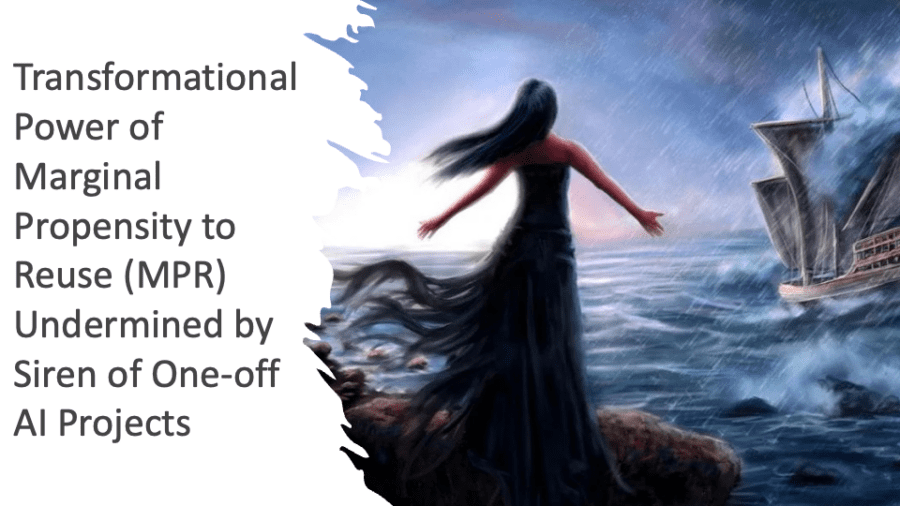
In the world of physical assets, the more something is used, the more it depreciates—it wears out, loses value, and eventually needs to be replaced. But in the digital economy, the opposite is true. Welcome to the Marginal Propensity to Reuse (MPR). I introduced MPR in the blog “Mastering the Data Economic Multiplier Effect and Marginal Propensity to Reuse.”
Marginal Propensity to Reuse (MPR) is the economic concept that digital assets (data, AI models, and analytics) appreciate in value as they are reused across multiple applications (Figure 1).
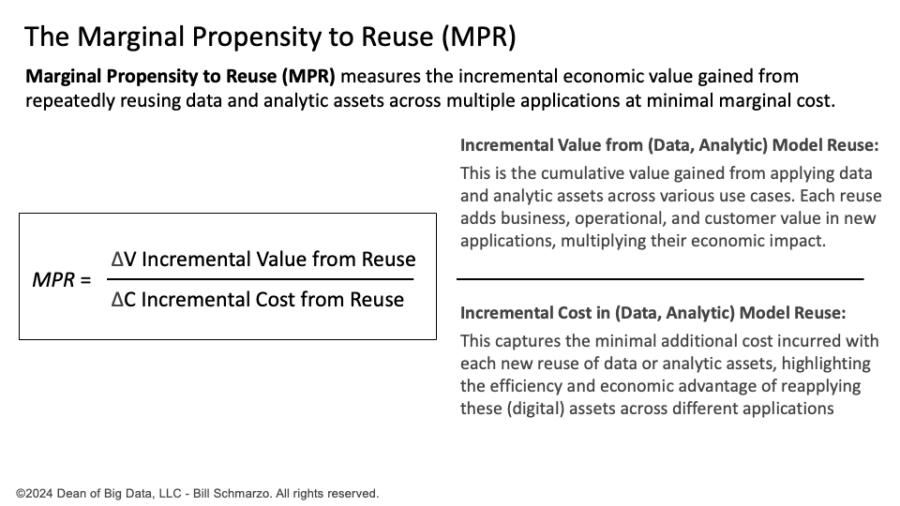
Figure 1: The Marginal Propensity to Reuse (MPR)
MPR is one of the most transformational economic factors of the digital age, and here’s why:
#1) The Exponential Value of Digital Assets. Unlike traditional physical assets, digital assets (data, analytics, AI models) don’t depreciate with use—they appreciate as they are reused across multiple use cases or applications. This breaks the classic constraints of scarcity and transactional limitations in traditional economics. For example, a physical asset like a factory machine wears out the more it is used. However, a knowledge-based asset like a dataset or an AI model becomes more valuable when reusing it across different scenarios.
💡This fundamental difference between physical and knowledge-based assets makes MPR one of the most economically significant forces in the digital economy.
#2) The Network Effect of Reuse. MPR enables a network effect where the same dataset, algorithm, or AI model generates incremental returns across multiple use cases. For example, a product recommendation algorithm built for Amazon’s e-commerce store can also be reused for AWS clients, Alexa’s voice shopping, and Whole Foods’ supply chain optimization. Each reuse compounds value, making the original investment in the model exponentially more profitable.
💡This network effect, where reuse multiplies value with each iteration, separates digital-first companies like Google, Amazon, and Tesla from traditional enterprises.
#3) MPR Drives the Data Economic Multiplier. Your Data Economic Multiplier (DEM) concept already highlights that the more a dataset is used across multiple use cases, the more valuable it becomes. MPR turbocharges this by emphasizing reusability as the key lever of digital asset monetization. For example, a medical AI model trained to detect lung cancer in X-rays can be reused for detecting pneumonia, identifying tuberculosis, and assisting radiologists in multiple hospitals. Each additional use case increases the economic return on the original AI investment, reducing marginal costs while maximizing marginal returns.
💡This ability to reinvest the same intellectual capital in multiple applications at zero marginal cost is the ultimate efficiency driver in the digital economy.
#4) MPR is the foundation of the AI revolution’s economy. It drives the entire AI and machine learning value proposition as AI models continue to learn and improve with each new application. Pre-trained AI models, such as OpenAI’s GPT and Google’s BERT, can be fine-tuned for specific industries—like healthcare, finance, cybersecurity, and marketing—without starting from scratch. This AI-powered automation allows decision-making intelligence to be reused across multiple domains.
💡The fact that AI doesn’t “wear out” but instead compounds in value makes MPR one of the biggest economic drivers of AI’s success.
#5) MPR Drives Competitive Differentiation. MPR is rewriting the game of competitive advantage in the digital economy. Traditional competitive advantages (cost leadership, differentiation, economies of scale) are based on physical constraints. Knowledge-driven companies win by leveraging MPR—exploiting data, AI, and analytics across multiple domains at near-zero marginal cost. For example, every mile driven by a Tesla vehicle feeds data into a shared AI system. That same data is reused to improve Autopilot, navigation, traffic predictions, fleet management, energy optimization, and battery efficiency.
💡This is why companies that master MPR gain an insurmountable competitive edge, while those stuck in traditional economies of scale struggle to keep up.
Given all the powerful incentives for organizations to embrace MPR, why do they continue to screw it up? The siren’s song of a quick fix is too compelling to ignore. And these quick-fix solutions (“Get me something up and running now!”) lead to the archenemies of MPR – Orphaned Analytics and Data Siloes.
The Archenemies of MPR: Orphaned Analytics and Data Siloes
Despite the overwhelming economic power of MPR, many organizations are stuck in a broken mindset—rushing to build one-off, standalone AI projects to please management that fail to enable data and analytics reuse. This leads to:
- Data silos are isolated data systems that hinder the sharing, integration, and cross-functional AI learning, limiting innovation and efficiency.
- Orphaned analytics are disconnected AI projects that fail to deliver value due to a lack of reuse.
One example of this problem is today’s rush to deploy AI chatbots. AI-powered chatbots are a perfect example of how organizations sabotage MPR by investing in single-use AI systems instead of engineering shared intelligence platforms.
- They build multiple AI chatbots—one for customer support, another for HR inquiries, another for IT help desks, etc.
- Each chatbot is trained on different datasets, developed in separate silos, and lacks shared learning across the organization.
- Instead of one AI system that continuously learns and improves, companies end up with fragmented, underperforming chatbots that require constant retraining and redevelopment.
One-off AI projects challenge MPR’s value creation accelerator. Instead of enhancing intelligence, organizations are developing disconnected AI solutions that fail to optimize the economic potential of reuse and learning (Figure 2).
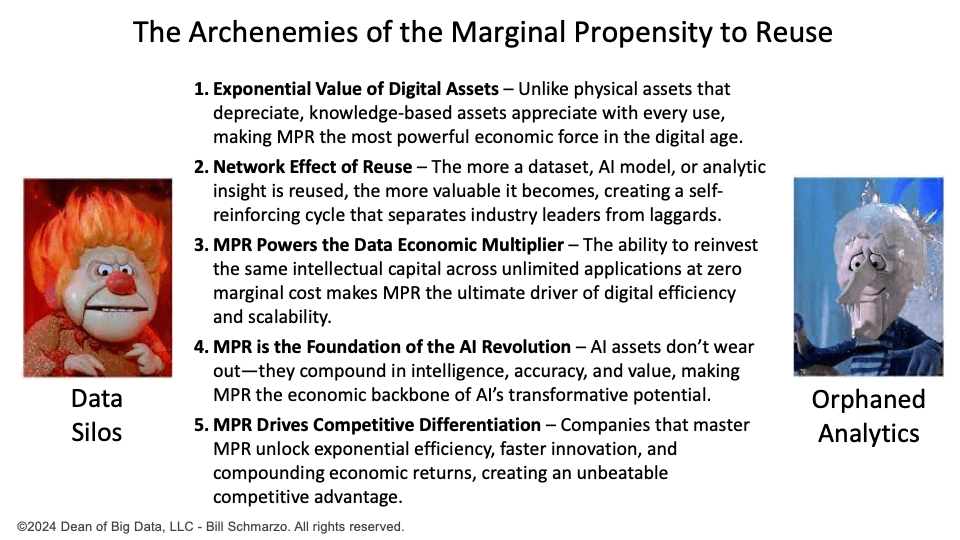
Figure 2: The Archenemies of the Marginal Propensity to Reuse
AI Chatbot Development: The Right vs. Wrong Approach
AI chatbots provide a robust, human-like user experience crucial in accelerating the adoption of AI models across the organization and improving personalized engagement with customers and constituents. However, there are right and wrong ways to approach these powerful enablers.
Best Practice | ❌ Wrong Approach | ✅ Right Approach | 🔹 Example |
1. Build a Core AI Model, Not Isolated Chatbots | Building separate chatbots for customer service, IT, HR, and sales, each with unique datasets and architectures. | Developing a single AI-powered conversational engine that can be fine-tuned for multiple functions. | Amazon Alexa: Instead of separate AI assistants for music, shopping, and smart home control, Alexa is one AI system that continuously learns and improves across tasks. |
2. Use Transfer Learning for AI Adaptability | Training each chatbot from scratch with new datasets, leading to redundant learning and inefficiencies. | Leveraging transfer learning to apply previous AI knowledge to new applications, reducing training time and improving efficiency. | Google’s BERT AI Model: Initially trained for search engine ranking, later repurposed for chatbots, text summarization, and sentiment analysis—one AI model, multiple use cases. |
3. Centralize AI Knowledge in a Model Hub | Allowing different departments to create separate, unconnected AI chatbots, leading to data silos. | Establishing a centralized AI Model Hub to standardize AI development and enable knowledge sharing across departments. | Tesla AI Strategy: Every Tesla feeds data into a shared AI model, improving every vehicle on the road instead of running multiple isolated AI systems. |
4. Align Chatbots to Business Objectives | Building AI chatbots just because “everyone else is doing it”, without a clear business strategy. | Designing AI chatbots that integrate across departments, align with business goals, and compound intelligence over time. | Bank of America: Erica is a unified AI assistant that handles customer service, fraud alerts, account insights, and financial guidance and aligns with customer service and fraud business objectives. |
Here are the key questions to ask to ensure that your AI Chatbots enable the marginal propensity to reuse:
- Will this chatbot integrate with existing AI systems to maximize reuse?
- Can this chatbot learn and improve across multiple business units?
- Is this AI chatbot a long-term investment or just a trendy experiment?
If the answer to these is “no,” rethink the approach. Resist the false urgency for the quick win that jeopardizes the longer-term economic potential enabled by MPR.
Senior Management MPR Checklist: Unlocking the Full Potential of AI and Data
Too many organizations are squandering the economic potential of AI and analytics by building one-off, siloed solutions that don’t leverage the Marginal Propensity to Reuse (MPR), which drives the compounding of value across use cases. This checklist helps senior executives, CIOs, and AI leaders ensure their organization fully utilizes MPR to maximize AI’s return on investment (ROI).
🔹 AI and Data Strategy
- Do we have a company-wide AI strategy that prioritizes reuse and shared learning across multiple functions?
- Are we resisting one-off AI projects and instead building AI solutions with long-term adaptability?
- Have we identified cross-functional AI use cases to ensure maximum organizational reuse?
🔹 AI Model Development
- Are we building a centralized AI Model Hub to ensure models are shared, refined, and reused?
- Do we leverage transfer learning and pre-trained AI models instead of retraining from scratch?
- Is your AI architecture designed to scale and improve over time rather than being locked into static applications?
🔹 Data Strategy & Governance
- Are we treating data as an economic asset and ensuring it is accessible, reusable, and well-governed?
- Have we eliminated data silos that prevent AI systems from learning across different use cases?
- Do we have a data-sharing framework that allows AI systems to continually learn and improve?
🔹 AI Investment & ROI
- Are we measuring the long-term economic impact of AI reuse instead of focusing only on short-term project success?
- Do we have MPR-driven KPIs that track how AI investments compound in value over time?
- Are we evaluating AI projects based on scalability, adaptability, and reuse potential, not just immediate results?
🔹 Organizational Readiness
- Have we established an AI Center of Excellence (CoE) to ensure best practices, governance, and knowledge-sharing?
- Are we encouraging cross-functional collaboration between IT, business units, and data science teams?
- Do we have the right talent and mindset to build AI for long-term impact rather than short-term gains?
AI success in the digital economy isn’t about building the most AI models—it’s about engineering composable, reusable, extensible AI models that continuously learn, scale, and compound in value. Organizations that master MPR will have AI systems that improve exponentially, reduce costs, and generate long-term competitive advantages.
The future belongs to those with the vision and patience to design AI for continuous learning, reuse, and adaptability. Will your company be one of them?