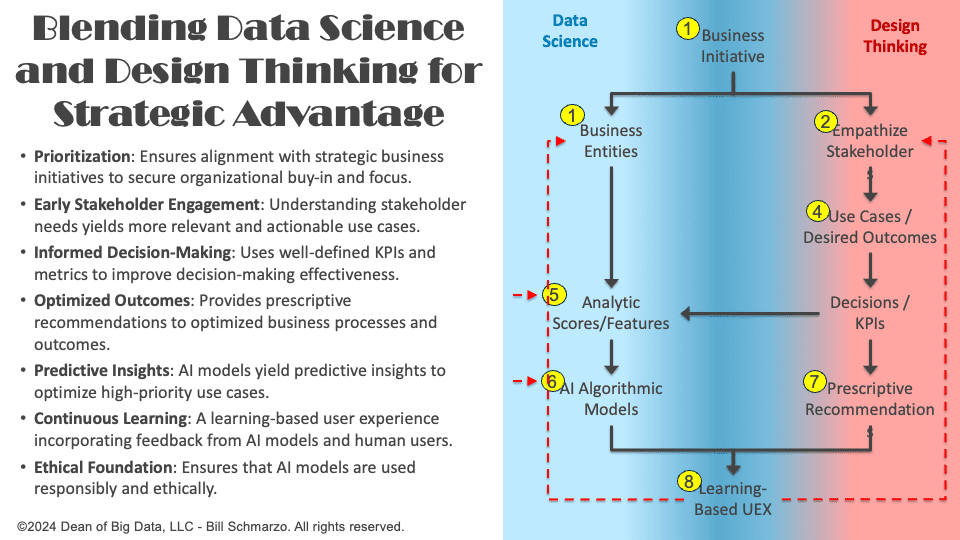
Leveraging AI and data effectively has become crucial to organizational success in a rapidly evolving digital landscape. The “Thinking Like a Data Scientist” (TLADS) methodology, detailed in the second edition of my book “The Art of Thinking Like a Data Scientist,” offers a comprehensive framework for integrating data science, design thinking, and economics to achieve meaningful, responsible, and ethical business outcomes.
I recently created a video highlighting how the steps and concepts that comprise the TLADS methodology interplay to help organizations leverage AI with their data to deliver more relevant, meaningful, responsible, and ethical outcomes. Here are the highlights of that video (see the video Thinking Like a Data Scientist 2nd Edition eBook Explanation).
Step 1: The Foundation: Business Initiatives and Desired Outcomes
Step 1 begins with identifying a critical business initiative that is highly important to the organization and must be addressed within a 12 to 16-month timeframe. This urgency ensures organizational focus and senior management buy-in. Examples of business initiatives include improving customer retention, enhancing on-time product delivery, increasing patient treatment effectiveness, or reducing inventory costs.
To fully understand the business initiative, it is essential to ask fundamental questions:
- What are the desired outcomes of this initiative?
- What are the business and operational benefits?
- What are the potential impediments?
- What are the ramifications of failure?
- What are the potential unintended consequences of success?
These questions help to build a robust set of Key Performance Indicators (KPIs) and metrics that will eventually be integrated into the AI utility function that guides the operations and performance of our AI models.
Step 2: Empathize and Understand Stakeholders
Step 2 involves empathizing with internal and external stakeholders impacted by the business initiative. Understanding their perspectives, desired outcomes, potential impediments, and the KPIs they value is crucial. Engaging stakeholders early ensures their buy-in and helps uncover valuable organizational knowledge (tribal knowledge) that can drive better decision-making.
Stakeholder involvement is also critical for adoption. By being involved in the definition and design phase, stakeholders are more likely to adopt the analytics results, leading to improved decision-making and achieving the business initiative.
Step 3: Model Key Business Entities
Parallel to stakeholder engagement, in Step 3, the data science track begins by modeling key business entities, such as humans (e.g., teachers, nurses, doctors) or devices (e.g., compressors, chillers, trains). This phase involves creating detailed analytic profiles that capture each entity’s predicted behavioral and performance propensities, akin to developing digital twins for these entities. By leveraging the concept of nanoeconomics, organizations can gain deep insights into the specific behaviors and interactions of individual entities, which is crucial for making informed, data-driven decisions later in the process.
The analytic profiles enable the identification of patterns and anomalies that might not be apparent at an aggregate level. By examining individual behaviors and performance metrics, organizations can tailor their strategies to optimize efficiency, mitigate risks, and enhance overall performance. Such detailed modeling forms the backbone of predictive analytics, providing a foundation for subsequent phases of the data science methodology (Figure 1).
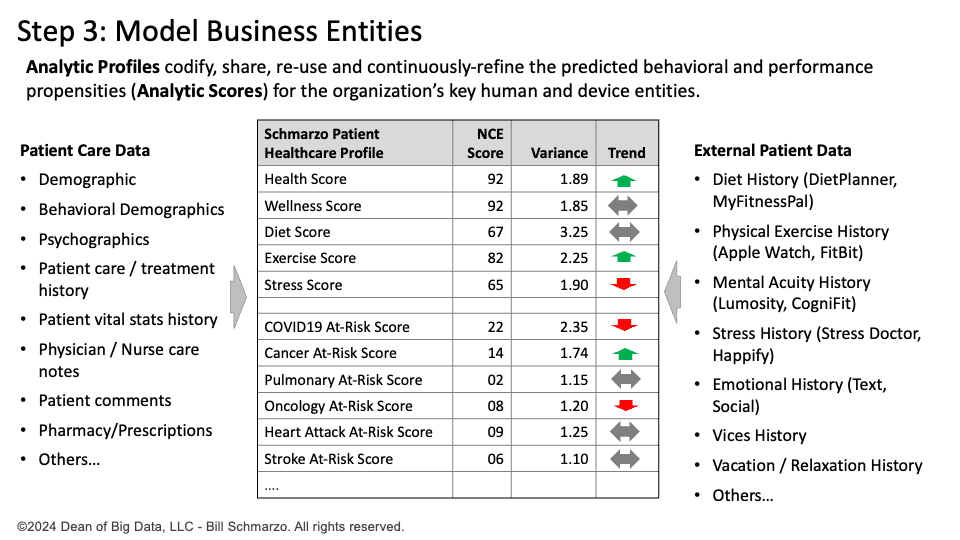
Figure 1: Step 3: Model Business Entities (Data Science)
Step 4: Identify and Prioritize Use Cases
A use case is a set of actions (narrative, blueprint, or workflow) outlining a business or operational scenario—framed by desired outcomes, critical decisions, and KPIs and metrics—against which to optimize the targeted business initiative.
Once the desired outcomes are understood, Step 4 identifies and clusters actionable use cases around these outcomes. Use cases are specific actions that can be taken to achieve the business initiative, often starting with verbs like optimize, increase, reduce, or improve.
Typically, a business initiative will generate multiple use cases, but it is important to prioritize them based on value and feasibility. This prioritization helps to create a roadmap that allows for learning and improvement from one use case to the next, leveraging the economies of learning to accelerate value and reduce risk (Figure 2).
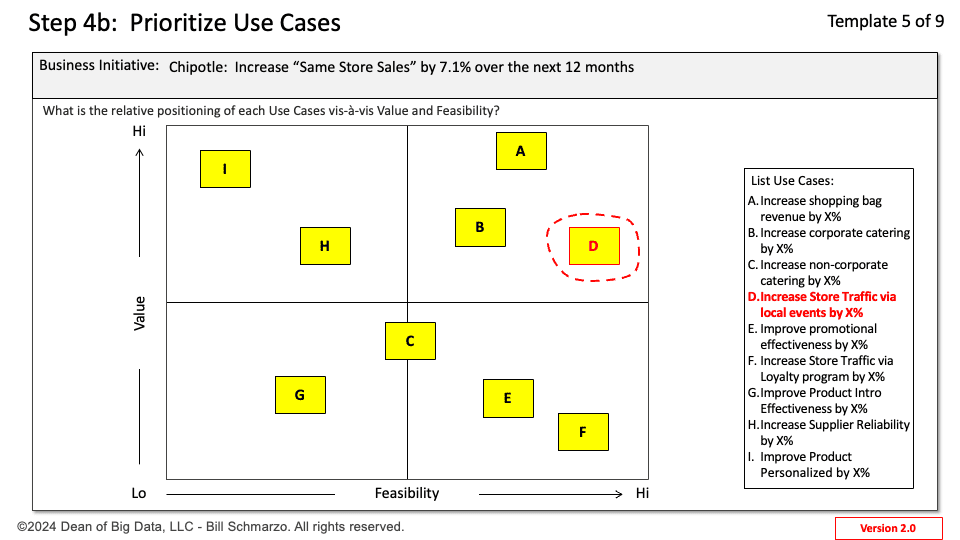
Figure 2: Identify and Prioritize Use Cases
Step 5: Identify Analytic Scores and ML Features
The next step involves identifying the analytic scores and machine learning features supporting decision-making.
- An Analytic Score quantifies a business entity’s behavior or performance propensity based on critical variables and metrics.
- A Machine Learning (ML) Feature is an individual measurable property or characteristic used as input to a predictive model to help forecast outcomes.
This requires defining the key variables and metrics that will be used to evaluate performance. By scoring these variables, organizations can create detailed analytic profiles that provide insights into the behaviors and propensities of the business entities.
Based on these scores, machine learning features are developed, enabling the creation of predictive models. These models help forecast outcomes and inform decisions, ensuring the organization can respond proactively to changes and opportunities. This step is crucial for building robust, effective AI models that drive actionable insights.
Step 6: Develop and Deploy AI Models
Our prioritized use cases focus on identifying the decisions these use cases need to empower, the impacted stakeholders, and the KPIs and metrics to measure decision effectiveness. This information feeds into the data science track, where analytic scores and machine learning features are developed to aid decision-making.
Different AI capabilities, including generative, causal, analytical, and autonomous AI, are employed to build models that drive predictive recommendations.
- Generative AI builds upon patterns found in text and other structured data sets to generate new content, offering innovative ways to solve problems or create products.
- Analytical AI analyzes existing data to uncover patterns and trends, making predictions and recommendations.
- Causal AI uncovers and quantifies cause-and-effect relationships to provide insights into the underlying reasons behind observed patterns and phenomena.
- Autonomous AI focuses on independent operation and continuous learning to make real-time decisions with minimal human intervention.
These recommendations are linked to the stakeholders’ decisions, ensuring a cohesive and effective strategy.
Step 7: Map Prescription Recommendations to Decisions
With the analytic scores and ML features in place, the focus shifts to mapping these prescriptive recommendations to specific business decisions. This involves linking the output of AI models to the decisions that stakeholders need to make. By doing so, organizations can ensure that the insights generated by the models are directly applicable to real-world scenarios.
This mapping process requires close collaboration with stakeholders to understand their decision-making processes and the key metrics they rely on. Organizations can enhance decision accuracy and effectiveness by integrating prescriptive recommendations into these processes, ultimately achieving the desired business outcomes.
Step 8: Create a Learning-Based User Experience
The final step in the methodology is to create a learning-based user experience. This involves algorithmic and human learning processes to continuously improve the effectiveness of AI models, better understand business entities, and gain more insights into the needs of critical stakeholders.
Algorithmic learning captures success and failure data to refine models, while human learning collects user feedback to understand what’s working and why. This feedback loop, akin to reinforcement learning with human feedback, ensures that the system continuously evolves and improves (Figure 3).
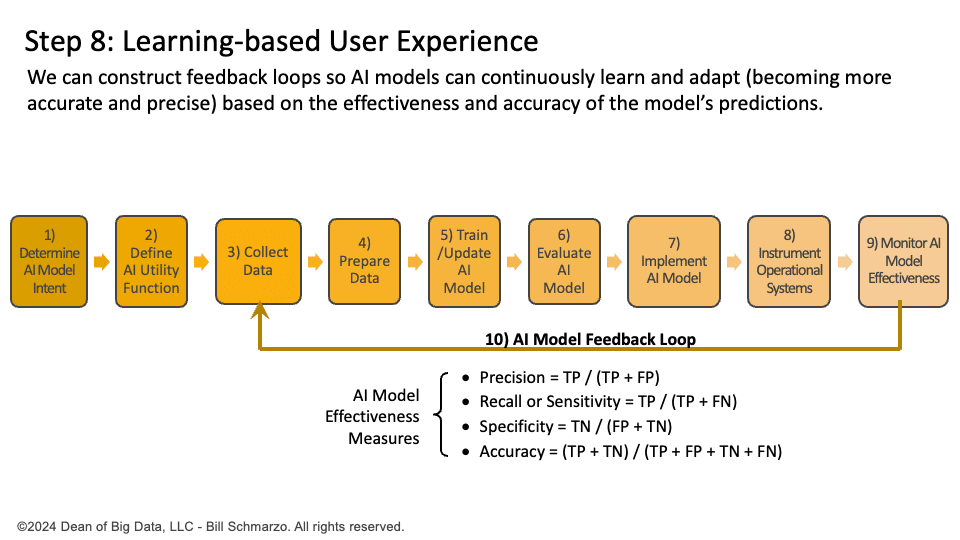
Figure 3: Step 8: Create a Learning-based UEX
Conclusion: A Passion for Responsible AI
In summary, the “Thinking Like a Data Scientist” methodology offers a powerful blueprint for organizations seeking to harness the full potential of AI and data analytics. By focusing on critical business initiatives, engaging stakeholders, modeling key entities, assessing and prioritizing use cases, developing robust AI models, and fostering a continuous learning environment, businesses can thrive in leveraging AI and its wealth of data to deliver more relevant, meaningful, responsible, and ethical outcomes.