Takeaways from my July 2024 interview with cloud architect and author David Linthicum
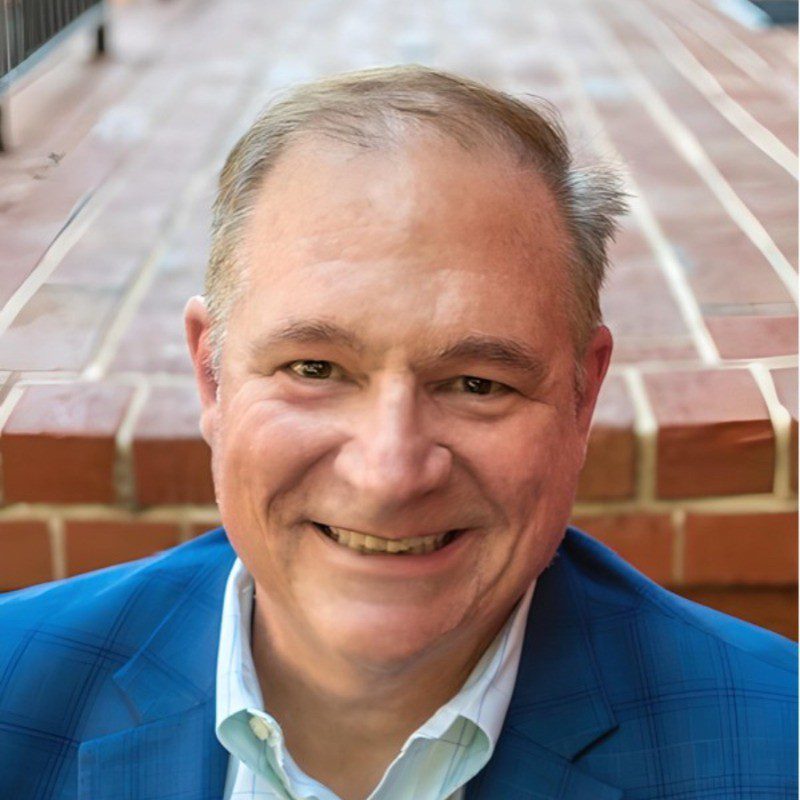
David Linthicum
Wall Street now agrees that generative AI is past the Peak of Inflated Expectations and heading down into the Trough of Disillusionment,
Analysts are starting to paint a clearer picture of how far expectations have fallen, “expecting Big Tech companies to spend $60 billion a year on developing AI models by 2026, but reap only $20 billion a year in revenue from AI by that point,” according to Gerrit De Vynck in the Washington Post, who cited Barclays in a July 24, 2024 article.
So what about large enterprises in other industries? Fortunately, most aren’t that far along with their own generative AI efforts. Hopefully cooler heads will eventually prevail.
Once they do, ex-CTO, CEO and former Chief Cloud Strategy Officer at Deloitte David LInthicum would like a word with them on crafting an AI strategy for the long term. Most of all, he doesn’t want these organizations to repeat the same kinds of mistakes over and over again when it comes to new technology adoption.
Linthicum has lived through numerous hype cycles before. Back in the 2010s, many of these same organizations–-established multinationals in mature industries–were all just as eager to move to Amazon Web Services. The result was a mixed bag. While some companies made out okay, many others did not.
In 2024, one big lesson Linthicum imparts to his clients (not to mention those who read his blogs and books) is to find some impulse control. Don’t give in to the solutions gaining the most media attention, in other words. Have the patience to do the hard work to seek out, wait for, discover and tailor a solution to the needs of the business.
A short list of the main takeaways
The complete conversation below is definitely worth a listen. Listed next are the top five takeaways from our conversation that apply to generative AI.
5. Take the time and locate the expertise to make good architectural choices.
Linthicum: “We went through this whole cloud computing mix in 2011-2013. People knew they wanted cloud. There were some reference architectures out there. At the end of the day, they needed much more tactically focused, bespoke architectures.
“The same thing is happening with generative AI, to a larger degree…. These [generative AI clusters designed for large language model development] cost five to ten times as much to build in the power. (‘See How AI growth has triggered data center redesign’ https://www.datasciencecentral.com/how-ai-growth-has-triggered-data-center-redesign/ for more information on the power management and cooling requirements for LLM development.)”
4. Small language models make the most sense for practical, detailed decision making.
“With supply chain integration, you’re dealing with a very finite dataset: Your logistics, sales and inventory information, for example. You’ll use that to train a small language model to make very tactical decisions in terms of how to best deploy your trucking environment, the best paths for the trucks to take, where to find the cheapest fuel, how to address weather anomalies…. These are real live business decisions that people deal with on a daily basis.”
3. Your generative AI system is stupid because your data is stupid.
“You’re only going to have access to three datasets out there when you’ll need access to 50. That’s because they’re siloed, not integrated. Companies have bad data hygiene. They don’t know the meaning of the data. We have to have a semantic understanding of that data. So we get into this fantasy that this AI system is going to automagically fix the backend system…. Either you fix your data, or your AI system is going to be worthless.”
2. Try to gain strategic advantage this time with a transformed data architecture, instead of kicking the can down the road again.
“A lot of technical debt has been created, and data is scattered across public cloud providers and on premise. It’s not well integrated. These companies have no data abstraction layers and no common semantic understanding. The older the companies are, the more likely it is that they have these problems. To get the value out of a generative AI system, it will need access to all of the data holistically and the ability to have a common understanding of what that is.”
1. Brace yourself for more security challenges, given more tech debt and complexity.
“If you’re going to open up these chat engines and APIs to the public, then you’ll be exposed to malicious shenanigans, such as introducing logic where every Thursday the company sends a check for $25,000 to the malware supplier….. The vulnerabilities are coming from the complexity–50 different databases, platforms, government cloud systems…. We have a lot of cybersecurity experts out there who have no idea how to protect the AI systems. Fortunately, not a lot of these AI systems are built yet…. There’s not anything to worry about because nothing’s there to protect.”
Linthicum gives readers suffering from AI and cloud hype a regular antidote, and he’s even more outspoken during an interview. I hope you find this interview as compelling as I have.
Podcast interview with David Linthicum, author of An Insider’s Guide to Cloud Computing