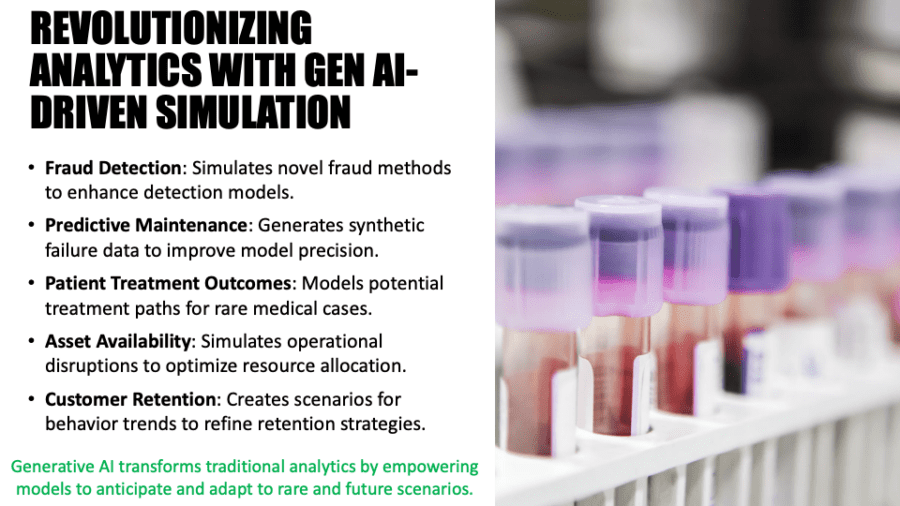
While much of the focus on using Generative AI (GenAI) has been to improve worker productivity with tools like OpenAI’s ChatGPT and Microsoft’s Copilot, GenAI offers so much more. I wrote about the expansive potential of GenAI to enhance your existing analytic models in my blog, “How to Transform Your ML Models with Generative AI.” In that blog, I highlight how GenAI can be leveraged as a “mix-in” to enhance the relevance and accuracy of your existing analytic models (Figure 1).
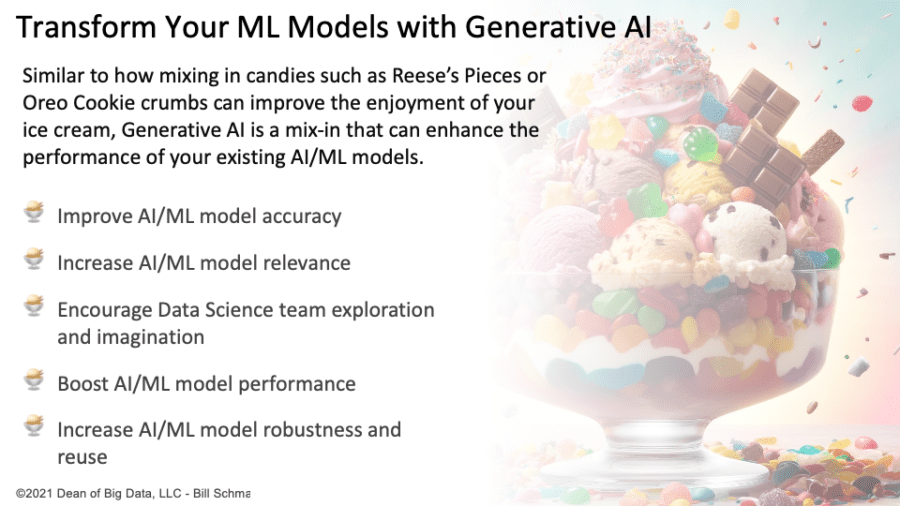
Figure 1: Transform Your ML Models with Generative AI
In this blog, I aim to expand on that conversation by providing real-world examples of how GenAI can simulate rare and hypothetical “what-if” scenarios to improve the relevance of your existing ML models. These simulations can significantly enhance current AI/ML models, offering deeper insights and greater accuracy in use cases such as fraud detection, predictive maintenance, patient treatment outcomes, asset availability, demand forecasting, and customer retention. By enabling subject matter experts to brainstorm and simulate hypothetical what-if scenarios, GenAI allows organizations to enhance their analytic models for a wider array of potential operational challenges, making them more robust, adaptive, and effective.
GenAI as an Event Simulator
GenAI is invaluable for simulating potential events for which no historical data exists. GenAI enables organizations to train models on a broader range of scenarios by generating synthetic data replicating the characteristics of these hypothetical scenarios. This capability is crucial for applications like fraud detection, predictive maintenance, and disaster preparedness, where real-life data on rare occurrences is often limited. GenAI-driven simulations allow organizations to test “what-if” scenarios, enhancing their ability to prepare for high-impact events and optimize response strategies.
Below are some industry-specific use cases that demonstrate how organizations can harness GenAI to generate synthetic data for high-stakes scenarios:
Financial Services: Simulating New Fraud Scenarios
- Use Case: Financial institutions can use GenAI to simulate new fraudulent activities, creating synthetic data to model the impact and refine their fraud detection algorithms. For instance, when a novel fraud method is uncovered, synthetic transaction data can be generated to train machine learning models to detect these anomalies in real-time. This allows financial service organizations to avoid emerging fraud tactics and rapidly implement proactive countermeasures.
- Impact: By synthesizing variants of fraudulent behavior, organizations can improve fraud detection accuracy and reduce false positives, protecting clients and reducing potential financial losses.
Insurance: Modeling Hurricane Impacts with Synthetic Data
- Use Case: Insurance companies can use GenAI to simulate extreme weather events, such as a hypothetical “Hurricane Milton” affecting Gulf Coast cities like Mobile, Alabama and Biloxi, Mississippi. Synthetic data replicates storm paths, flooding patterns, and structural damage. It enables insurance companies to project potential claims, optimize coverage terms, and evaluate their risk exposure in areas vulnerable to catastrophic events.
- Impact: This proactive use of GenAI in disaster modeling supports more accurate policy pricing and better customer preparedness and enables insurers to allocate resources efficiently for post-event claims management.
Government Agencies: Simulating War, Economic, and Pandemic Scenarios
- Use Case: Government agencies can use GenAI to simulate rare, large-scale scenarios such as potential wartime events, economic crises, or pandemics. Synthetic data representing hypothetical infection spread patterns, economic downturns, or even logistics during conflict enables agencies to run response drills and refine national preparedness strategies. This is crucial for ensuring effective resource allocation and communication channels during real crises.
- Impact: These simulations allow governments to stress-test response plans, develop rapid-response capabilities, and make informed policy decisions that reduce the effects of such events on society.
Healthcare: Rare Disease Progression and Pandemic Simulation
- Use Case: In healthcare, GenAI can simulate the progression of rare diseases or sudden outbreaks (e.g., during COVID-19). By creating synthetic patient data, hospitals can prepare for various infection scenarios, assess resource demands, and refine treatment protocols without relying solely on limited real-world data.
- Impact: This application helps bolster the healthcare system’s response capacity, ensuring facilities are better prepared with equipment and personnel allocation. This ultimately improves patient outcomes and reduces mortality rates.
Manufacturing: Predictive Maintenance for Rare Equipment Failures
- Use Case: In sectors like energy or aerospace, where specific types of equipment failures (e.g., nuclear reactor malfunctions or airplane engine issues) are infrequent, GenAI can generate synthetic failure data. This data supplements predictive maintenance models, allowing them to learn from a broader set of rare scenarios and thus enhance their reliability.
- Impact: GenAI allows companies to foresee and prevent potentially catastrophic failures, improving safety, reducing maintenance costs, and minimizing operational downtime.
Public Safety and Disaster Preparedness: Emergency Response Simulations
- Use Case: Law enforcement and emergency response teams can use GenAI to simulate emergency scenarios, such as large-scale evacuations or earthquakes, which may only occur infrequently. This allows them to test response times, communication protocols, and resource allocation in controlled, synthetic environments.
- Impact: These simulations enhance the readiness and responsiveness of public safety teams, ensuring better-managed resources and improved outcomes during real-life emergencies.
By simulating diverse, high-impact scenarios, GenAI enables decision-makers across various industries to develop data-informed strategies, ensuring resilience and adaptability when the unexpected occurs.
Mastering the Four Types of Artificial Intelligence (AI)
GenAI’s full economic potential can be unleashed when you consider the role of GenAI in turbocharging the other types of analytics – predictive, causal, and autonomous.
- Predictive Analytics. GenAI enhances predictive analytics by generating synthetic data for rare or hypothetical scenarios, allowing models to anticipate broader outcomes. This increases forecast accuracy and makes predictions more relevant to complex real-world situations. Additionally, by creating personalized data profiles, GenAI enables predictive models to produce insights tailored to specific needs, adding meaningful value beyond generalized predictions.
- Causal Analytics. GenAI allows for the simulation of “what-if” scenarios, helping organizations test interventions and uncover the true drivers of outcomes without relying solely on real-world data. This capability provides more precise, actionable insights and allows causal models to simulate ethical and social impacts, making recommendations more responsible and comprehensive.
- Autonomous AI. GenAI is a powerful training tool that creates synthetic environments that expose systems to diverse and challenging conditions. This enhances their adaptability in real-world situations, while simulations of ethical dilemmas teach autonomous AI to make responsible choices. With continuous GenAI-generated data, autonomous systems can refine their decisions to align with user needs and social expectations, ensuring more relevant and accountable outcomes.
Integrating Generative AI (GenAI) with predictive, causal, and autonomous analytics unlocks its full transformative potential. GenAI broadens predictive models with synthetic data, deepens causal analytics with actionable “what-if” scenarios, and trains autonomous systems to adapt ethically to diverse challenges. Together, these synergies enable more robust, relevant, and responsible AI-driven solutions, driving innovation and competitive advantage across industries.
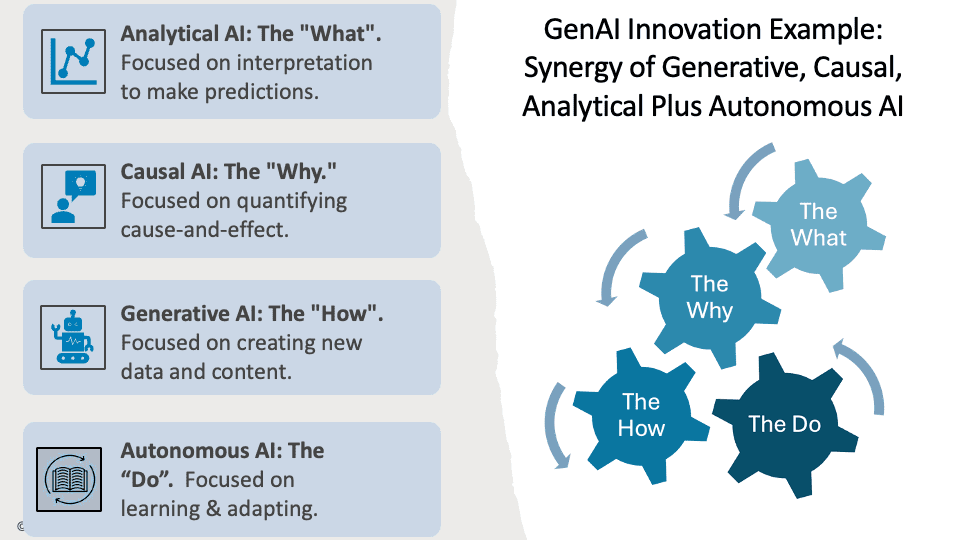
Figure 2: Blending the Four Types of AI
Generative AI (GenAI) amplifies the capabilities of predictive, causal, and autonomous analytics. It broadens predictive models with synthetic data, enhances causal models through actionable “what-if” scenarios, and trains autonomous systems to adapt to broader and more diverse challenges. Together, these synergies make AI-driven strategies more robust, relevant, and responsible, driving innovation and competitive advantage across industries.