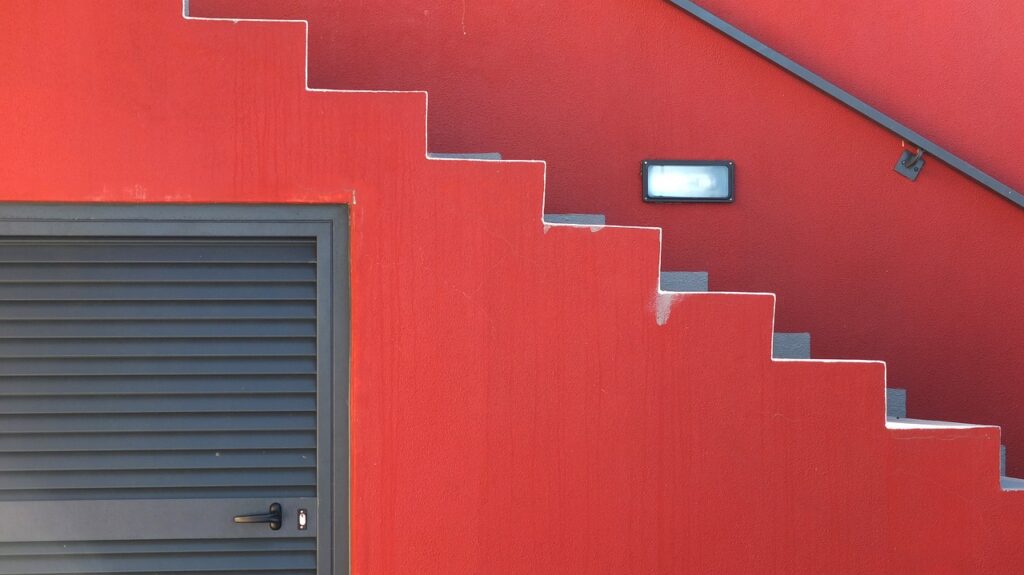
Andrew Holloway on Pixabay
The vast majority of companies have been addicted for many years to software-as-a-service applications. Month after month, organizations worldwide pay tens of billions of dollars in efforts to obtain “easy,” on-demand solutions to the problems of the day.
But the solutions are never easy. Most apps never really deliver on their promises. Users typically get the blame.
It’s never really the application’s fault. Instead, the users didn’t really do what they should have to make the application work as designed.
Years later, more providers make compelling pitches. The same companies try to work the problem from the vantage point of new providers or at least a new generation of apps, committing to yet another subscription.
The result from a workflow point of view is a doom loop of fragmentation, forcing users to dive task after task into as many as a dozen or more different applications to get their work done.
Typical SaaS and other enterprise applications do three things:
- A means for data input
- A means for information output
- Connections to input and output workflows
For that reason, each application becomes one of many black boxes, each of which duplicates or overlaps the others’ functions. Moreover, each new SaaS adds a new and different data model to the mix, implying a continually rising integration workload for those who are trying to use application data downstream in a unified fashion.
Ironically, by indiscriminately subscribing to more and more SaaSes, enterprises are adding another layer of complexity on top of an already challenging situation.
Knowledge graphs, by contrast, allow the visibility and shareability of common functions and data. Knowledge graph architecture encourages the elimination of functional and data duplication.
SaaS spending and the related data challenge
In 2024, global SaaS spending will reach $247 billion, rising to $295 billion in 2025, according to Gartner, a growth rate of over 19 percent.
By unthinkingly throwing good money after bad in pursuit of the killer cloud app, leadership continues to demonstrate a disregard for the root problem — the need for the workforce to get hands on with data as an organically evolving asset, an asset more and more in demand considering the growth of AI.
With a knowledge graph to anchor the development of an enriched, reusable, interoperable data asset, a company can work toward a dynamic data fabric for all applications. The ultimate goal with knowledge graph is findable, accessible, interoperable and reusable (FAIR) data for the tasks at hand.
SaaSes can become a major obstacle to a knowledge graph development effort. SaaSes by definition vest responsibility with the service provider to do the data management. By handing that responsibility over, organizations create a situation in which the organization doesn’t even control its own data, and has to ask permission from the provider to get that data.
The alternative is to shift investment to knowledge graph development. Such investment makes it possible for the organization’s own workforce to commit, domain by domain, to a scalable FAIR data development program. Such a program dovetails nicely with enterprise AI data needs, in fact.
The root cause of AI failures: A lack of purpose-built FAIR data to feed the AIs
Vernon Keenan, an analyst for SalesforceDevops.net, reported in August 2024 on a RAND Corporation study which estimated that 80 percent of corporate AI projects fail. “The most common cause of AI project failure?” He asked. “It’s not the technology – it’s the people at the top. Business leaders often misunderstand or miscommunicate what problems need to be solved using AI. As one interviewee put it, ‘They think they have great data because they get weekly sales reports, but they don’t realize the data they have currently may not meet its new purpose.’”
The backstory evident behind each AI failure is the inadequacy of the overall data lifecycle management approach of the organizations in question. AIs need more than the sparse tabular, numerical data organizations typically think of first when it comes to the data they inventory; instead they need the richness of a holistically integrated, contextualized multimedia data environment, with information from a wide variety of sources, systematically collected and described in a graph that scales.
12 steps to a contextualized data fabric
12-step programs help by identifying and addressing root psychological problems that lead to abuse and addition.
Using a 12-step program, many corporations can own up to the state of their data circumstance. They can identify and embrace the best ways to rectify the situation. Knowledge graphs provide a ready, established means of transforming existing sources into discoverable, reusable and even interoperable assets. A reduction in overspending on SaaS apps can fund such a knowledge graph effort.
Here are 12 steps organizations can use to come to terms with their data problems and develop a means of overcoming those problems.
- Identify and admit the extent of the system’s most deeply rooted data problems.
- Redefine business requirements in light of new data demands such as AI.
- Engage business unit professionals with domain knowledge in the areas relevant to requirements as a network of data stewards.
- Design and adopt a data lifecycle management program that harnesses knowledge graph power.
- Inventory and catalog all existing data relevant to current needs.
- Collect additional data suited to current requirements with the help of business unit data stewards.
- Clean the data and contextualize it with a unitary, semantic graph data model that can become the parent for each domain’s data model children.
- Harmonize the data and knowledge graph metadata for sharing and interoperability purposes.
- Enable feedback loops for usability, data management and refinement purposes with the help of a search function.
- Expand the utility of the knowledge graph and continue desiloing efforts with the help of natural language processing, advanced analytics, reasoning and other capabilities.
- Tackle new use cases and evolve the graph case by case.
- Plan for new capabilities such as event-based and stream-oriented graphs.
Key to success with such a 12-step program is a continual focus on desiloing. Leaders as well as technologists and business unit data stewards need to adhere to desiloing principles. Otherwise, the new means of data fabric construction and management won’t scale.