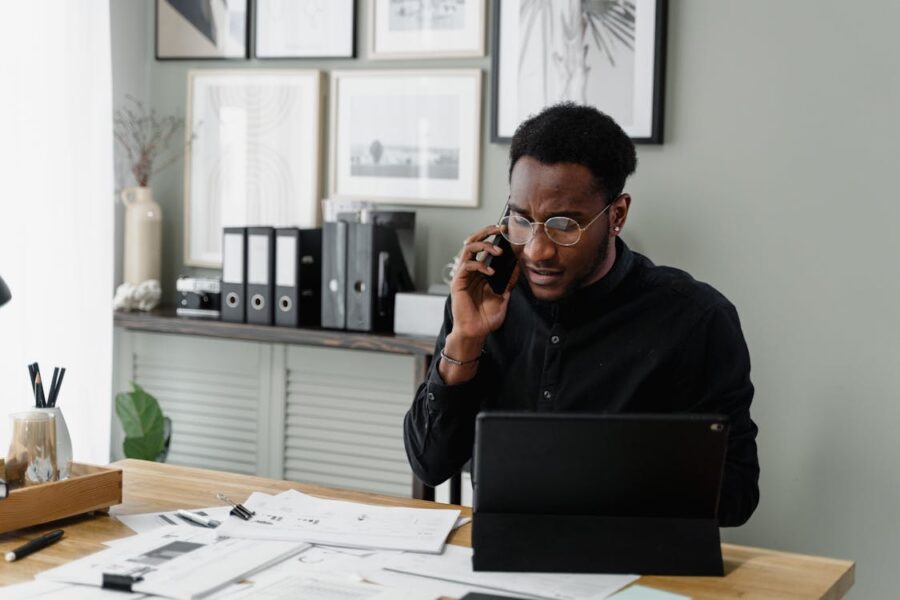
Image source: https://www.pexels.com/photo/man-in-black-jacket-using-black-laptop-computer-6693637/
Ensuring pay stubs are accurate is crucial – for both companies and employees. After all, errors in payroll can lead to financial losses, compliance issues, and even strained employee/employer relationships.
This is where data science steps in. It is transforming the way businesses verify pay stub details. From spotting accidental errors to detecting fraud, its applications are varied and powerful.
So, let’s learn about the role that data science can play in verifying the accuracy of pay stubs.
The role of data cleaning in pay stub verification
Data cleaning is essential for accurate pay stub verification. In payroll systems, messy data – such as duplicate entries, formatting errors, or incomplete records – can lead to inaccuracies in employee payments and tax reporting.
By using data science techniques like deduplication and standardization, businesses can ensure that payroll data remains consistent across all platforms. This process identifies irregularities within pay stubs caused by human error or outdated information.
For example, a mismatched Social Security number or misaligned deduction entry will get flagged during the cleaning phase. Once corrected, the clean dataset becomes a reliable source for further analysis. Accurate pay stub audits rely on this foundational step to prevent calculation errors and compliance issues.
Using predictive analytics to spot payroll discrepancies
Predictive analytics can play a key role in identifying errors and inconsistencies within payroll data. By analyzing historical pay records, these tools detect patterns that indicate typical payment behaviors and flag outliers.
For instance, if an employee’s hours or deductions suddenly deviate from their usual range without explanation, the system raises a red flag for further review. It processes large volumes of payroll entries quickly, pinpointing potential issues like missed overtime payments or incorrect tax withholdings. This proactive approach allows organizations to address discrepancies before they escalate into larger problems.
With accurate forecasting models powered by data science, companies can ensure timely corrections and improve overall payroll reliability for employees and management alike.
Automated fraud detection in payroll
Fake pay stubs are more common than you’d think. They’re often used by unscrupulous people to manipulate income verification processes. Well, data science provides the tools to spot these fakes efficiently.
Machine learning algorithms analyze genuine payroll patterns, like tax deductions, employer details, and formatting styles. When anomalies appear – such as unrealistic earnings ratios or mismatched fonts – the system flags the document.
Plus, AI-powered tools can cross-reference reported information against trusted databases to verify accuracy. These methods streamline fraud detection by automating complex comparisons that would otherwise require extensive manual effort. This ensures organizations identify and prevent fraudulent activities while maintaining the integrity of payroll systems and decision-making processes tied to verified records.
Remember, using inaccurate pay stubs (that are perhaps created with a fake paystub generator) to commit fraud is a crime. But there are reputable online paystub generators available, which can be used to quickly create pay stubs for proof of income or employment.
How data visualization enhances pay stub audits
Data visualization tools simplify complex payroll data, making pay stub audits more efficient. By presenting patterns and trends through graphs, heatmaps, or dashboards, discrepancies become easier to identify. For example, an employee’s earnings history can be plotted to highlight sudden anomalies like unexplained deductions or irregular salary spikes. Visual reports allow HR teams to quickly interpret deviations that might otherwise go unnoticed in raw datasets.
These tools also help break down details such as tax contributions and benefits into digestible formats for both employees and auditors. With clearer insights derived from visualized data, organizations improve their ability to maintain accurate payroll records while increasing transparency across their systems.
Leveraging statistical models for payroll compliance checks
Lastly, statistical models play a critical role in verifying pay stub accuracy and ensuring compliance with payroll regulations. These models evaluate data against predefined rules, such as tax thresholds, overtime calculations, and benefits distributions.
For instance, regression analysis can identify discrepancies between reported deductions and legal limits. Similarly, hypothesis testing checks whether payroll figures align with expected values or signal potential errors. Outliers are flagged immediately for deeper investigation.
These techniques not only streamline audits but also minimize the risks of regulatory penalties due to inaccurate reporting.
By applying statistical methods effectively, organizations will uphold compliance standards while maintaining consistent and accurate payment practices across all levels of their workforce operations.