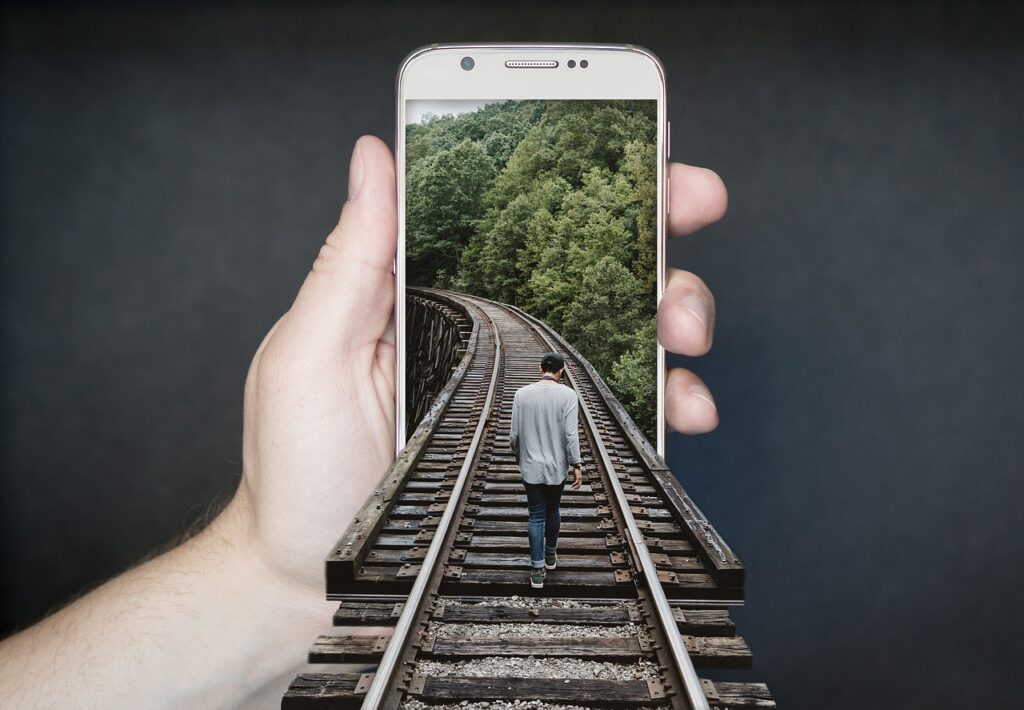
Image by David from Pixabay
Mobile phones make it possible to secure and manage personal data on-device, which opens up a novel opportunity for both phone owners and device manufacturers: AI personalization via a data resource that stays on the phone. With the right design, personal knowledge graph on-device could provide contextualization while at the same time ensuring user data security and opening the door to an own-your-own-data paradigm.
Samsung’s hybrid AI on phones
Device makers have been exploring these possibilities and making investments. Samsung in July 2024 acquired Oxford Semantic Technologies (OST), a spinout of the University of Oxford that offers a standards-based knowledge graph development platform and reasoning engine called RDFox. Knowledge graph developers have the option on RDFox to choose either OWL (Web Ontology Language) or Datalog for their ontology (semantic graph data modeling) efforts.
Samsung plans to leverage both the speed and safety advantages of on-device data storage, management and processing in its personalized AI efforts. In a contributed article on the Samsung Newsroom site posted on November 6, 2024, Samsung Research Global AI Center Director Dae-hyun Kim states,
“We want to provide new experiences through generative AI that goes beyond simply processing or analyzing data and creates unique results according to user needs. In particular, we plan to develop knowledge graph technology, one of the key technologies for personalized AI, and organically connect it with generative AI to support customized services for users.
“In addition, Samsung Electronics is applying hybrid AI to efficiently implement AI experiences. Hybrid AI is a technology that provides a balance of speed and safety by using on-device AI and cloud AI together. By utilizing on-device AI, which has the advantage of fast response speed and strong privacy protection that operates within the device, and cloud AI, which provides various functions based on massive data and high-performance computing, together, it is possible to provide the optimal AI experience in various environments and conditions.”
Apple’s latest Siri upgrade
Apple’s Siri has a long history that predates the iPhone era, beginning with the Defense Advanced Research Project Agency’s funding of the Cognitive Assistant that Learns and Organizes (CALO) project at Stanford Research Institute in 2003. SRI researchers Dag Kittlaus, Tom Gruber and Adam Cheyer on the CALO project launched an SRI spinoff called Siri and released the Siri app for the iPhone in 2010. By February 2011 Apple had acquired the startup and released its own beta version of Siri.
Kittlaus, Gruber and Cheyer designed an early version of a knowledge graph into the foundation of the original Siri “Virtual Personal Assistant” and layered API services on top of the graph. In a 2010 talk, Gruber described the Siri architecture using the term “semantic web.”
“…it’s the connectivity of that data,” said Gruber, ”that’s going to be the tipping point for these intelligent applications. What we call the Gigantic Join is when you take that structured data from one source and structured data from another source and combine them to produce a new service that was never there before.
“Speaking of service, it’s not just data. I was very happy to be part of the early days of the semantic web. And I think it’s fantastic, but I think in 2010, the next level is here. It’s Semantic Web with APIs on top, and it’s the APIs that deliver services that’s going to make these intelligent applications happen.
“And in fact, just like data needs to be connected. Services need to be combined. So intelligent applications of the future of today are going to be masters of mashed up. We call it the mother of all mashups.”
By 2012, Google had coined the term “knowledge graph” to describe a closely related approach, a year and a half after it had acquired semantic web startup Metaweb.
14 years later, though there’s been some hemming and hawing at times, Apple’s Siri unit as recently as September 2024 has continued to hire ontologists.
Apple also recently funded more of its own knowledge graph research with KGLens, a framework that facilitates alignment between large language models (LLMs) on the statistical side and parameterized knowledge graphs on the symbolic side of AI. (For a comparable approach to Apple’s LLM-KG alignment from the academic community, see my November 4 2024 post “A feedback loop alternative to RAG that aligns LLMs with knowledge graph models” for more information.)
It’s all about the data
The buzz surrounding generative AI continues unabated. The reason gen AI is so compelling is because the power of the data becomes so evident on the front end due to the friendly natural language-oriented user experience and the size and diversity of LLM datasets.
Though Apple and Samsung lead with generative AI in their messaging about their AI-enabled phones, it’s clear on-device knowledge graphs are a key way these companies hope to deliver better accuracy, personalization and data security than the central LLMs we’re all so familiar with have been able to thus far.