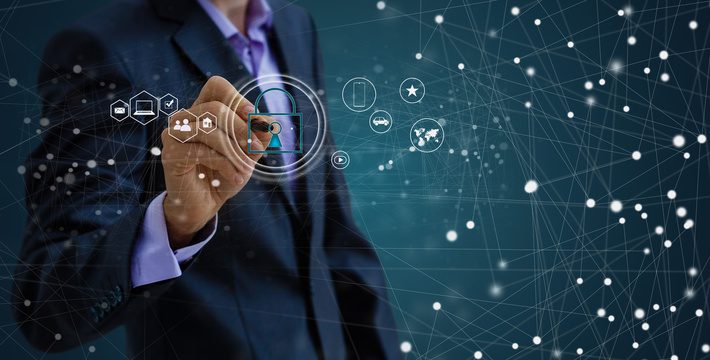
Insurance plays a pivotal role in providing financial security against unforeseen risks and losses. A critical metric for evaluating the financial performance and health of insurance companies is the loss ratio, which measures the ratio of claims paid out to premiums earned. High loss ratios can threaten the profitability and sustainability of insurers. In recent years, data science and artificial intelligence (AI) have emerged as powerful tools to help insurance companies manage and reduce their loss ratios effectively. This article explores how these technologies are being utilized across various aspects of the insurance industry.
Understanding loss ratios in insurance
The loss ratio is a key indicator of an insurance company’s profitability. It is calculated as follows:
A high loss ratio indicates that a large proportion of premium income is being paid out in claims, which can be detrimental to the insurer’s financial stability. Traditionally, insurers have used actuarial analysis and historical data to manage loss ratios, but these methods often lack the predictive power and agility required to respond to emerging risks effectively.
Predictive modeling for risk assessment
Predictive modeling leverages statistical algorithms to forecast future outcomes based on historical data 1. In the insurance sector, predictive models can enhance risk assessment by analyzing numerous factors, such as demographic information, historical claims data, and even social and economic indicators.
Various methods, such as logistic regression, are employed to predict the likelihood of a claim arising from specific variables. Decision trees offer a visual representation of decision rules and their potential consequences, making it easier for insurers to understand the risk landscape. Moreover, ensemble methods like random forests and gradient boosting combine multiple decision trees to improve prediction accuracy 2, enabling insurers to assess risk more effectively and make informed pricing decisions
Case Study: A prominent insurance company implemented a gradient boosting model to identify high-risk policyholders. By incorporating diverse data sources, including social media activity and credit scores, the company was able to tailor premiums more accurately, resulting in a significant reduction in loss ratios 3.
Fraud detection
Fraud detection is essential for mitigating the financial impact of fraudulent claims, which can substantially inflate loss ratios. Insurers often use machine learning algorithms to identify patterns and anomalies within claims data. Techniques such as anomaly detection are employed to spot deviations from normal behavior that may indicate fraudulent activity. Clustering methods group similar claims together, allowing insurers to identify outliers more easily. Advanced approaches, including neural networks, can learn intricate patterns in data, enabling insurers to detect subtle indicators of fraud and take preventive actions before claims are paid.
Case Study: An insurance firm employed anomaly detection algorithms to scrutinize claims data. This approach allowed them to identify and investigate potentially fraudulent claims early, reducing payouts and lowering their overall loss ratio 4.
Telematics and usage-based insurance
Telematics involves the use of devices installed in vehicles to collect data on driving behavior, such as speed, braking patterns, mileage, and time of day. Usage-Based Insurance (UBI) leverages this data to offer premiums based on actual usage and driving habits, promoting safer driving and reducing the likelihood of claims. Some current implementations of telematics run on an insured’s smart phone and do not require a physical connection to the automobile.
This data allows insurers to conduct a more precise risk assessment, tailoring premiums based on actual usage and encouraging safer driving habits. By adjusting premiums according to individual driving behavior, UBI rewards safe drivers with lower rates, ultimately contributing to reduced claims frequency and lower loss ratios.
Case Study: A major auto insurer introduced a UBI program that utilized telematics data to offer personalized premiums. The initiative not only attracted safer drivers but also incentivized policyholders to adopt safer driving habits, and led to a decrease in claims frequency, significantly lowering their loss ratio 5.
Natural Language Processing for claims processing
Natural Language Processing (NLP) enhances claims processing efficiency by automating the extraction of valuable information from unstructured text data, such as accident reports and customer statements. Text mining techniques enable insurers to sift through large volumes of documentation quickly, while sentiment analysis helps gauge customer satisfaction and detect potential issues early in the claims process. This automation speeds up the claims handling time and reduces the chances of human error, leading to quicker settlements and overall operational cost savings.
Large language models (LLMs) are a subset of NLP that allow users to converse with a smart-AI system in text. Insurers can use these system to produce smart chat-bots that help customers submit claims or answer specific questions. Furthermore, insurers can build internal LLMs that intake proprietary documents during a process called training. These internal LLMs can then be used to help agents, underwriters, and support staff query the LLM to ask questions about complex rules and internal policies.
Case Study: Insurers use NLP to automate the initial assessment of claims by extracting key information from accident reports and customer statements. This speeds up the claims process and reduces the likelihood of errors, leading to faster settlements and lower operational costs 6.
Customer segmentation and personalization
Customer segmentation is critical in modern insurance practices, where insurers leverage clustering algorithms to divide customers into groups based on shared characteristics. This segmentation allows for tailored risk management strategies and personalized pricing approaches. Clustering algorithms group customers based on behavior, demographics, and claims history. By identifying low-risk customers and offering them customized insurance products, insurers can attract more desirable policyholders, which ultimately contributes to a reduction in overall loss ratios.
Case Study: An insurer used clustering algorithms to segment their customer base. By offering personalized discounts and coverage options to low-risk customers, they attracted more desirable policyholders and reduced their overall loss ratio 7.
A warning on regulation and the use of personally identifiable information
The use of data and AI can lower loss ratios, but are not without their own unique set of potential issues. Tech-driven insurance companies face risks in balancing innovation with ethical considerations and regulatory compliance in an evolving digital landscape.
One insurance company, known for its innovative approach to insurance, utilized video analysis as a part of its claims process to expedite and enhance the accuracy of claims assessments 8. By integrating technology, the company allowed policyholders to submit videos of incidents, such as property damage or accidents, which could then be analyzed using AI algorithms 9. This method not only speeds up the claims process but also helps in minimizing fraudulent claims. The incorporation of video evidence allowed the company to streamline operations, making the insurance experience more efficient and user-friendly.
However, this company faced criticism and legal scrutiny over its use of video analysis in claims processing. Concerns arose regarding privacy and consent, as many users felt uneasy about how their video submissions were being used and stored 10. In some instances, customers were reportedly unaware that their videos would be analyzed for patterns or trends, raising ethical questions about data usage and consumer transparency 11. These concerns culminated in complaints and allegations that the company’s practices might violate privacy laws 12, leading to investigations, court cases 13, and discussions about the need for stricter regulations.
Conclusion
Data science and AI are revolutionizing the insurance industry, providing powerful tools to reduce loss ratios and improve profitability. By leveraging predictive modeling, fraud detection, telematics, NLP, and customer segmentation, insurers can more accurately assess risk, streamline operations, and enhance customer satisfaction. The future of insurance lies in the continued integration of these advanced technologies, promising even greater efficiencies and cost savings.
References
- King, M.R.N., Timms, P.D., Rubin, T.H. (2021). Use of Big Data in Insurance. In: Rau, R., Wardrop, R., Zingales, L. (eds) The Palgrave Handbook of Technological Finance. Palgrave Macmillan, Cham.↩︎
- Henckaerts, R., Côté, M.P., Antonio, K., Verbelen, R. Boosting Insights in Insurance Tariff Plans with Tree-Based Machine Learning Methods. North American Actuarial Journal.↩︎
- Sahai, R. et al. (2023). Insurance Risk Prediction Using Machine Learning. In: Wah, Y.B., Berry, M.W., Mohamed, A., Al-Jumeily, D. (eds) Data Science and Emerging Technologies. DaSET 2022. Lecture Notes on Data Engineering and Communications Technologies, vol 165. Springer, Singapore.↩︎
- Albrecher, H., Bommier, A., Filipović, D. et al. (2019) Insurance: Models, Digitalization, and Data Science. European Actuarial Journal 9, 349–360.↩︎
- Guillen M., J.P. Nielsen, M. Ayuso, A.M. Pérez-Marín, (2018), The Use of Telematics Devices to Improve Automobile Insurance Rates. Risk Analysis.↩︎
- Shaw, Jack, Insurance Thought Leadership, Using NLP to Detect Fraud in Insurance Claims, February 4, 2024.↩︎
- Hu, Sen, Adrian O’Hagan, The Actuary, Insurance Claims Forecasting with Cluster Analysis, July 8, 2020.↩︎
- Wininger, Shai, Lemonade Blog, The Sixth Sense, 2019.↩︎
- Kabir, Usman, Yahoo Finance, Lemonade, Inc. Revolutionizes Insurance with AI-Powered Claims Processing, Fraud Detection, and Personalized Pricing, October 5, 2024.↩︎
- Morrison, Sara, Vox, A Disturbing Viral Twitter Thread Reveals How AI-powered Insurance Can Go Wrong, May 27, 2021.↩︎
- Greig, Jonathan, ZDNet, Lemonade Insurance Faces Backlash for Claiming AI System Could Automatically Deny Claims, May 27, 2021.↩︎
- Quach, Katyanna, The Register, Insurance Startup Backtracks on Running Videos Of Claimants Through Ai Lie Detector, May 26, 2021.↩︎
- Adriano, Lyle, Insurance Business Magazine, Lemonade Faces Class Action Lawsuit Over Alleged Mishandling Of Customers Biometric Data, Aug 30, 2021.↩︎
Author
J. Joseph Rusnak received his Ph.D. and S.M degrees from Harvard University and his M.Eng. and S.B. degrees from the Massachusetts Institute of Technology.
The application of data science and AI algorithms to reduce insurance loss ratios is a game-changer for the industry! Leveraging advanced analytics can significantly improve risk assessment and claims management, leading to more accurate pricing and reduced fraud. It’s exciting to see how technology is transforming traditional insurance practices into more efficient and data-driven processes. This shift not only benefits companies but also enhances customer experience by enabling more personalized services!
Brandon,
Thank you for your insightful comment! I completely agree that the integration of data science and AI is revolutionizing the insurance industry. It’s exciting to see how these advancements enhance risk assessment and improve customer experiences through personalized services. Thank you for sharing your thoughts.
Joe