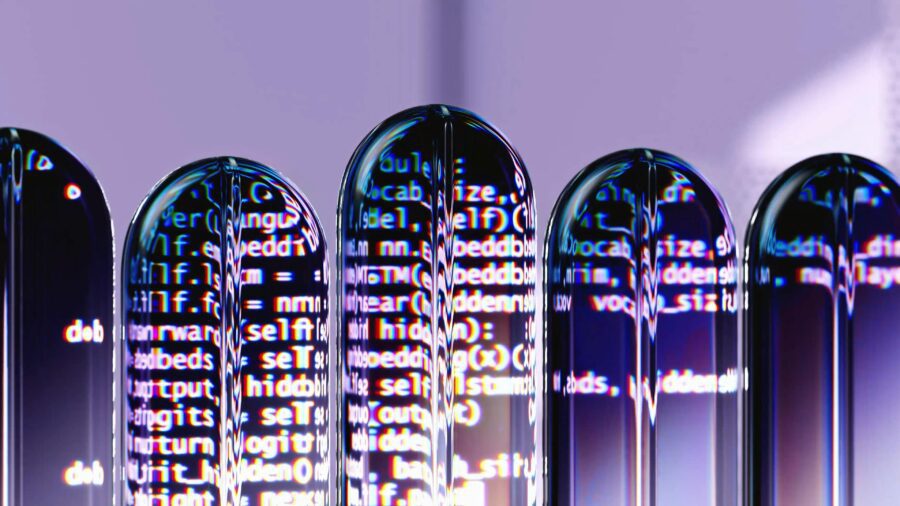
Harnessing artificial intelligence to manage vulnerabilities opens the door to efficient threat detection and proactive risk management.
Organizations of all sizes stand to benefit when they look beyond traditional methods, so here’s the lowdown on AI-focused strategies that are worth adopting today.
Integrating AI to enhance vulnerability management
There’s no excuse for being behind the curve on CVE trends, and there are a number of resources to track security vulnerabilities that apply to specific vendors like Intel and others. This process is a lot less of a struggle when AI is brought to bear on it.
Key strategies include:
- Automating routine tasks such as patch management and system updates
- Using machine learning algorithms to identify patterns and predict future vulnerabilities
- Implementing real-time monitoring systems for instant threat detection
- Incorporating AI-driven risk assessment tools for better decision-making
The shift towards AI in cybersecurity involves adapting these strategies into existing frameworks. This requires understanding both the technology’s capabilities and its limitations.
Businesses can leverage data insights to proactively address potential threats. They can then optimize resources by focusing efforts where they are most needed.
Integrating these approaches creates a rigorous defense against cyber threats while improving response times.
Adopting machine learning models for identifying weaknesses
Machine learning models are increasingly important for detecting vulnerabilities with precision and speed. They analyze vast amounts of data, enabling cybersecurity teams to identify threats more efficiently.
For instance:
- Training algorithms on historical security breach data helps to recognize potential risks
- Using anomaly detection is a means of spotting unusual behavior patterns in network traffic
- Employing classification techniques lets you differentiate between benign and malicious activities
- Continuously updating models with new threat intelligence allows for improved accuracy
These models adapt over time, refining their detection capabilities as they process more information. This dynamic approach helps organizations stay one step ahead of cybercriminals.
In addition, the implementation of machine learning irons out the kinks in the identification process reduces false positives, and enhances overall security posture. Organizations can then focus resources on addressing confirmed threats rather than chasing false alarms.
Predictive analytics in preventing security breaches
Predictive analytics offers a proactive approach to vulnerability management by forecasting potential security incidents before they occur. This method relies on data-driven insights to strengthen cybersecurity strategies.
Effective tactics include:
- Leveraging historical breach data and patterns to predict future attacks
- Applying statistical models that estimate the likelihood of vulnerabilities being exploited
- Utilizing scenario analysis for assessing possible threat outcomes and impacts
- Integrating real-time data streams for timely updates on emerging risks
Analyzing trends and predicting threats in this way means organizations can prioritize their security efforts more effectively. This leads to faster responses, minimizing damage from potential breaches.
Also, implementing predictive analytics enhances situational awareness, providing valuable foresight into an organization’s risk landscape. It allows teams to develop tailored defense mechanisms against likely attack vectors.
With these insights, businesses can allocate resources wisely while maintaining a strong defense posture against malicious actors of all types.
Synergizing AI and traditional security measures
Combining artificial intelligence with traditional security practices strengthens an organization’s defense capabilities. This synergy allows for comprehensive vulnerability management.
The aim here is to:
- Enhance existing security protocols with AI-driven threat detection systems
- Augment human expertise by using AI to process data at scale, identifying hidden threats
- Implement layered security models that combine both proactive and reactive measures
- Use AI analytics to refine traditional methods like firewalls and intrusion detection systems
This integration ensures that conventional strategies benefit from the speed and precision of AI technology. It enhances their effectiveness in mitigating risks.
Moreover, this collaborative approach supports continuous improvement in cybersecurity practices.
As threats evolve, organizations can adapt quickly by leveraging both modern tools and time-tested techniques. With even a single breach costing upwards of $9.36 million to recover from, it’s about financial stability as much as anything else.
Wrapping up
With AI-driven strategies in place, vulnerability management is less of a chore and cyber threats can be minimized with greater efficiency. Moreover, combining AI technologies with traditional security measures lets businesses create a dynamic defense system that’s easier to adopt today.
Implementing these approaches strengthens cybersecurity and also enhances operational resilience. As technology continues to advance, staying proactive ensures that companies remain secure and adaptable.