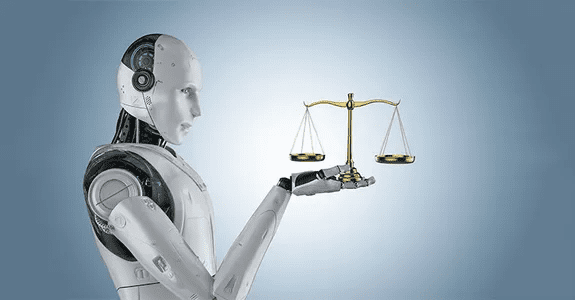
Data is at the heart of any system. Today, AI is largely used to maintain large volumes of data and make decisions from it. AI is even being used in sensitive areas such as healthcare, hiring, and criminal justice which has sparked a huge debate in recent years.
The debate is about whether AI can be trusted in decision-making. Is it susceptible to biases? It’s important to note that human decisions can also be biased. But the question is can AI give less biased outcomes compared to humans? Or will they amplify and perpetuate these biases? And if it’s true that AI is susceptible to biases, how can we overcome it?
In this blog, we will discuss how to address bias in AI. We’ll explore the different types of biases that can exist in AI systems, the causes behind the bias, and how to prevent or mitigate it.
What is AI bias?
AI bias is a term referring to the prejudiced or discriminatory results of AI algorithms. These results may be unfair or discriminatory towards specific groups or individuals, based on factors like race, gender, age, or socioeconomic status. This can happen when the algorithm is fed biased data or when the developers make biased assumptions during the algorithm development process.
Types of AI bias
1. Data bias
Data bias occurs when the data used to train an AI system does not match the population it is meant to serve. This can result in the AI system making biased decisions or recommendations that disadvantage certain groups of people.
For example, a facial recognition algorithm may produce biased results when it is fed more white faces but used on individuals of different races.
2. Algorithmic bias
Algorithmic bias occurs when the algorithm produces biased results. This can happen due to various factors, such as the selection of the training data, the choice of features, or the use of biased models.
3. User bias
User bias refers to the occurrence of biased output by AI algorithms. This can happen when users enter discriminatory or inaccurate data that reinforces the existing bias in the system.
4. Technical bias
When the technology used is not apt for the machine learning model, it can introduce technical bias in the system. For instance, the data set may be limited due to a lack of computing power or storage capacity.
This can lead to the algorithm being less accurate and producing biased results due to the lack of exposure to a wide range of data.
How to mitigate AI bias?
You must use different techniques at different stages of the process to ensure the impartiality of AI systems. This includes pre-processing, algorithmic, and post-processing methods. By using these methods, we can help mitigate bias in AI, promoting a more inclusive environment for everyone.
- Pre-processing techniques: This technique involves transforming the input data before it is fed into the machine learning program. The dataset is more diverse and representative, which helps mitigate bias.
- Algorithmic techniques: You can also adjust the machine learning algorithm itself to mitigate bias.
- Post-processing techniques: These techniques analyze the output after training and remove bias from machine learning algorithms.
How to tackle AI bias?
Tackling AI bias requires a thoughtful approach. It’s better to keep in mind that AI bias has its roots in human bias. So, it’s important to address the root cause and remove these biases from the data set. However, it’s not as simple as deleting labels or removing the protected classes.
1. Accept the existence of bias
Accept that bias exists. This involves recognizing that AI systems can be biased and can have serious consequences.
2. Build a team
AI systems should be built with fairness and inclusivity in mind. So, you must have a diverse team from different backgrounds, experiences, and perspectives working on their development. Having a diverse team can help you identify potential sources of bias in your AI systems that you may not have considered otherwise.
A great example of a top AI software developed by a diverse team is text-to-speech software. It does what it says. It expertly converts text inputs to different voice types as per your choice.
3. Identify potential biases
Next, techniques like data auditing or algorithm auditing should be used to identify potential biases in the data, algorithms, and decision-making process used by AI systems.
Auditing is a term referring to analyzing the functions of a system and making changes as required to attain optimal performance. Though not directly related to AI, you can get a fair idea of what an audit looks like from this Backlink Audit blog, which provides a comprehensive guide to analyzing and optimizing your backlink profile effectively.
4. Establish an ethical framework
Now, develop an ethical framework for your AI system that outlines the values and principles that should guide the use of the technology. This will help you identify and address ethical concerns and make sure that the system is aligned with your ethical standards.
5. Test your AI systems
Use diverse data sets to test your AI system. This will help you identify any biases and ensure your system is fair and reasonable for all. Test your system using data that represents the people who will be impacted by it. This way, you can easily find any biases and work to correct them.
6. Involve stakeholders
Involve stakeholders in the development process. This will help identify and correct any bias easily while at the same time, it will build trust. The stakeholders can also guide you on their needs and concerns and make sure the AI system meets them.
7. Conduct regular audits
AI models are not static and can change over time, so you should monitor your system’s bias on a regular basis. Regular examination will help identify any new biases that may emerge as your system evolves.
8. Learn and adapt
You must remember that finding and addressing biases in AI is an ongoing process. As new biases are identified and newer techniques are built to mitigate them, you must remain alert and updated to revise your algorithm accordingly.
Conclusion
Biases in AI algorithms are quite common and it’s important to remember that there’s no one-size-fits-all approach solution. However, by adopting a holistic approach and using a combination of tools and techniques, we can eliminate biases to a great extent.
It’s however, important to remember that AI systems are not stable and new biases can arise with time. So, it’s important to stay updated and revise your AI algorithm regularly. That way, new biases can be found easily and you can mitigate them easily.