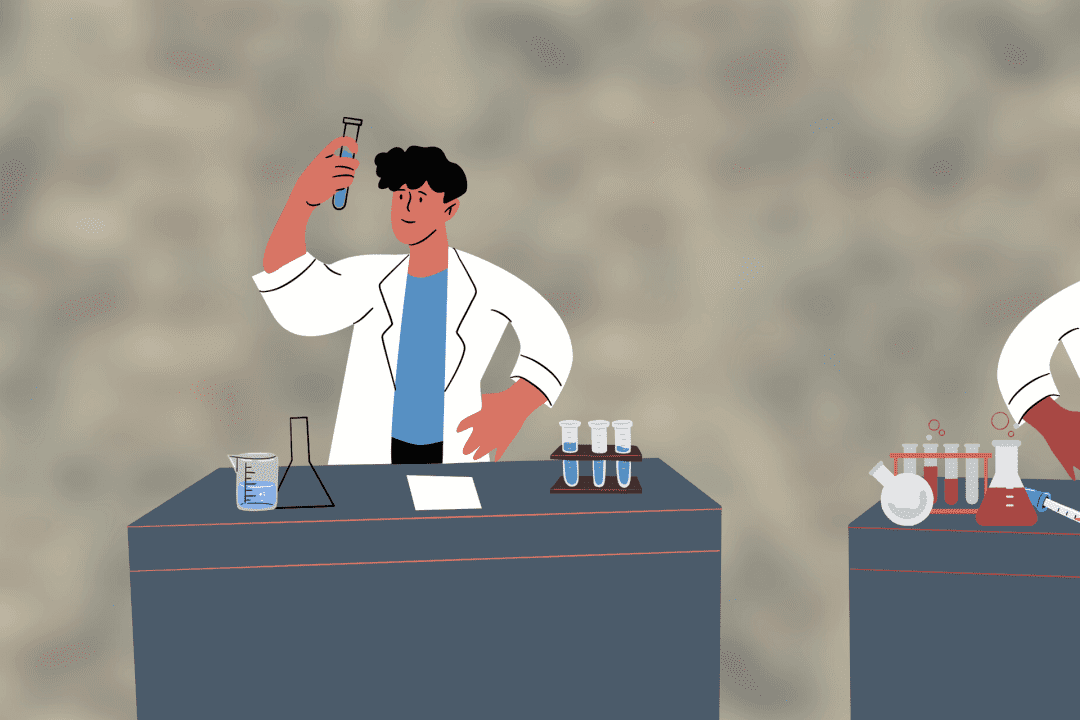
It’s that time of the year again, and I have a prediction.
We could see the rise of a low-code data scientist based on current low-code updates, increased demand for data science skills, and also the incorporation of generative tools like chatGPT into low-code tools.
Let me expand more.
The idea of low code itself is not novel – think of CASE tools, 4GGL, ABAP(SAP), etc. Low-code features include drag-and-drop user interface, Model-based development, Pre-built templates, Cross-platform accessibility, and better scalability and security. It’s targeted at citizen developers, but also traditional developers.
General acceptance from the analysts as a trend,
- Low Code Development Platform Market Size is projected to reach USD $148.5 Billion by 2030, growing at a CAGR of 27.8%: Straits Research. Growth The global low code development platform’s market size was worth USD $16.3 billion in 2021. It is estimated to reach an expected value of USD $148.5 billion by 2030, growing at a CAGR of 27.8% during the forecast period (2022–2030). North America accounted for the largest market share during the forecast period.
- Gartner predicts that 65% of all application development will be done with low code by 2024 and that 66% of large companies will use at least four different low-code application-building platforms.
Now, let’s look at AI.
Could there be a new role as a Low-code data scientist?
Let’s consider the types of data scientists
Commercial data scientist (ex: working in applications like price comparison and fraud detection)
Healthcare data scientist (emphasising explainable, responsible AI)
Research data scientist (ex from Deepmind, Oxford, Cambridge, MIT who push the boundaries of AI)
Engineering data scientist(combining physics-based modelling and simulation with ML)
Business intelligence data scientist (familiar with Cognos, Business objects, End-user, typically still working with structured data)
Data scientists with a Statistics background: more familiar with parameterised ML models and frequentist thinking and non-generative models
Now, consider that low code, when applied to AI has some advantages and could create a new category to the above list
- skills: this is the biggest reason. AI skills are expensive and low-code AI platforms could mitigate this
- integration: Many low-code AI platforms integrate with other development tools such as data validation and MLOps. This could save development time
- cloud: Low code development platforms could leverage the power of the cloud
- automl: low code development platforms could use automl to help build models faster
- access to pre-trained models: you could easily access pre-trained models and build on top of these
- security and privacy: you could benefit from the security and privacy of existing platforms
- scalability: finally, low-code solutions could be scalable.
- End-to-end benefits: Visual development: AI model building and training, AI workflow, Easier evaluation metrics, Easier feature engineering, Integration (database mlops etc), adoption of new technologies, process automation
Especially with access to new models like chatGPT which could complement tools – we could see a new category of a low code data scientist.
Wish you a Merry Christmas and Happy holidays!
Image source: https://pixabay.com/illustrations/laboratory-chemistry-scientists-7012275/