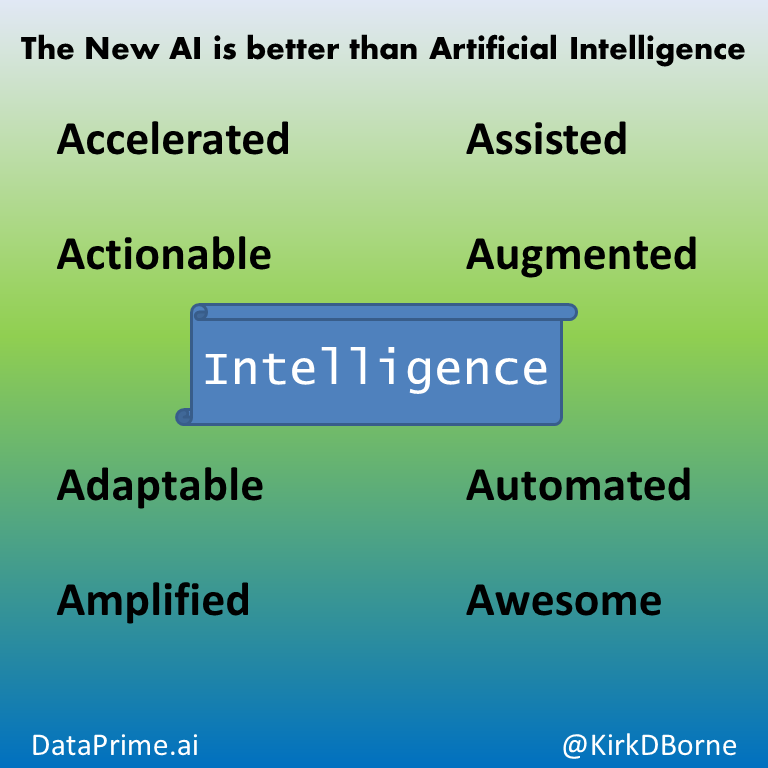
In the context of digital transformation and innovation, there is no lack of “hot topics” to discuss. Emerging technologies are truly emerging everywhere. What is most exciting – and what demonstrates their greatest promise – is that these new technologies are converging to produce innovative new businesses, products, and services.
Over the past decade, we have watched the blossoming of Big Data Analytics and Data Science, Machine Learning (ML) and Artificial Intelligence (AI), Internet of Things (IoT) and Industrial IoT (IIoT), Autonomous Systems (vehicles, drones), Augmented Reality (AR) and Virtual Reality (VR), Metaverse (immersive mixed reality environments), Digital Twins, Blockchain, 5G/6G, Deep Learning, Computer Vision, Conversational AI (virtual assistants, ChatGPT), and Quantum Computing. We will focus here on only a few of those.
From Data to Magic
Data comes from sensors, measuring and monitoring the states and behaviors of people, products, and processes. There is a massive amount of data streaming from billions and billions of sources. We don’t need to explain that to this audience. Data-collecting sensors are ubiquitous, both large and small, both external and embedded, both personal (wearables) and industrial. Sensors in the IoT and IIoT deliver digital data (and insights) in abundance.
ML algorithms discover and learn patterns in streaming data. Applying these algorithms to processes, actions, and machines leads us to implementations of AI, including robotics and autonomous intelligent systems. We can secure and/or encrypt the data flows from sensors to AI operations via Blockchain technology. When those AI operations become time-critical, we can greatly speed up the essential data rates (bandwidth) via 5G and 6G networks. Ultra-Reliable Low-Latency Communication (URLLC) is moving from an operational requirement in autonomous enterprise systems to an operations reality [1].
One such implementation of these converging technologies is in the platooning of autonomous vehicles, which drive in tight formation, synchronously, thereby vastly improving traffic flow and traffic safety. When people see test runs of platooning on the road [2], they might be startled by the precision and tight coordination of the driving – it is almost like magic. This is an example of what famous science author Sir Arthur C. Clarke meant when he said that any sufficiently advanced technology is indistinguishable from magic. So, the great magic that is now being revealed to us comes through the convergence of the current flood of emerging technologies.
Using Machine Learning to Turn Data into Insights
Measurements of things are collected as raw data by IoT sensors. That data then flows from the sensors (often through 5G/6G wireless networks) as “sensory” inputs to other things (devices, machines, computer logic) and to people (individuals and enterprises). Data-fueled ML algorithms then provide the power to drive amazing AI applications.
How does ML do this? ML represents a class of mathematical algorithms that learn from experience. This means that the algorithms learn the patterns, trends, segments, behaviors, what’s normal, and what’s abnormal in a system via the data emanating from that system’s sensors. The exploratory, investigative, and hypothesis-driven discovery of new insights from data using ML algorithms is called data science. The products of these activities are the analytics, which bring our data to life and put our discoveries into action for value creation.
AI is the application of ML in cyber-physical systems to create action, to make decisions, and to steer resources in response to signals from sensors. I think that we can now agree that enterprise AI is best understood not as “Artificial” intelligence, but as something much more practical and real: Accelerated, Actionable, Adaptable, Amplified, Assisted, and Augmented intelligence. That includes both human-assisted machine-based actions and machine-assisted human actions. That’s the real power of AI.
In many application areas where we are using sensor (IoT, IIoT, device) data and ML algorithms, it is possible for the AI to emulate the human cognitive abilities of foresight and optimization. Specifically, ML enables predictive modeling (forecasting) and prescriptive modeling (optimization) of systems’ behaviors and outcomes for optimal operating decisions and actions. In the wireless industry, examples of this include forecasting (predicting) loads on networks and optimized (prescriptive) load balancing.
The exciting role that IoT and IIoT streaming data play in these use cases and applications is to allow the insertion of multiple, diverse, and even “third party” data sources into the data-fueled and ML-powered decision processes. Those additional “external” data sources provide rich context to the signals coming from the primary systems. Since we anticipate that much of those contextual data will come from ubiquitous devices and sensors, the IoT might be re-labeled as the “Internet of Context” for AI applications.
Given the ability of rich contextual data to deliver multi-perspective insights, even an “ability to see around corners”, I have sometimes envisioned an API-accessible platform for IoT data coupled with ML predictive algorithms that can deliver Forecasting-as-a-Service to other enterprise processes, systems, and applications.
Ultimate Business Outcomes at the Edge
Consequently, predictive and prescriptive AI is driven by three categories of data: (1) historical (training) data, (2) real-time streaming data; and (3) independent co-occurring contextual data. We thereby are able to build better models based on such enriched data sources. For example, we will not simply base a forecast on what is the past history of network load as a function of time and the network’s current state, but we will also seek out and discover contextual events that were occurring at the specific times of historical network peak loads or previous anomalous network behaviors, such as social events, pandemic conditions, economic activities, sporting events, weather patterns, or any other patterns in streaming data from relevant sensors.
Applications at the convergence of emerging technologies offer the promise of novel businesses, products, services, and outcomes, which are realized through ubiquitous streaming data sources at the edge, which are processed and analyzed by edge computing, thereby creating an Internet of Context for digital transformation and AI innovation across the whole enterprise. See the following article for additional perspectives on this topic: [3].
References:
[1] “Ultra-reliable and low-latency communications: applications, opportunities and challenges” https://link.springer.com/article/10.1007/s11432-020-2852-1
[2] “What is Vehicle Platooning?” https://www.drivingtests.co.nz/resources/what-is-vehicle-platooning/
[3] “The Convergence of 5G, Artificial Intelligence, Data Analytics, and Internet of Things” https://blog.marketresearch.com/the-convergence-of-5g-artificial-intelligence-data-analytics-and-internet-of-things