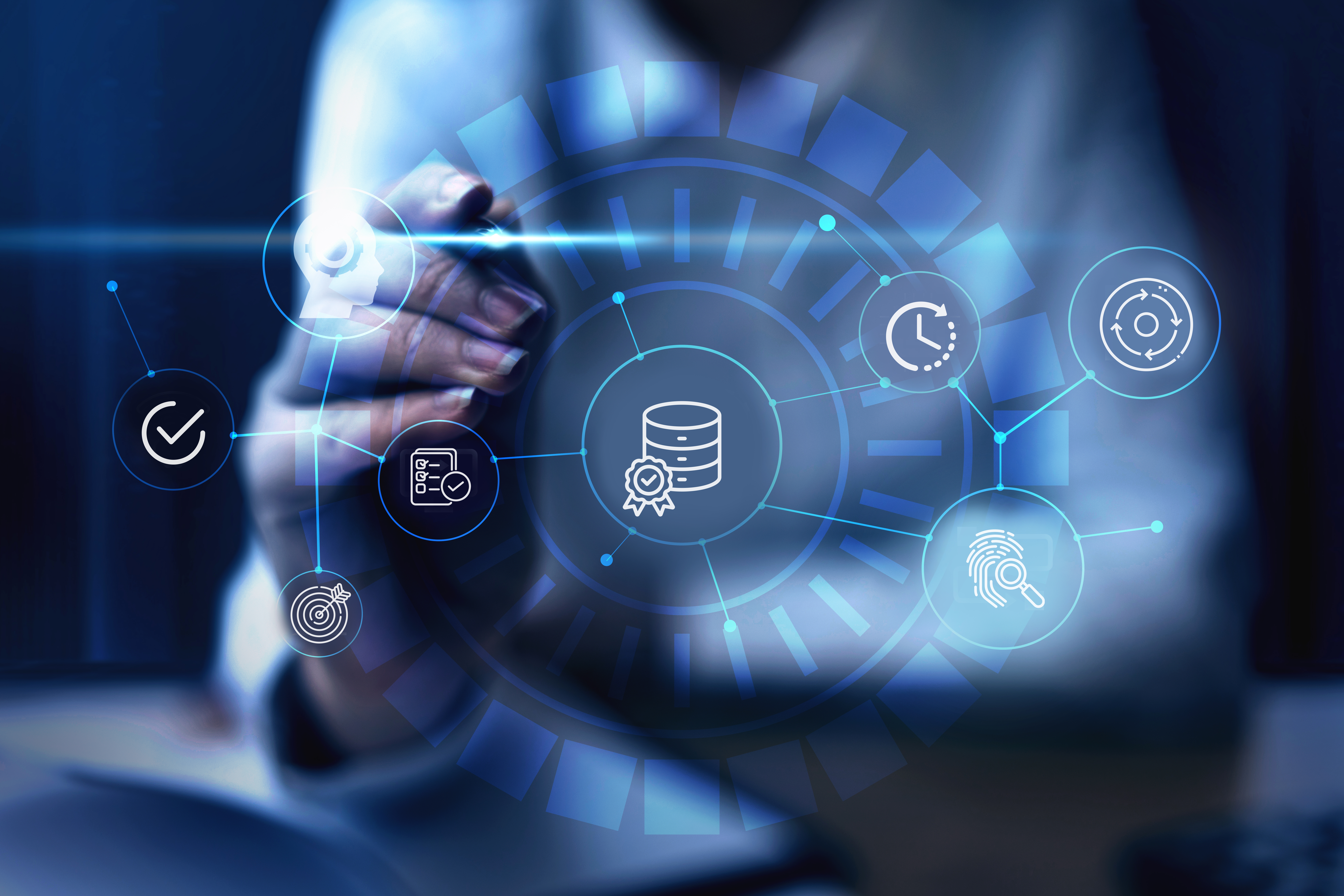
The need for high-quality, trustworthy data in our world will never go away.
Treating data quality as a technical problem and not a business problem may have been the biggest limiting factor in making progress. Finding technical defects, such as duplicate data, missing values, out-of-order sequences, and drift from expected patterns of historical data are no doubt critical, but this is just the first step. A more demanding and crucial step is to measure the business quality which checks to see if the data is contextually correct.
Let us see the pillars of Modern Data Quality:
1. Top-down Business KPI – Perhaps the IT teams would have benefited if the term data quality had never been coined, and instead “business quality” was the goal. In that case, the raison d’être of ensuring data is correct would have been to ensure the business outcomes were being met. In this scenario, the focus shifts from the data’s infrastructure to its context.
But what exactly is “context?”
It is the application of business use to the data. For example, the definition of a “customer” can vary between different business units. For sales, it is the buyer, for marketing, it is the influencer, and for finance, it is the person who pays the bills. So, the context changes depending on who is dealing with the data. Data Quality needs to keep in lockstep with the context. In another example, country code 1 and region US and Canada may appear to be analogous, but they are not. Different teams can use for vastly different purposes the same columns in a table. As a result, the definition of data quality varies. Hence, data quality needs to be applied at the business context level.
2. Product Thinking – The concepts evoked by the data mesh principles are compelling. They evolve our thinking so that older approaches that might not have worked in practice actually can work today. The biggest change is how we think about data: as a product that must be managed with users and their desired outcomes in mind.
Organizations are applying product management practices to make their data assets consumable. The goal of a “data product” is to encourage higher utilization of “trusted data” by making its consumption and analysis easier by a diverse set of consumers. This in turn increases an organization’s ability to rapidly extract intelligence and insights from their data assets in a low-friction manner.
Similarly, data quality should also be approached with the same product management discipline. Data producers should publish a “data contract” listing the level of data quality promised to the consumers. By treating data quality as a first-class citizen, the producers should learn how the data is being used and the implications of its quality. Data products’ data quality SLA is designed to ensure that consumers have knowledge about parameters like the freshness of data.
3. Data Observability – Frequently, the data consumer is the first person to detect anomalies, such as the CFO discovering errors on a dashboard. At this point, all hell breaks loose, and the IT team goes into a reactive fire-fighting mode trying to detect where in the complex architecture the error manifested.
Data Observability fills the gap by constantly monitoring data pipelines and using advanced ML techniques to quickly identify anomalies, or even proactively predict them so that issues can be remediated before they reach downstream systems.
Data quality issues can happen at any place in the pipeline. However, if the problem is caught sooner, then the cost to remediate is lower. Hence, adopt the philosophy of ‘shift left.’ A data observability product augments data quality through:
- Data discovery extracts metadata from data sources and all the components of the data pipeline such as transformation engines and reports or dashboards.
- Monitoring and profiling – for data in motion and at rest. What about data in use?
- Predictive anomaly detection – uses built-in.
- Alerting and notification
Data quality is a foundational part of data observability. The figure below shows the overall scope of data observability.
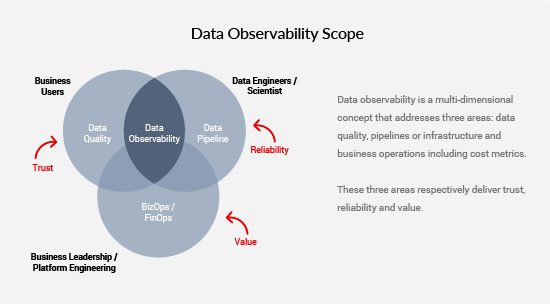
4. Overall Data Governance – The data quality subsystem is inextricably linked to overall metadata management.
On one hand, the data catalog stores defined or inferred rules, and, on the other hand, DataOps practices generate metadata that further refines the data quality rules. Data quality and DataOps ensure that the data pipelines are continuously tested with the right rules and context in an automated manner and alerts are raised when anomalies are inferred.
In fact, data quality and DataOps are just two of the many use cases of metadata. Modern data quality is integrated with these other use cases as the figure below shows.
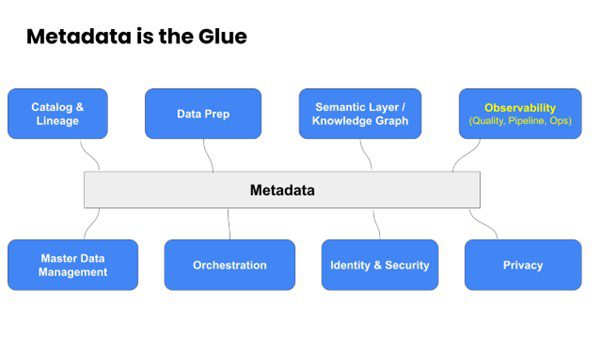
A comprehensive metadata platform that coalesces data quality within other aspects of data governance improves the collaboration between the business users, such as data consumers and the producers and maintainers of data products. They share the same context and metrics.
This tight integration helps in adopting the shift left approach to data quality. Continuous testing, orchestration and automation help reduce error rates and speed up delivery of data products. This approach is needed to improve trust and confidence in the data teams.
This integration is the stepping stone for enterprise adoption of modern data delivery approaches of data products, data mesh, and data sharing options, like exchanges and marketplaces.
Contact us to know more about Modern Data Quality Platform.