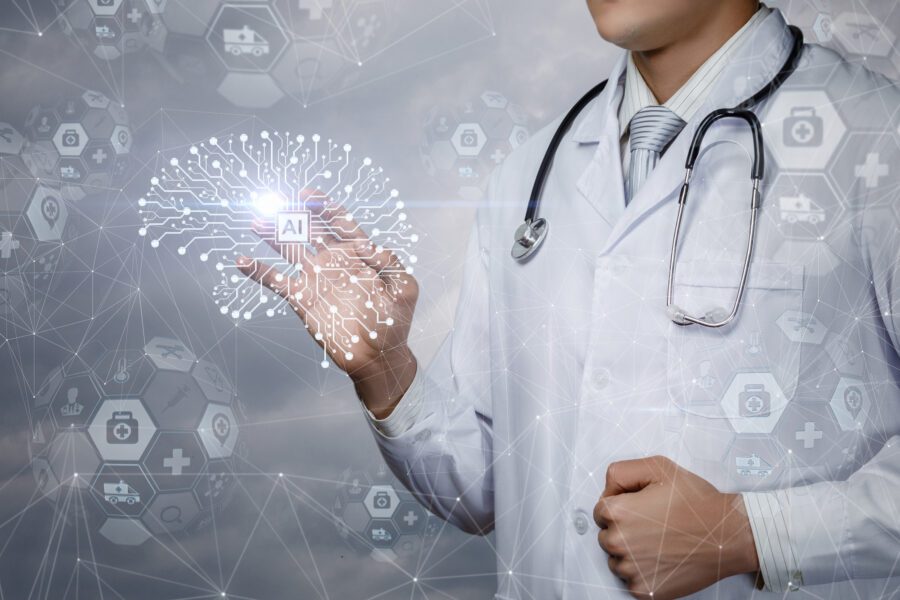
The medical field has some of the greatest potential for artificial intelligence (AI) implementation. The right algorithms and data could identify hard-to-pin illnesses and create new recipes for lifesaving medicines. This urgency led the famous Mayo Clinic to devise a uniquely constructed AI for next-generation medical attention — hypothesis-driven AI.
What is hypothesis-driven AI?
Traditionally, an AI takes information and categorizes, recognizes, or synthesizes it. These use cases rely on hard, clarified data, where information integrity is a priority. In practice, unbiased datasets are invaluable to some applications but limit others.
Hypotheses and in-progress research fuel sectors like health care. AI may need to learn how to view health information because it requires critical context. How could an AI generate a solution for a patient with a recently discovered ailment without first knowing the guesses and research attempts doctors have made?
Mayo Clinic wanted to fix this by giving AI access to current knowledge and theories in medical science. This way, the AI will know what doctors presently understand about a disease, medicine, or concern and take it into consideration.
The researchers announced their first findings in an oncological study, suggesting an AI like this could advance cancer research and make diagnostics, treatmen,t and development more agile and patient-specific.
How is this better than conventional AI?
Regular AI will still have a place in medical care. Hypothesis-driven AI will be more useful for tasks with more abstract concepts. Before suggesting medical advice, an AI should know what hypotheses have failed and what the most successful answers have been so far.
This process would be helpful in instances like finding the right treatment plan for a patient with a rare and underresearched illness. It could resemble hard AI models, which are better at replicating human-like thinking.
Because the AI understands the nature of hypotheses, the questions it produces and the tasks it performs will have more productive results. A conventional AI would be bound by its strict dataset and have inaccurate or nonsense responses. The AI would feel outdated because it would not have existing knowledge to inform its determinations. Adding hypotheses to the dataset will make answers easier to interpret and machine learning-based training efficient.
The tests also show that hypothesis-driven AI requires fewer resources to power than other algorithmic setups. The computational requirements for traditional AI models are extremely high, and this version of AI does not have to wade through as much data.
Despite these advantages, the AI is imperfect. Bias is a constant concern for all algorithms, but hypothesis-driven versions may be more prone to it. As the AI trains on these thoughts, it could skew an informed hypothesis into a supposed fact.
Hiring numerous contributing researchers can help keep the AI balanced. The diverse perspectives will also prevent it from developing a limited view. These influences will make AI more effective at identifying patterns and considering all health factors, especially for developing and threatening diseases.
How do medical professionals use it?
These are the most likely ways healthcare professionals would use this AI design.
Educating other medical professionals
Hypothesis-driven AI can become inaccessible to practitioners who are not contributing to the dataset. They would be unfamiliar with the training progress since it is always updated with new findings. Sharing these types of AI with other medical professionals could expedite knowledge sharing. Publicizing insights rapidly with AI could boost treatment speeds for the most vulnerable patients.
Sharing information could solve major oversights in areas like cancer research and disease funding. Consider how lung cancer accounted for 18% of global deaths but only 5.3% of research funding investments.
Alternatively, breast cancer receives a nearly equal balance of funds compared to how many cases there are yearly. Hypothesis-driven AI could mend some research gaps until investments become more balanced.
Designing treatment strategies
Numerous patients need personalized attention, even though doctors may default to the same prescriptions and treatment plans. This AI could consider the patient carefully based on the medical staff’s understanding of them and their condition.
Whether the person is assigned immunotherapy or surgery, the AI might predict risks, timelines or benefits for a specific recovery plan. This personalization is critical for fields like oncology, where cancer diagnoses and experiences vary widely from person to person. It would also consider phenomena like antibiotic resistance, which causes up to 70,000 deaths yearly.
Parsing cancer data more comprehensively
Every cancer diagnosis is one-of-a-kind. While experts know how cancer cells work, clinicians may not know how they react in an individual’s body given their health records, cell makeup, diet, genetics, and more information. These factors make the variability of cancer treatment broader than any healthcare issue. Fortunately, the research claims tests were successful in predicting the following:
- Patient stratification
- Tumor classification
- Cancer gene discovery
- Drug response prediction
- Tumor spatial organizations
It is important to leave the guesswork
Even though hypotheses are inherently malleable, healthcare would benefit from including them in their AI models. It would make treatment plans more customized and information-sharing between medical professionals easier and more transparent.
To care better for all patients, medical facilities should look to the Mayo Clinic study as a model for leveraging more profound relationships with AI in the future.