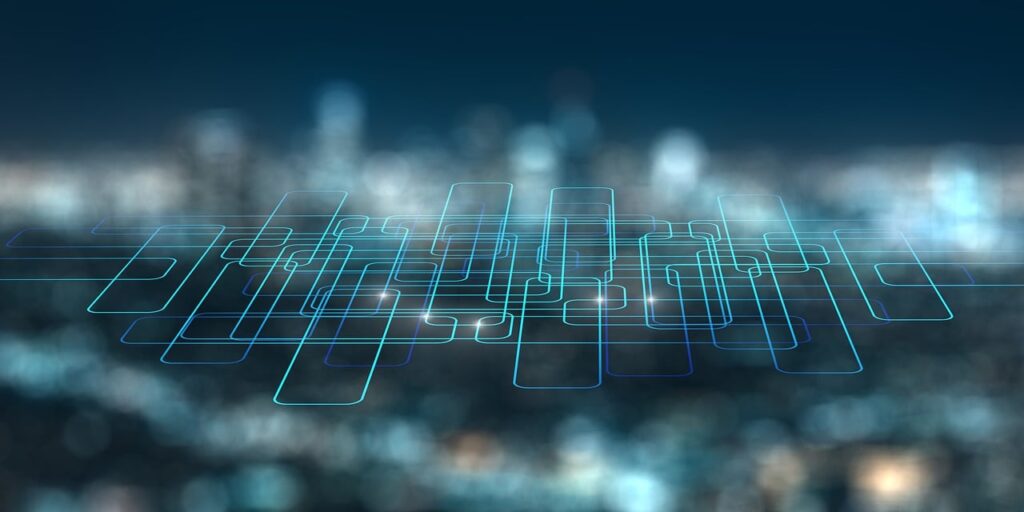
Image by Gerd Altmann from Pixabay
A few enterprise takeaways from the AI hardware and edge AI summit 2024
Enterprises haven’t seemed as enthusiastic about generative AI and large language models (LLMs) lately as they have been in previous years. The Kisaco Research event I attended in September provided some reasons why.
Current gen AI processing far too centralized to be efficient or cost effective
If there’s a single takeaway that I could point to, it’s how overloaded data centers are and how limited edge infrastructure has been when it comes to effectively reducing that data center load for gen AI applications. Ankur Gupta, Senior Vice President and General Manager at Siemens Electronic Data Automation noted during his talk that “the opportunity for low power needs to be met at the edge.”
Gen AI-oriented data centers must handle an inordinate amount of heat per GPU. Gupta asserted that half a liter of water evaporates with every ChatGPT prompt.
The newest, largest GPUs run even hotter. Tobias Mann in The Register in March 2024 wrote that “Nvidia says the [Blackwell] chip can output 1,200W of thermal energy when pumping out the full 20 petaFLOPS of FP4.” Even so, Charlotte Trueman writing in August 2024 in Data Center Dynamics and citing Nvidia CFO Colette Kress, wrote that “Nvidia was expecting to ship ‘several billion dollars in Blackwell revenue during Q4 2024.’”
Edge infrastructure innovation is key, with significant spending planned. IDC recently estimated that global spending on edge computing will reach $228 billion in 2024, a 14% increase from 2023. IDC forecasts spending to rise to $378 billion by 2028, a 13 percent CAGR from 2024 levels.
The research firm “expects all 19 enterprise industries profiled in the spending guide to see five-year double-digit compound annual growth rates (CAGRs) over the forecast period,” with the banking sector spending the most, according to CDO Trends.
To justify this level of investment, Lip-Bu Tan, chairman of Walden International, forecast edge AI revenue potential of $140 billion annually by 2033.
Aren’t smaller language models (SLMs) better for most purposes?
Donald Thompson, Distinguished Engineer @ Microsoft / LinkedIn, compared and contrasted LLMs with SLMs, saying he favors SLMs. It’s not often, he says, that users really need state-of-the-art LLMs. SLMs can allow faster inference, more efficiency and customizability.
Moreover, a solid micro-prompting approach can harness the power of functional, logically divided tasks, in the process improving accuracy. Thompson shared an example user dialogue agentic workflow that enables a form of knowledge graph creation. A dialectic that’s part of this user dialogue flow includes thesis, antithesis and synthesis, eliciting a broader, more informed viewpoint.
Pragmatic enterprise AI starts with better data and organizational change management
Manish Patel, Founding Partner at Nava Ventures, moderated a panel session on
“Emerging Architectures for Applications Using LLMs – The Transition to LLM Agents.” Panelists included Daniel Wu of the Stanford University AI Professional Program, Arun Nandi, Senior Director and Head of Data & Analytics, at Unilever, and Neeraj Kumar, Chief Data Scientist at Pacific Northwest National Laboratory.
The prospect of agentic AI is placing much more focus on the need for governance, risk assessment and improved data quality.
In order for those improvements to be realized, AI adoption must wait for organizational change. Wu pointed out that inside enterprises, “Change management is the single point of failure.” Even successful change efforts take years.
Moreover, expectations about AI are often unrealistic, with executives who don’t have the patience to wait for return on investment.
Kumar underscored the cross-functional nature of AI deployments and ownership considerations that arise as a result.
Nandi figured that “70 percent of the effort (in enterprise AI initiatives)” are change management related, and that such initiatives imply a need for much more extensive collaboration, given AI’s cross-functional nature, with the right people in the right roles in the loop.
Effective edge AI requires a different, linear modeling approach
Stephen Brightfield, CMO of neuromorphic IP provider Brainchip, presented on “Combining Efficient Models with Efficient Architectures.” Brainchip specializes in on-chip, in-cabin processing technology for smart car applications.
Brightfield asserted that “most edge hardware is stalled because it’s designed with a data center mentality.” Some of the observations he made underscored the learnings of an edge-constrained environment:
- Assume fixed power limits.
- Lots of parameters implies a lot of data to move.
- Most data isn’t relevant.
- Sparse data implies more efficiency.
- Don’t recompute what hasn’t changed.
Rather than stick with a transformer-based neural net of the kind used in LLMs, Brainchip advocates a state space, state evolving, event-based model that promises more efficiency and lower latency.
A final thought
Much media coverage is focused on LLM behemoths and the data center-related activities of hyperscalers. But what I found much more compelling were the innovations of smaller providers who were trying to boost the performance and utility of edge AI. After all, inferencing is consuming 80 percent of the energy AI demands, and the potential clearly exists to improve efficiencies through better and more pervasive edge processing.