations. From these associations, many models can be created. Businesses can improve decision-making by applying model-specific conditions.
How can predictive analytics be leveraged in healthcare?
Healthcare has always looked ahead. Many have wondered how predictive analysis can benefit medicine given recent advances in AI. Big-data analytics, AI, and machine-learning models can improve healthcare in high-risk areas. AI and predictive analytics can help data scientists understand various health factors.
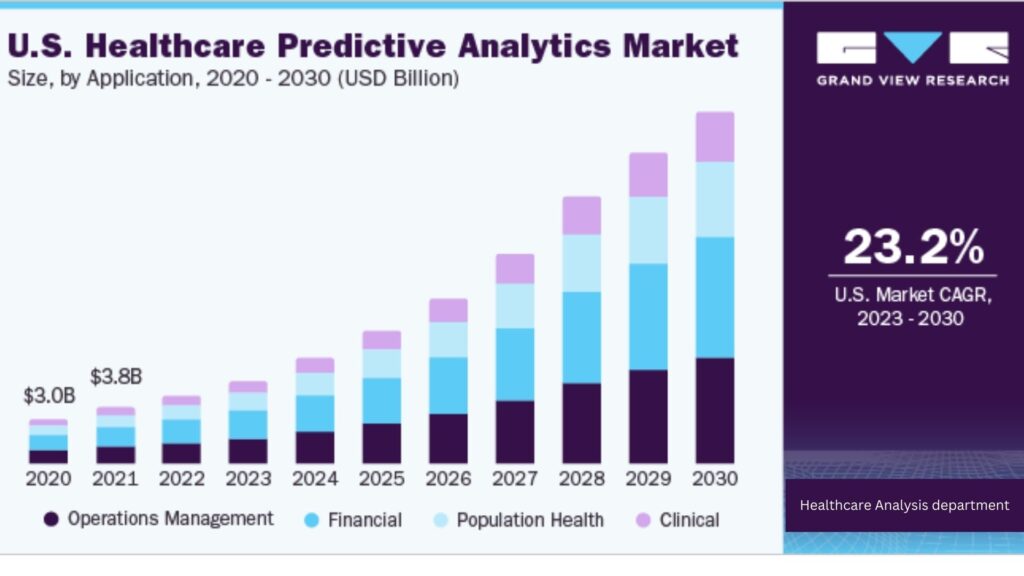
The Role of AI in Enhancing Predictive Analytics:
Predictive analytics in healthcare revenue forecasting are enhanced by AI. EHRs, billing systems, patient demographics, and payer data can be analyzed by AI algorithms. AI-driven predictive models improve with new data.
Consider some ways AI can improve population healthcare management:
Predicting chronic and infectious diseases
Due to data points like the patient’s birth location, work location, lifestyle habits, and local environmental conditions, predictive analytics can help doctors predict illness with high probability. With this information, providers can assess chronic disease risk and prevent it. Diabetes, congestive heart failure, and COPD rates are declining in the sector.
Streamlining patient throughput and workflows
Predicting patient flow with AI-powered predictive analytics rationalizes resource allocation. By extrapolating patient needs, these forecasts can optimize bed allocations, staff reorganization, and healthcare facility employment rates.
Predicting hospital readmissions
Big data analytics can predict extreme epidemics using machine learning and high computational power. Weather-reported cases, population density, economic profile, etc. can predict contagious diseases.
Analyzing patient deterioration
Data scientists use predictive factor analysis to track disease progression and predict medication side effects to give patients the right treatment at the right time.
Tackling Real-World Data Challenges
One needs large amounts of historical data, “real-time” data from a similar distribution (from which historical data was sampled), and infrastructure to process, analyze, and generate predictive cloud models. Since many groups have siloed their data in private clouds, access is limited to themselves and select partners. Healthcare privacy, PHI, and HIPAA regulations also block data access. Patient’s willingness to share data digitally in patient-centric situations where trust is hard to earn. To build inclusive algorithms, we need full population representation and data sets. Healthcare leaders must explain the benefits of data sharing.
4 strategies for an AI-driven Approach to improve Revenue cycle Performance
Healthcare organizations should consider the following four revenue cycle management AI and automation strategies as use cases for healthcare AI development.
1. Automate manual tasks and focus staff on high-value tasks. 92% of hospitals plan to automate claims management and payment reconciliation. However, much can be improved: Automating revenue cycle functions could save providers $9.8 billion. Automating claims status inquiries alone could save $9.22 per transaction, or over $2.6 billion.
Automate the front-end revenue cycle. Real-time patient eligibility checks keep providers informed of patient coverage and deductible progress. Benefit information automatically pulled from the system to create patient charts reduces manual data entry and error risk. Registration and eligibility errors cause 23.9% of denials.
Automating these tasks speeds up patient check-in and lets staff focus on value-added activities like financial counseling.
2. Prevent denials with machine learning. Payers deny 9% of claims annually. This statistic means the average hospital risks losing nearly $5 million in payments each year. Administrative costs average $118 per claim to recover 63% of denials.
Machine learning lets providers anticipate denied claims. That’s how:
- Payer and CPT code denial causes
- Automated claim reviews using this intelligence
- Flagging missing or incorrect information like charges or patient identifiers
- triggering staff follow-up
Staff can correct claims before submission, increasing clean claim rates. It also helps revenue cycle teams manage denials by focusing on high-value denials and those likely to be overturned.
3. Use demographic data to predict patient billing methods. AI can help revenue cycle teams create highly targeted collection strategies based on a patient’s demographics, payment history, communication preferences, and payment methods. It can also suggest the best time and message to send to individual patients. AI can alert patient financial services to early signs of a patient defaulting on a hospital payment plan. AI-enabled patient financial communications and collections may be crucial in the future since half of patients lack confidence in their ability to pay medical bills.
Propensity-to-pay scoring predicts whether patients will pay their out-of-pocket healthcare costs. Despite the availability of propensity-to-pay solutions, one survey found that only 14% of healthcare organizations use advanced modeling tools to segment accounts and predict propensity-to-pay. Only 25% of providers use data or partners. Data-driven intelligence and processes are needed to boost collection rates.
4. Anticipate payer payments. Predictive analytics lets providers examine payer-specific payment behavior by CPT code to estimate how long a claim will take to be paid and when it will arrive. It accurately predicts claim remittance dates. Coding compliance is critical in healthcare in general but especially in long-term care to ensure accurate reimbursement and avoid penalties for fraudulent or erroneous billing.
Integrating Predictive Analytics into Revenue Management:
Healthcare organizations must prioritize these to maximize AI-driven predictive analytics:
- Data Integration: Integrate data from multiple sources to create a complete and accurate dataset for analysis.
- Technology Infrastructure: Buy powerful AI and data analytics platforms for large datasets and complex algorithms.
- Expertise and Training: Help data scientists and analysts use and interpret AI-driven predictive models.
- Data Privacy and Security: Protect patient data while using AI.
AI in healthcare is expected to grow 50.2% from 2018 to 2023, with hospitals and health systems being the biggest adopters. Leaders should carefully evaluate the business case for AI-driven revenue cycle management and explore small-scale innovations with high returns as the healthcare cost curve bends. Starting now will help healthcare organizations keep up with AI advances and improve financial performance.