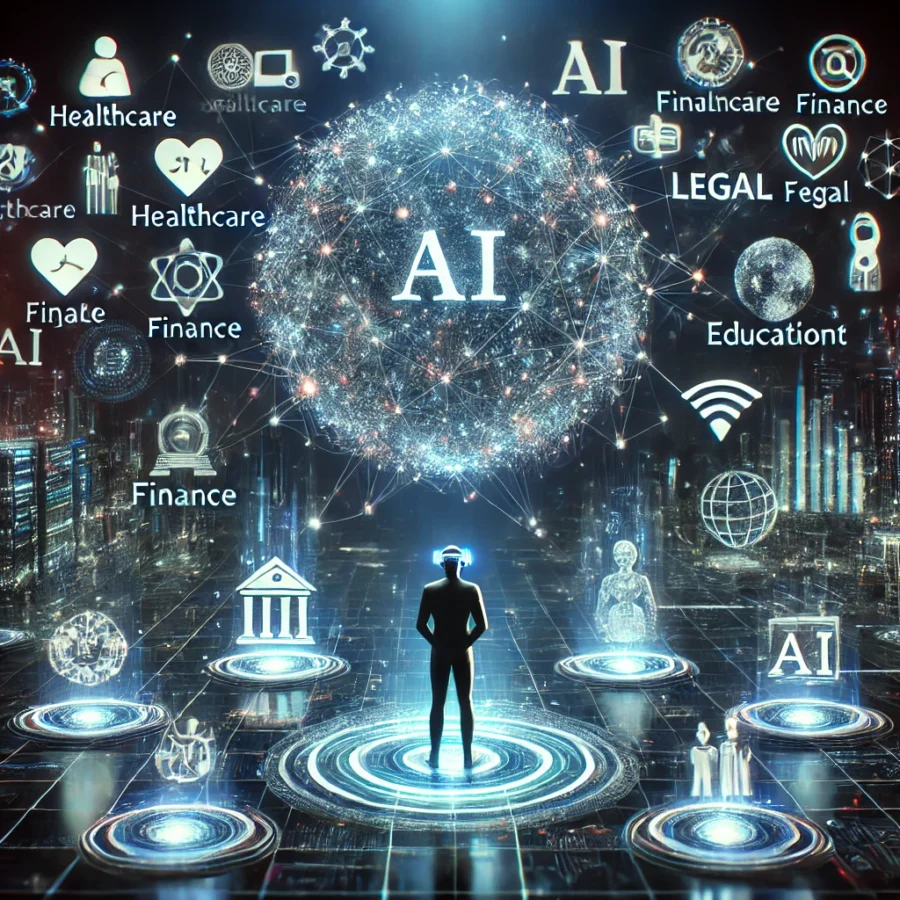
The AI market is demonstrating sustained growth, expanding at a compound annual growth rate of 38.1% from 2022 to 2030. From voice assistants to autonomous vehicles, we have witnessed that AI technologies have become increasingly prevalent.
However, as AI systems grow more complex, the industry faces a significant challenge: the opacity of decision-making processes in many advanced AI models, often called “Black Box” models.
This article explores Explainable AI (XAI) ‘s role in bridging the gap between complex AI systems and human understanding. We aim to examine the characteristics of Black Box models, their challenges, and the various approaches being developed to make AI more transparent and interpretable.
Black Box Models: Explained
Black Box models are AI systems whose internal workings are not easily understood or interpreted by humans. Often based on deep learning and neural networks, these models process vast amounts of data to make predictions or decisions. While they can achieve impressive accuracy, their complexity makes it difficult to discern how they arrive at their conclusions.
Challenges associated with Black Box models
The opacity of Black Box models presents several challenges:
- Trust issues: Users and stakeholders may hesitate to rely on systems they need help understanding.
- Regulatory concerns: Many industries require explainable decision-making processes, which Black Box models struggle to provide.
- Bias detection: Identifying and correcting biases in the model becomes easier with transparency.
Given these difficulties, there has never been a more pressing need for interpretable AI systems. Explainable AI (XAI) addresses the need for transparency and confidence in AI decision-making.
Explainable AI: The purpose
XAI aims to create AI systems that provide clear, understandable explanations for their decisions and actions.
XAI methodologies focus on developing techniques that make AI models more transparent and interpretable without sacrificing performance.
As XAI develops, it has the potential to impact a wide range of sectors, including public policy, industry, education, legal, and retail, where openness is crucial to establishing responsibility and confidence in AI-driven judgments. These industries gain by explaining things in a way that makes sense to stakeholders, helping them comprehend AI predictions and make wise decisions.
Real-world applications and wider effects of XAI
Explainable AI (XAI) is revolutionizing the trust and utilization of AI systems by finding applications in various industries. By making complicated AI models interpretable, XAI assists multiple industries in addressing accountability, transparency, and bias issues. Furthermore, as XAI develops further, its ramifications become more widespread throughout businesses since it becomes essential for fostering ethical AI practices and establishing confidence.
Healthcare:
- Present Use: XAI has been used to promote transparency in AI-driven medical judgments by offering justifications for diagnostic suggestions. For instance, XAI is utilized in individualized treatment plans to support cancer treatment recommendations and in medical imaging to clarify how AI finds abnormalities in scans. This makes it easier for doctors and patients to understand and accept the system’s recommendations.
- Broader Impact: As XAI is employed more frequently, medical professionals and AI systems are growing more confident in one another. Long-term implications of XAI in healthcare are expected to improve patient outcomes and decision-making by increasing the overall accountability of AI diagnoses.
Finance:
- Present Use: XAI methods like SHAP and LIME have helped explain intricate credit scoring models in the financial sector, boosting the equity and transparency of lending procedures.
- Broader Impact: XAI’s broader impact in finance is in the public’s trust and regulatory compliance. By improving the interpretability of AI-driven credit decisions, financial organizations can enhance client confidence in automated financial systems and comply with regulatory obligations.
Legal sector:
- Present Use: XAI enhances the openness of AI-driven court decisions, including predictive policing and criminal sentences. By giving transparent explanations of the decision-making process, XAI helps assure fairness and reduce bias.
- Broader Impact: XAI’s promise to create more egalitarian legal systems has a wider impact on the legal industry. AI’s capacity to explain rulings will increase confidence in legal systems as they become more frequently used tools, guaranteeing open and accountable justice.
Education:
- Present Use: XAI makes course recommendations and student success projections more understandable for AI technologies that tailor learning experiences.
- Broader Impact: XAI can build more individualized and efficient educational systems by making AI tools more transparent. Long-term effects will increase trust among students, teachers, and AI-powered learning systems, encouraging more expansive use of AI in the classroom.
Manufacturing and supply chain:
- Present Use: XAI is being utilized in manufacturing to improve the interpretability of predictive maintenance systems, enabling engineers to understand better why specific equipment is expected to fail. Similarly, XAI enhances transparency in AI-driven logistics and inventory choices in supply chain optimization.
- Broader Impact: XAI will eventually lead to increased operational efficiency, decreased downtime, and greater confidence in AI-driven systems as it is more thoroughly incorporated into supply chains and manufacturing processes. Additionally, transparency will facilitate greater cooperation between AI systems and human operators.
Retail:
- Present Use: XAI’s capacity to provide precise product recommendations is advantageous to retailers like Amazon and Netflix, which use AI-based recommendation systems. XAI enhances consumer happiness and maximizes marketing tactics by giving context for particular goods or information recommendations.
- Broader Impact: XAI can increase trust in AI-driven retail strategies by making recommendations more transparent. This transparency fosters better customer interactions and enables firms to make data-driven decisions that are simpler to defend and adjust.
Government and public policy:
- Present Use: Using XAI, governments can guarantee openness to resource distribution and AI-driven public policy formulation. XAI gives the justifications required for AI models employed in resource allocation or public policy decision-making.
- Broader Impact: XAI’s wider impact on government includes its potential to produce more responsible and transparent public services. Governments may assure fairness in using AI for social governance and create public trust by explaining AI-driven judgments.
Even though XAI is revolutionizing businesses and building trust, the efficacy of the underlying methods used to interpret AI models determines XAI’s success.
Current approaches to XAI
XAI techniques can be broadly classified into two categories:
- Model-agnostic techniques: The application of these methods extends to all AI models, irrespective of their internal architecture.
- Model-specific techniques: Tailored to specific types of AI models, these explanations offer detailed insights, enhancing understanding and performance.
Popular XAI methods include LIME (Local Interpretable Model-agnostic Explanations) and SHAP (SHapley Additive exPlanations), which help interpret individual predictions made by complex models.
Current XAI techniques’ limitations
While current XAI approaches have made significant strides, they face limitations:
- Trade-offs between accuracy and interpretability: Pursuing simpler, easier-to-understand models may lead to sacrificing predictive potential. Neural networks, for instance, have the potential to provide highly accurate medical diagnoses. Still, their complexity makes them difficult to understand, restricting their use in delicate fields like healthcare, where openness is essential.
- Universality challenges: It isn’t easy to provide explanations generally understood by different user groups and areas. For example, significant differences exist in the explanation requirements for financial credit scoring algorithms and judicial determinations.
Even though these restrictions pose serious difficulties, they are spurring creativity in how XAI and human cognitive processes interact to improve the interpretability of AI systems.
How to minimize the gap between AI and human understanding?
To truly bridge the gap between AI and human understanding, XAI must align with human cognitive processes. This involves:
- Developing intuitive visualizations and interfaces
- Providing context-aware explanations
- Adapting explanations to different user expertise levels
Communication guidelines for XAI
Effective communication of AI decisions to non-expert audiences is crucial. This includes:
- Using clear, jargon-free language
- Providing multi-modal explanations (e.g., text, visuals, interactive elements)
- Offering varying levels of detail based on user needs
Where is eXplainable AI (XAI) headed?
Emerging trends in XAI research include:
- Integration of cognitive sciences with AI to create more human-like explanations
- Development of dynamic explanation systems that adapt to user feedback
- Exploration of causal AI models for enhanced explainability
- Collaborative Intelligence: Human-AI Synergy
The potential of AI extends beyond autonomous systems to collaborative intelligence, wherein AI augments human capabilities. XAI facilitates this collaboration by enabling effective communication between humans and AI systems.
Ethical considerations and governance
Addressing ethical implications becomes paramount as AI systems become more prevalent and influential. XAI can contribute to responsible AI practices by:
- Facilitating audits of AI systems for bias and fairness
- Enabling compliance with regulations requiring transparency in decision-making
- Make users capable enough to understand and challenge AI-driven decisions affecting them
Bottom line
We are progressing towards a future where openness and collaboration underpin an AI ecosystem, unlocking its potential across all industries while upholding ethical standards and ensuring human oversight. Explainable AI (XAI) revolutionizes legal systems, education, retail, government, finance, and healthcare. XAI advances fairness, trust, and moral AI practices by making complicated AI models interpretable and transparent.
Adopting and developing XAI approaches is essential for maintaining openness, credibility, and moral accountability as AI systems become increasingly integrated into business decision-making processes. To ensure that AI stays a tool that improves society, organizations need to give XAI top priority to bridge the knowledge gap between AI complexity and human comprehension.
The future of AI is in its capacity to collaborate with people in valuable and intelligible ways; XAI is the means of closing this divide between AI’s complexity and human trust.
Now is the time to embrace and prioritize XAI. Organizations should ensure that AI remains a tool that improves society and fosters trust by including transparent AI models, abiding by legal requirements, and training stakeholders on AI-driven decision-making processes.