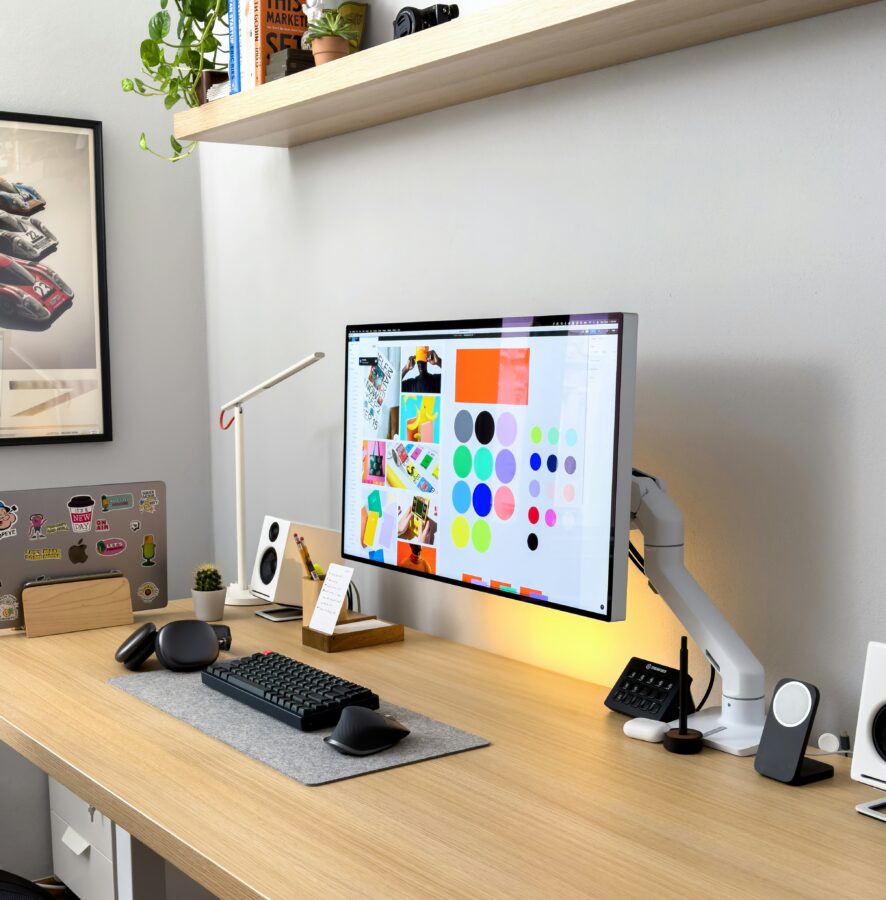
The rapid advancements in artificial intelligence (AI) have significantly altered the landscape of user interface (UI) design, shifting from static, one-size-fits-all interfaces to highly adaptive, personalized experiences. AI-driven personalization leverages machine learning algorithms, behavioral analytics, and real-time data processing to tailor digital interactions for individual users. With 92% of companies now using AI-driven personalization to drive growth, users are each seeing a different version of the website tailored to their experiences and preferences. This article explores the underlying mechanisms that enable AI-driven UI personalization, its practical implementations, and the challenges that come with it.
Understanding AI-driven personalization
AI-driven personalization relies on deep learning models, natural language processing (NLP), and predictive analytics to modify UI elements dynamically. These models analyze user behavior, historical interactions, and contextual data to create individualized experiences. The fundamental steps in AI-powered UI personalization include:
- Data collection and preprocessing – AI systems gather data from multiple sources, such as browsing history, demographic details, purchase behavior, and device interactions. This data is then cleaned, structured, and anonymized to ensure privacy compliance.
- Pattern recognition and clustering – Machine learning algorithms identify patterns in user behavior, segmenting users into distinct profiles based on their preferences and interaction history.
- Real-time content adaptation – AI-driven interfaces leverage reinforcement learning and real-time analytics to modify layout structures, recommend relevant content, and adjust UI elements dynamically.
- Feedback loops and continuous learning – Personalization models continuously refine their predictions by incorporating user feedback, click-through rates, and session duration metrics.
Applications of AI-driven personalization in UI design
Dynamic interface adaptation
Traditional responsive design adjusts layouts based on device specifications, but AI-driven personalization enhances this concept by predicting user preferences. For instance, an AI-powered dashboard can reorganize itself by prioritizing frequently accessed features, reducing cognitive load and improving efficiency. Notably, 76% of consumers admit they get frustrated when organizations do not deliver personalized interactions, making adaptive interfaces crucial for user engagement.
Conversational user interfaces and virtual assistants
Conversational AI, powered by NLP and contextual awareness, enables intelligent virtual assistants and chatbots to refine user interactions. Unlike rule-based chatbots, AI-driven assistants adapt responses based on previous interactions and user sentiment analysis. Companies integrate AI into customer service platforms, allowing automated assistants to resolve queries efficiently while escalating complex cases to human agents. In fact, more than 987 million people use AI chatbots daily, saving time and improving service efficiency.
AI-assisted wireframing and prototyping
Generative design models streamline UI prototyping by producing multiple wireframe variations based on predefined business goals and industry standards. AI-driven design tools such as Uizard and Adobe Sensei analyze industry best practices and user engagement metrics to suggest optimal layouts, colors, and content placements. These tools accelerate the design process while maintaining adherence to usability heuristics.
Personalized content delivery
AI algorithms predict user intent by analyzing clickstream data and engagement history. This predictive capability enables platforms like Netflix and Amazon to deliver customized recommendations, dynamically adjusting homepage content based on real-time interactions. In web applications, personalized dashboards prioritize information that aligns with a user’s historical behavior, minimizing irrelevant distractions.
Accessibility optimization
AI-powered personalization extends beyond convenience to inclusivity. Computer vision algorithms detect accessibility needs, such as font size adjustments for visually impaired users or speech-to-text conversions for hearing-impaired individuals. AI-driven accessibility tools dynamically modify UI elements based on user preferences, ensuring compliance with Web Content Accessibility Guidelines (WCAG). A significant 73% of disabled customers experience accessibility barriers on more than a quarter of websites they visit, highlighting the necessity of AI-driven accessibility solutions.
Automated landing page generation
Creating new landing pages for different search queries, marketing campaigns, or locations can be a time-consuming process. AI can help streamlines this by automating the generation of personalized landing pages, ensuring relevant content is dynamically tailored to user intent. This capability allows businesses to scale their digital marketing efforts efficiently while ensuring content relevance and user engagement.
Challenges and ethical considerations
Data privacy and security concerns
Personalization hinges on vast data collection, raising concerns about data security and user privacy. Regulatory frameworks like GDPR and CCPA mandate strict data protection protocols, compelling organizations to implement robust encryption, anonymization techniques, and transparent data policies. Companies must balance personalization benefits with ethical data usage to maintain user trust.
Algorithmic bias and fairness
Machine learning models may inadvertently reinforce biases if trained on unbalanced datasets. Biased AI personalization can lead to exclusionary experiences, affecting marginalized demographics. Addressing bias requires continuous model audits, fairness-aware algorithms, and diversified training datasets.
Over-automation and user autonomy
Excessive reliance on AI-driven personalization may strip users of autonomy by limiting exposure to diverse content. Filter bubbles—where AI systems prioritize familiar content—can restrict discovery and serendipity. Designers must strike a balance between automation and manual customization options, allowing users to override AI-generated recommendations when necessary.
Future outlook: Balancing automation and human-centric design
As AI-driven personalization continues to evolve, the focus must shift toward explainability, transparency, and user control. Future advancements in federated learning and edge AI will enhance privacy-preserving personalization, minimizing reliance on centralized data storage. Additionally, human-centered design principles should guide AI deployments, ensuring technology complements rather than dictates user experiences.
By integrating ethical AI practices and maintaining a balance between automation and user agency, AI-driven UI personalization can enhance digital interactions while respecting user preferences and privacy.