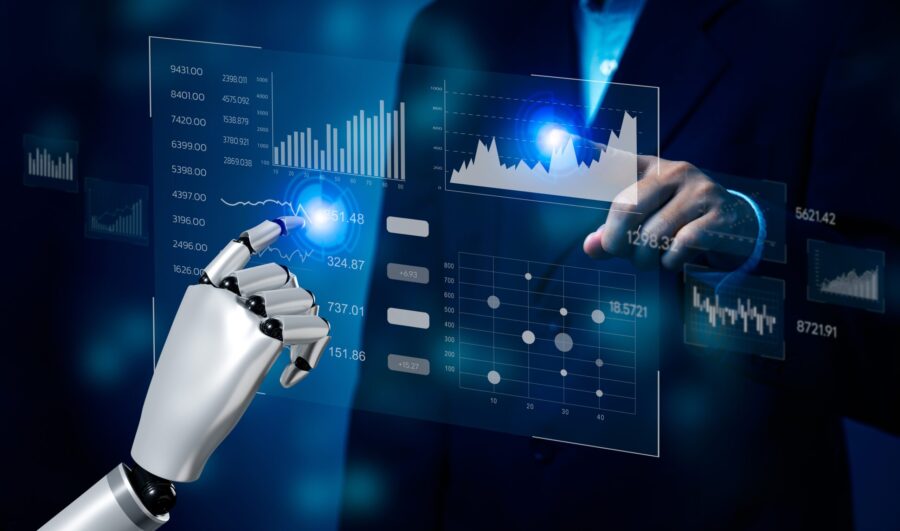
Introduction
The financial world is on the cusp of a remarkable transformation, thanks to the integration of advanced AI models like GPT-4. In this article, we delve into the evolving landscape of Machine Learning (ML) in finance and explore the potential impact of these cutting-edge AI systems.
The need for speed: Reacting to regime shifts
The ability of AI systems like GPT-4 to respond swiftly to regime shifts in the financial landscape is a crucial aspect of their effectiveness. We examine the factors that contribute to the speed at which these AI models can adapt to changing conditions in the market.
- Pre-trained Knowledge: Leveraging existing knowledge for rapid analysis.
- Fine-Tuning Skills: Adapting to specific financial contexts.
- Real-time Data Sources: Staying up-to-date with market changes.
- Parallel Processing: Accelerating data analysis.
- Incremental Learning: Continuous improvement over time.
However, we also highlight the limitations, emphasizing that AI is not a crystal ball and cannot predict all shifts with absolute certainty.
The missing ‘why’: Explaining AI decisions
The importance of understanding the “why” behind AI decisions cannot be overstated, especially in critical domains like finance. We explore the challenges posed by the “black-box” nature of deep learning models and the significance of Explainable AI (XAI) in bridging the gap.
- AI’s Limitations: The struggle to explain decisions.
- The Rise of XAI: Efforts to provide transparent explanations.
- Building Trust: The role of explanations in fostering trust.
- Collaborative Decision-Making: Enhancing cooperation between AI and humans.
While XAI holds promise in enhancing transparency, we acknowledge the ongoing challenges in achieving complete interpretability in complex AI models.
Data’s double-edged sword: The perils of unclean data
The article concludes by underscoring the dangers of using unclean data in AI and machine learning systems, particularly in finance. We shed light on how dirty data can lead to unexpected and undesirable results and discuss the importance of data preprocessing and quality assurance.
- The Data Quandary: The allure and pitfalls of data abundance.
- The Garbage In, Garbage Out Principle: Why data quality matters.
- Data Preprocessing: Cleaning and refining data for reliability.
- Maintaining Data Governance: Upholding standards in critical domains.
Conclusion
The fusion of AI models like GPT-4 with finance holds immense potential, but it requires careful navigation through the seas of data quality and interpretability. Understanding the capabilities and limitations of AI systems is key to harnessing their power while mitigating risks in the financial world.