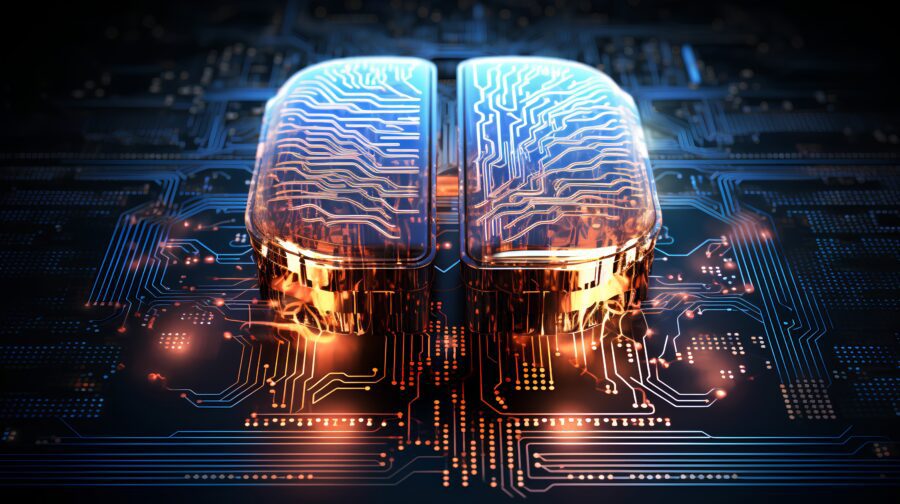
The basis of how the human brain works is conceptually the mechanism of the mind—which is the electrical and chemical signals of neurons, in sets, with their interactions and features.
Recently, the Department of Commerce released a Strategic Vision on AI Safety, stating that, “The U.S. AI Safety Institute will focus on three key goals: Advance the science of AI safety; Articulate, demonstrate, and disseminate the practices of AI safety; and Support institutions, communities, and coordination around AI safety.”
In a publication on AI interpretability, Mapping the Mind of a Large Language Model, Anthropic wrote, “We successfully extracted millions of features from the middle layer of Claude 3.0 Sonnet, (a member of our current, state-of-the-art model family, currently available on claude.ai), providing a rough conceptual map of its internal states halfway through its computation. This is the first ever detailed look inside a modern, production-grade large language model. For example, amplifying the “Golden Gate Bridge” feature gave Claude an identity crisis even Hitchcock couldn’t have imagined: when asked “What is your physical form?”, Claude’s usual kind of answer is – “I have no physical form, I am an AI model” – changed to something much odder: “I am the Golden Gate Bridge… my physical form is the iconic bridge itself…”. Altering the feature had made Claude effectively obsessed with the bridge, bringing it up in answer to almost any query—even in situations where it wasn’t at all relevant. “
What should be the basis—or neuroscience—of AI safety? The brain or the mind?
Artificial neural networks are digital simulations of biological neural networks. However, with several neuroimaging techniques, fMRI, EEG, electron microscopes, CT, PET, and others, the brain is yet to be fully understood for numerous mental states.
Although the anatomy of neurons is delineated with correlations to physiology, neurons—conceptually—are not the human mind. It is theorized that the human mind has functions and features. Functions arise from interactions of electrical and chemical signals, in sets. They include memory, feelings, emotions, and modulation of internal senses. Features qualify or grade the functions. Simply, features place what functions do in any instance. They include attention, awareness [or less than attention], self or subjectivity, and intent or free will. Sets of electrical and chemical signals are conceptually obtained in clusters of neurons—across the central and peripheral nervous systems.
Though Anthropic labeled features as “matching patterns of neuron activations, to human-interpretable concepts”, in the human mind, features, and functions are not the same.
In the human mind, the Golden Gate Bridge is a memory, which can be qualified by attention, awareness, self or intent. Anthropic noted that “Looking near a “Golden Gate Bridge” feature, we found features for Alcatraz Island, Ghirardelli Square, the Golden State Warriors, California Governor Gavin Newsom, the 1906 earthquake, and the San Francisco-set Alfred Hitchcock film Vertigo. Looking near a feature related to the concept of “inner conflict”, we find features related to relationship breakups, conflicting allegiances, and logical inconsistencies, as well as the phrase “catch-22” This shows that the internal organization of concepts in the AI model corresponds, at least somewhat, to our human notions of similarity. “
In the human mind, conceptually, there are thick sets and thin sets. Thick sets [of electrical and chemical signals] collect whatever is similar between information, leaving thin sets with whatever is unique. This means that several interpretations in the human mind are possible by thick sets of signals—doors, windows, chairs, desks and others.
Thick and thin sets are also qualifiers [or features] on the mind. Thick sets broadly explain what is referred to as associative memory, concepts, and categories. There are qualifiers on the mind like sequences, which can be old or new, principal spots, splits, and others.
Anthropic wrote, “The features we found represent a small subset of all the concepts learned by the model during training, and finding a full set of features using our current techniques would be cost-prohibitive (the computation required by our current approach would vastly exceed the compute used to train the model in the first place). Understanding the representations the model uses doesn’t tell us how it uses them; even though we have the features, we still need to find the circuits they are involved in. And we need to show that the safety-relevant features we have begun to find can actually be used to improve safety.”
AI does not have emotions or feelings, but it has memory—or it uses digital memory. All the memory that is available to AI is never brought to attention at once, just like the human memory, showing that the memory gets qualified or graded. Some of the qualifiers of human memory are similar to those used by large language models, though they might have additional variations.
The goal is to seek out what qualifiers work for LLMs, and to explain how they come by their outputs, either positive or not. It is possible to draw from the qualifiers of the human mind, for how order is established and what to seek.
When Anthropic tweaked a feature, it answered, “I am the Golden Gate Bridge… my physical form is the iconic bridge itself…”.
Something like this is possible in the human mind—with neural probes, certain mental conditions and some psychoactive substances. If it were the mind, it would be a problem of distribution [a qualifier], where, rather than discuss the bridge as something else in the memory, it instead personalized it, since there was a cut from that distribution of being [for the awareness of self and things]. So, the memory was not used as something the self knows, it made it what the self is.
Guardrails are already shaping what some AI can output—or not, but even the mechanics of guardrails can be defined by the human mind, like what not to give attention to, what to be aware of, and what to use its sub-intent to evade.
How the electrical and chemical signals of neurons interact is postulated by the action potentials—neurotransmitters theory of consciousness.
The qualifiers of the human memory can then be used to seek out explainable artificial intelligence, for what to look for, not just to find “a full set of features using their current techniques.”