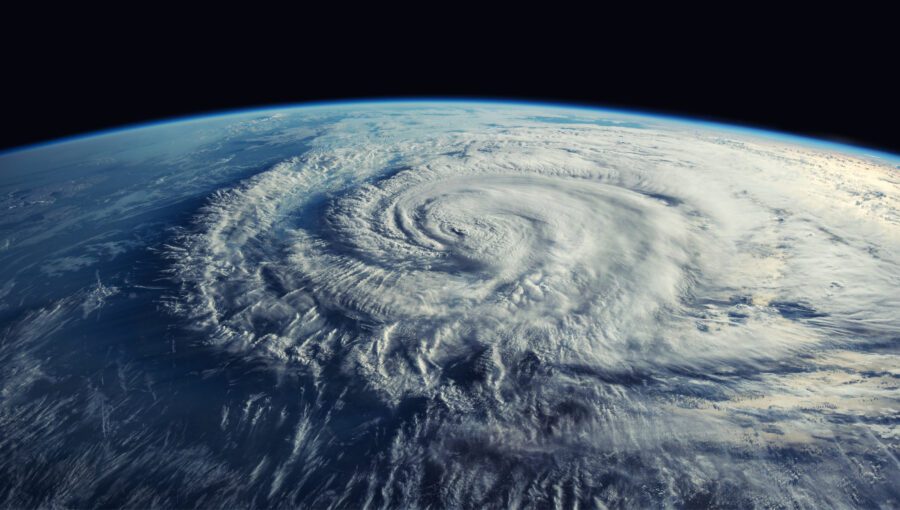
As climate change worsens, severe weather events are becoming increasingly common. Consequently, it’s never been more important to predict and respond effectively to these disasters. That’s often easier said than done, but artificial intelligence (AI) and digital twins are making it easier.
Two recent projects — one from Nvidia called Earth-2 and another from the European Commission named DestinE — have built digital twins of the Earth. While both are still in their early stages, simulations and predictions from these AI-powered models may help researchers improve disaster response and inform broader climate goals.
Building a digital twin of Earth
A digital twin is a virtual recreation of real-world objects, systems or processes. Manufacturers often use them to predict maintenance issues or optimize workflows, but their potential applications span far beyond the production floor. Earth-2 and DestinE use them to model weather patterns.
Unlike conventional weather forecasting, digital twins process a massive amount of data through AI. By analyzing both historical trends and real-time information from monitoring tools across the globe, DestinE and Earth-2 can simulate multiple scenarios to predict likely outcomes. In this case, that means determining what to expect from incoming weather patterns.
Such a massive amount of data and the power of AI lets Earth-2 update forecasts within seconds instead of the hours it takes conventional, CPU-based approaches. Because algorithms are often better at detecting subtle changes in large datasets than humans, digital twins also provide insights that traditional methods may miss.
Conventional weather modeling also typically focuses on precise locations. By contrast, Earth-2 and DestinE replicate the entire globe. While this requires far greater processing power — necessitating AI — it provides a more comprehensive, reliable look at the world’s changing climate.
Short-term benefits of weather digital twins
Building a virtual replica of the planet’s weather systems is more than just a testament to AI’s processing capabilities. Nvidia hopes to use Earth-2 to enable faster, more effective emergency responses to incoming natural disasters.
Taiwan’s Central Weather Administration (CWA) is among the first agencies to deploy Earth-2. The nation has experienced at least 77 typhoons in the last 40 years, and these storms have grown 35% stronger over the same period. By deploying Earth-2, the CWA could learn of typhoons and gauge their strength earlier, letting them prepare accordingly.
The more time officials have to get ready, the better they can stave off the worst effects of extreme weather. That may include facilitating evacuations, erecting flood barriers, or moving the staff and equipment necessary for larger rescue efforts to an affected area before the disaster occurs.
Digital twins can go beyond predicting when storms might happen. DestinE can estimate the size, intensity and duration of flash floods and other extreme weather events before they arrive. This level of detail makes it easier to know which resources to divert toward an area as governments have to deal with multiple emergencies.
Long-term benefits of weather digital twins
Digital twins of the Earth also provide longer-term advantages. Data center operators use the technology to identify inefficiencies through energy simulations, and researchers can perform similar analyses to predict broader climate outcomes.
Climate change is a complex issue, making effective mitigation strategies difficult to verify. DestinE and Earth-2 could make such decision-making easier by simulating how various changes would impact the climate. Because they model the entire Earth, they can replicate how multiple variables influence one another, providing a comprehensive outlook on how scenarios may play out.
Policymakers could simulate changes after enacting a given piece of legislation to see how required changes may improve the world’s climate outlook. Alternatively, researchers could model how growing environments may shift to learn how farms must adapt to feed the population with minimal crop loss despite changing conditions.
While all predictive analytics solutions are prone to some level of inaccuracy, they’re generally more reliable than conventional methods. Digital twins have the added advantage of growing increasingly accurate as they collect additional data, leading to greater confidence down the road.
Remaining challenges
Despite their impressive benefits, AI-powered weather digital twins face some lingering obstacles. Their environmental impact is the most significant. Training a machine learning model can produce hundreds of thousands of kilograms of CO2 emissions thanks to their energy consumption, potentially hindering their sustainability potential.
Nvidia claims Earth-2 is far more energy-efficient than other computing methods, so it doesn’t produce as many emissions as another model of similar size might. DestinE addressed the issue by relying on computers in regions where much of the local electricity comes from renewables. In either case, greater reliance on efficient computing and renewable power could minimize AI’s carbon footprint.
Costs and complexity pose another challenge. Earth-2 and DestinE were both expensive projects, so smaller organizations or governments in less wealthy nations may struggle to achieve similar results. However, the resulting savings from preventing extreme weather-related losses may make up for the initial investment.
AI could lead to a safer, greener future
While many AI applications raise environmental concerns, the technology may also provide climate solutions. Earth-2 and DestinE showcase how predictive analytics can make it easier to respond to immediate weather issues and inform long-term sustainability projects. As the technology matures, digital twins could pave the way for substantial environmental improvements.