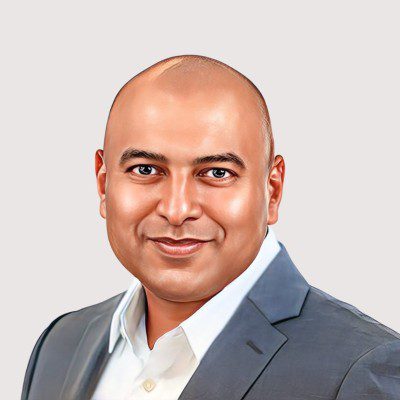
An interview with Chetan Conikee, founder and CTO at Qwiet.ai
Serial entrepreneur Chetan Conikee honed his skills as a technical lead, engineering manager and architect in the financial services industry. Even though his role wasn’t dedicated to cybersecurity, in such a highly regulated environment, security was part and parcel of what he was focused on.
“When you’re dealing with both investments and retail from a banking perspective,” Conikee explained, “you’re actually touching many underlying systems that are verifying your identity, checking your balances, helping you consolidate your assets and move your money within institutions in the United States and across the border into other countries as well.
“Now, all of this is regulated strictly in order to ensure that there is no malicious intent and there is no fraud that happens when any such transactions are playing out. So I spent over 15 years essentially building protocols, building backend systems for high frequency trading, building backend systems for identity verification and fraud detection, both on streaming data and transactional data.”
“At the time, teams provisioned many security measures manually, which left a lot of room for human error,” Conikee said. “And progressively over time, when I was managing many teams, I began to automate those functions so that I’m not the human gathering that information or I’m not asking another human to gather that information.”
Qwiet.ai, Conikee’s latest venture, adds a predictive modeling capability to the security automation challenge, harnessing a layered property graph to assist with path predictions, identifying where attacks are most likely to occur.
As Conikee describes it, “The code property graph is like a map. First, it plots your source code as various routes. The next thing it does is place context information on those map packs or those routes, like your API endpoints. Here are points of presence in your code where you’re interacting with cloud resources, file systems, caches, and so on and so forth….
“We’re figuring out which path is more amiable to traverse, and then begin the traversal on that path by enumerating that path with various types of attacks.
“At some point, something’s going to succeed, and that’s the next step. So what we do is after plotting the map, we run a series of such scripts to figure out weaknesses on those facts. And then we classify that path as vulnerable, which would require attention and remediation.”
Using a blend of techniques, Quiet.ai has come up with a number of clever ways like these to make better security and related risk and compliance manageable. Hope you enjoy the podcast.