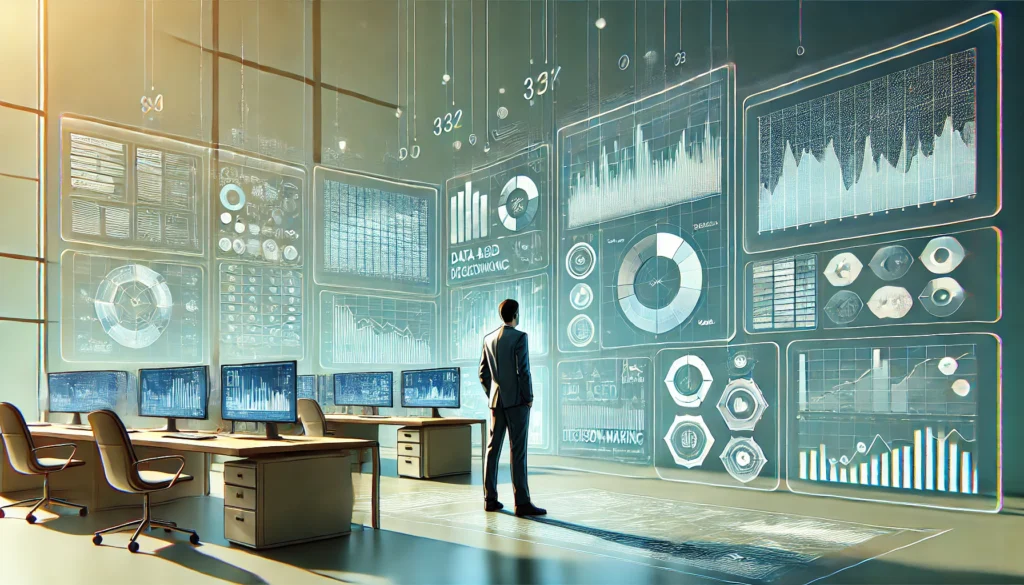
Whether launching a new product or changing an existing one, decision-makers are relying on data more heavily than ever. Today, we can track customer behavior, competitor prices, demand shifts, and even the performance of our products through countless metrics from complex data systems. But when does data-based decision-making go wrong? And what can we do about it?
In this article, I’ll discuss some common gaps I’ve seen in data-driven decisions at large and medium-sized companies (like Google, EY, and others) and share a few ideas we’ve used to close those gaps.
Where things go south
Let’s break down the process, from data gathering to making decisions, as errors can happen at every stage, including data import, data processing, interpretation, and the final application of the results. (The list below is by no means exhaustive and serves rather an illustrative purpose and as a warning signal against overconfidence when dealing with results.)
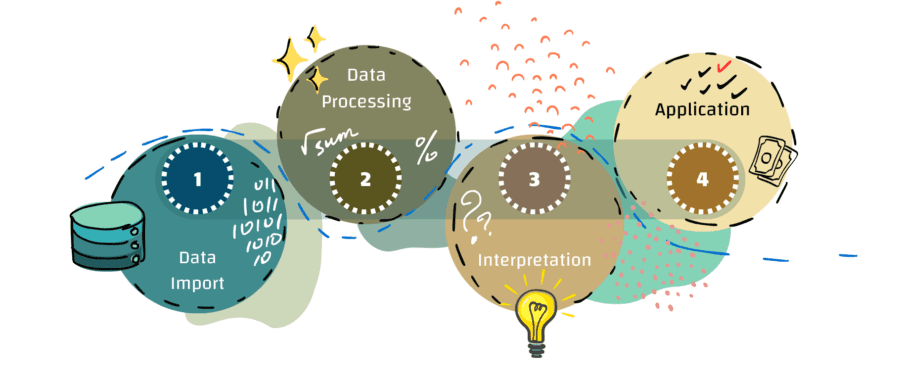
- Bad data in, bad results out
One of the biggest (and most frequent) issues? Bad data. Anything that doesn’t reflect reality can count as “bad” data: from typos (like a misspelled customer name in the CRM) to missing information that was mistakenly filtered out, or using outdated or low-quality vendor data.
Bad data leads to poor insights, and poor insights lead to bad decisions. The results range from inconveniences (someone finds a mistake, and you need to redo the whole analysis) to losing credibility with a customer, or even losing that customer altogether. - Good data, wrong context
Past data can only teach us so much. If you know that consumer behavior or other big factors are shifting, using even high-quality historical data might be pointless. As Ford famously said, “If I had asked people what they wanted, they would have said faster horses”. In other words, just because data worked for past decisions doesn’t mean it should guide future strategy (otherwise, maybe we’d have no cars). - Edge cases (and other processing errors)
Even with good data, processing issues can mess things up. Think about specific cases like leap years (with 29 days in February), or handling edge scenarios for different customers. Miss one of these, and you might end up with inaccurate or incomplete insights. Ensuring a system is in place to catch these unique cases can save time and prevent errors. - Statistical issues
If the statistical methods are used (like regression, machine learning, etc.), a wide range of issues can affect the statistical significance of the obtained results (take multicollinearity, for example). Those issues might be caused by an inadequate design of the statistical model as well as by the limitations of the data at hand. - Correlation vs causality
Events happening together do not always mean that one of them caused the other. They might have a reverse relation (the latter caused the former), or there might be a third event that causes both original events. If correlation is misinterpreted as causation, it can shift the decision in the wrong direction, leading to costly missteps. - Leaning too much into data science
Sometimes, the people analyzing data aren’t closely connected to the business side, which might lead to two kinds of issues.- During data processing, a small mistake might go unnoticed from a purely data-driven perspective, even though it would be obvious to someone with a business context. For example, if results show an odd consumption pattern, a data analyst might miss that easily, whereas a business expert would spot it immediately.
- During interpretation, a tech-focused approach can also lead to creating irrelevant or non-actionable insights (like the number of churned customers without a detailed list of them to work with). Here, a business colleague can help determine the most useful KPIs to show to decision-makers, saving time and effort on both ends.
- It’s just slow
Finding the right data, interpreting it and challenging the results can take a really long time. This is especially true if your company has a complex data landscape and/or a shortage of analysts available to process it. But not all decisions need perfect data. Some decisions, as Jeff Bezos would say, are “two-way doors” – they’re easily reversible if proven wrong. In these cases, it’s often better to just try something quickly and iterate later rather than wait for perfect analysis.
Keeping things on track
Although working with data often brings one of these issues up, the good news is, many of these challenges are manageable (at least to some extent). Here’s what we’ve found works well:
- Decide whether your decision is a “two-way door”. Sometimes, a couple of quick calculations are all you need before moving forward.
- Ask yourself whether historical data aligns with current conditions or if something needs to be adjusted before processing.
- Introduce checks at each step. Check not only for obvious things (like that revenue should be positive, or the financial balance should balance), but also review more specific business-critical KPIs, like total revenue or number of customers. Additionally, skim through the data that got filtered out to ensure that it’s not needed.
- Try double-checking with another analyst: often, a fresh pair of eyes can help spot mistakes and inconsistencies, or improve the design of your statistical models.
- Partner data analysts with business experts to challenge insights and keep interpretations practical.
- Remember that data insights might be wrong (even with checks, yes). This will help you avoid overconfidence when presenting insights to decision-makers.
- Be open to the feedback of your customers, colleagues, or other stakeholders, because your data might be wrongER than theirs 🙂
What about AI?
With greater adoption of AI, the complexity – and sometimes the impossibility – of identifying and verifying exact data sources and processing algorithms adds even more to the pressure on decision-makers to challenge AI-generated results. As of the moment of writing this article (beginning of 2025), OpenAI’s ChatGPT has started incorporating sources for the results generated so that users could verify them or research the topic deeper.
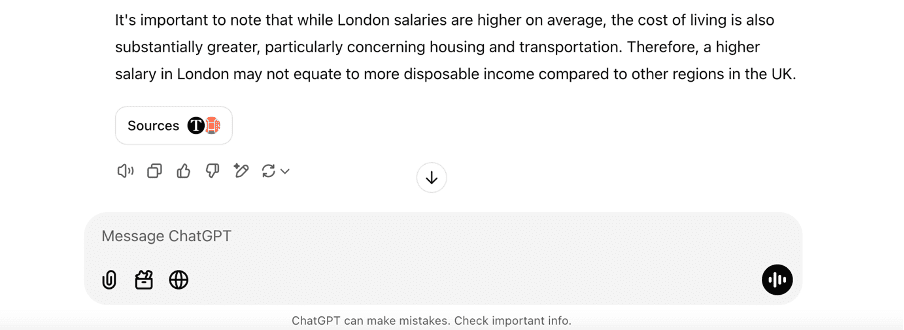
ChatGPT’s interface now includes sources for the results provided. Source: chatgpt.com
At the same time, Google’s Gemini went another way to increase users’ confidence and developed a possibility to back-solve the generated results to check if Google’s search could find similar facts on the web. Later this year, they’ve additionally added a section on sources of the data used.
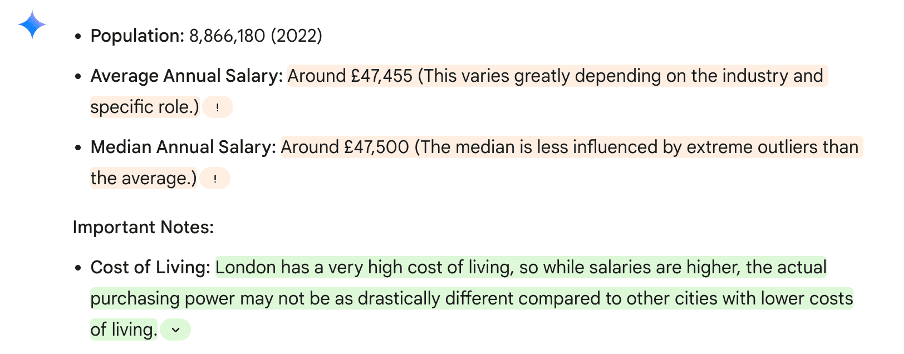
Verification of the results by Gemini: green marks information where similar data can be found in Google search, while amber highlights cases where contradicting information is found. Source: gemini.google.com
Even though those improvements can help, a balance between relying on AI and re-checking the results is still to be found. Both ChatGPT and Gemini still explicitly state that tools can make mistakes and need verifying.

Disclaimer as a part of Gemini’s interface, Source: gemini.google.com
To sum it up, using data for business decisions can be both advantageous and tricky, as so much can happen on the way from inputs to insights to application. While there are steps you can take to prevent ill-informed decisions, the most important is to be mindful that no model (and no data) is perfect, and to be ready to adjust your strategy if and when the changes arise.