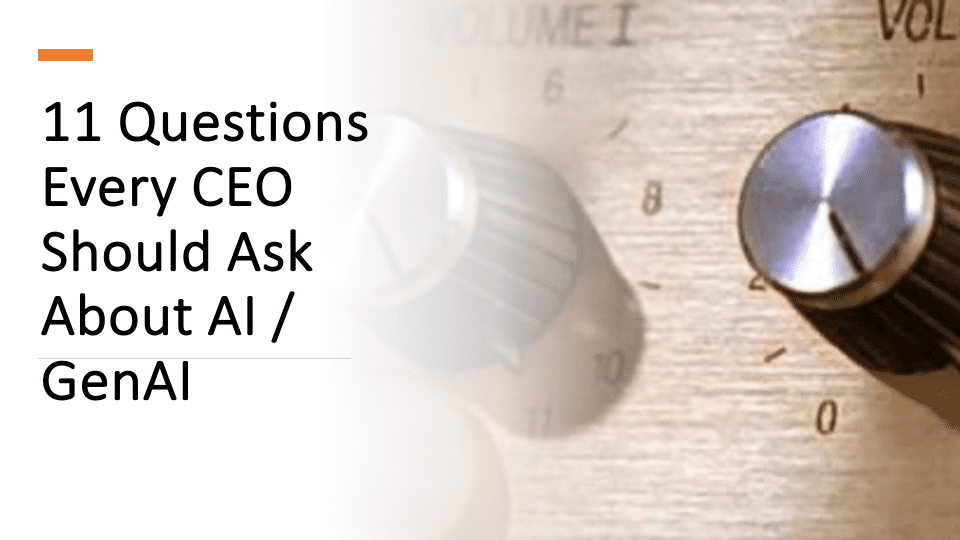
I’ve been in this industry for over 40 years (yes, I just started in the data and analytics industry when I was 11), and I have NEVER seen anything like Artificial Intelligence (AI) and Generative AI (GenAI) capture the attention of CEOs (and the dystopic fear of everyone else). Is AI a game-changer? Definitely! Will it impact all organizations equally? An unequivocal NO! There will be:
- AI Leaders: These organizations are at the forefront of AI adoption and are leveraging AI to create significant value for their stakeholders. They have a clear understanding of how AI can be used to deliver meaningful, relevant, responsible, and ethical outcomes. They foster a culture of curiosity, innovation, collaboration, and experimentation, which enables them to capitalize on AI’s value creation and cultural empowerment capabilities. They have a comprehensive plan to enable all the organization’s value-creation capabilities and exploit the economics of data and analytics.
- AI Followers: These organizations have deployed AI in targeted business areas (e.g., fraud detection, credit risk management, campaign optimization, predictive maintenance) and achieved impressive results. However, they lack a holistic plan to enable all the organization’s value-creation capabilities. They struggle with cross-organizational challenges such as misalignment, fragmentation, inconsistency, or inefficiency in institutionalizing the value-creation potential of AI and data. They need to invest in building organizational capabilities to exploit AI fully.
- AI Laggards: These organizations are skeptical of AI and lack the management fortitude to invest in and empower the organizational capabilities to exploit AI. They are overwhelmed by the challenges associated with responsible AI deployment, such as data quality, security, personal privacy, confidentiality protection, and ethics. They are unprepared to meet the hard cultural and organizational requirements for deploying AI effectively. Their infatuation with AI will dissipate as they realize the overly grand promises of AI gloss over the challenges associated with its deployment.
These segments should not be any surprise because we’ve seen this segmentation before from a 2020 study by Kearney that highlighted the profit impact between analytics leaders and the huddled masses (Figure 1).
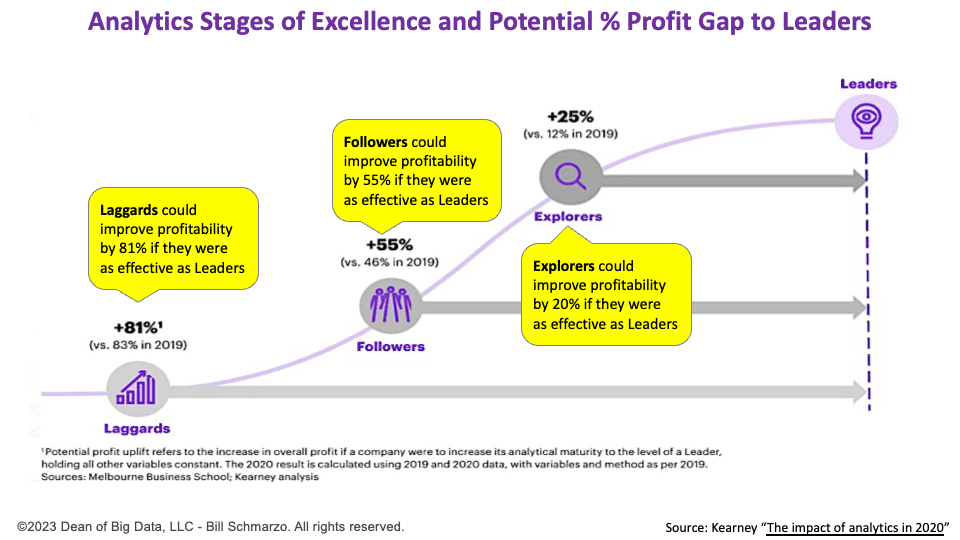
Figure 1: Costs of Being an Analytics Laggard…And Path to Becoming a Leader
I loved that this study put a number on the potential cost (profit impact) of being a second (or third or fourth) mover on the analytics opportunity:
- Explorers could improve profitability by 20% if they were as effective as Leaders
- Followers could improve profitability by 55% if they were as effective as Leaders
- Laggards could improve profitability by 81% if they were as effective as Leaders
Yeah, this is not small potatoes in getting AI and analytics right from a business viability and survival perspective!
11 Questions Every CEO Should Be Asking of their AI Strategy
To ensure that your organization is among the AI leaders (and not an AI pretender), your CEO should start by asking the following questions (and because this is critically important to the organization’s survival, we had to dial it up to 11 questions).
1. Lead with Value. Is our AI strategy being driven by and aligned with our business strategy?
Successful organizations start their AI strategies by clearly linking to how they create value. This includes identifying ideal outcomes, capturing key internal and external stakeholder decisions, and identifying a broad range of KPIs and metrics (e.g., financial, operational, customer, employee, partner, community, environmental, and ethical) against which the stakeholders will measure outcomes and decision effectiveness.
Once these (and a few other criteria) are known, the organization is not positioned to create an AI strategy that supports the organization’s value-creation processes. The organization can start envisioning and exploring where and how AI can improve and optimize use case outcomes and decision effectiveness.
2. Organizational Alignment. Does everyone understand their role and responsibilities in supporting the organization’s value-creation processes?
It is essential that all stakeholders have a clear understanding of the organization’s key business initiatives and how their roles and responsibilities align with those business initiatives. That is, leadership needs to ensure that everyone has a clear line of sight from what they do to how that creates value for the organization’s stakeholders.
Establish a clear connection between AI initiatives and the organization’s broader business goals to ensure that AI efforts are aligned with the organization’s objectives.
This requires clear and consistent communications to ensure that each employee understands the KPIs and metrics against which their value creation effectiveness will be measured. If this sounds like OKRs, then you’re heading in the right direction.
3. Business-driven Collaboration. Is the business equally invested in creating and maintaining the AI strategy?
Business stakeholders can’t just be involved at the “requirements definition” stage of the AI journey. The Business stakeholders must be heavily invested throughout the AI journey to ensure that the data science work delivers meaningful, relevant, responsible, and ethical AI outcomes.
This ongoing collaboration is critical throughout the journey, including 1) providing critical details about the targeted business initiative, 2) identifying, validating, valuing, and prioritizing the use cases that support the business initiative, 3) brainstorming the features that might be better predictors of behaviors and performance when building the analytics, and 4) determining when the AI model is “good enough” given the costs associated with making the wrong decisions.
4. AI & Data Literacy. Have we educated everyone on the potential and dangers of AI?
Educate all your employees and constituents about AI’s potential benefits and risks to ensure that they understand how AI can be used effectively and responsibly. This includes basic training in decision-making, foundational training in the different types of analytics, and how to apply basic stats to improve decision-making effectiveness.
Organizations can help prevent unintended consequences such as bias, discrimination, or privacy violations by promoting awareness of ethical considerations and responsible use of AI technologies. Providing training sessions or workshops on AI can help employees develop the skills necessary to work with AI technologies effectively.
5. Empowered Frontlines. Have we engaged the folks who will use the AI models’ outputs to develop the models – the frontlines of customer engagement and operational execution?
Involve your end-users and subject matter experts in the AI model definition, design, development, and ongoing management processes to ensure that the models meet their needs and expectations. Involving subject matter experts throughout the process ensures that the models are designed to address relevant business challenges and will actually be used by the people for whom the analytics were developed.
Also, create a continuous process for gathering user feedback to identify improvement areas and ensure that the models deliver relevant, meaningful, responsible, and ethical outcomes.
6. Assess Performance. How do we monitor and evaluate the performance of our AI models over time?
Establish key performance indicators (KPIs) against which to measure the ongoing effectiveness of AI models. Implement monitoring and observability processes to track model performance, identify anomalies, and trigger corrective actions to ensure that models remain effective and relevant over time.
Additionally, by regularly evaluating model performance, organizations can identify areas for AI model and operational improvement and take corrective actions as needed.
7. Integrated Learning Loop. Have we developed a feedback loop to ensure AI models continuously learn and adapt?
Implement mechanisms to collect model effectiveness feedback and incorporate that feedback into model updates to ensure that the organization is that the AI models continuously learn and adapt. Regularly evaluate model performance and iterate on model and process improvements based on real-world usage to ensure that models remain effective as the operational environment changes.
By the way, by incorporating user feedback into model updates, organizations can help improve user satisfaction with AI technologies.
8. Confidentiality Leakage. Do we have education and processes to ensure our confidential data isn’t accidentally divulged?
Educate employees about data privacy best practices, including handling sensitive information, to prevent accidental disclosure of confidential data. This includes implementing robust security measures such as access controls and encryption, which can help protect confidential data from unauthorized access or disclosure.
Organizations can minimize the risk of data breaches or other security incidents by establishing processes for handling confidential data effectively.
9. Unintended Consequences. Do we have a process for identifying an AI model’s potential unintended consequences?
Conduct thorough risk assessments to identify potential unintended consequences of AI models. This is crucial in preventing unintended negative impacts on business outcomes or stakeholders. This means establishing all-inclusive formal processes for ideating, addressing, and mitigating any identified risks can help prevent unintended consequences from occurring.
Additionally, by regularly reviewing risk assessments and updating protocols as needed, organizations can ensure that they remain prepared for any potential risks associated with their AI initiatives.
10. AI Model Transparency. Do our AI models provide the transparency to withstand legal scrutiny?
Ensure that your AI models are explainable, meaning humans can understand the factors that drove an AI model’s recommendation or decision. Documenting model development processes and preserving documentation for legal compliance can help support transparency in model development.
Also, organizations can minimize legal risks by ensuring that AI models comply with relevant national and local regulations and standards.
11. Mitigate Biases. How do we ensure our AI models are unbiased, discrimination-free, and minimize confirmation bias?
Conduct thorough data assessments to identify potential sources of bias in training datasets. Implement techniques such as data augmentation, adversarial training, and fairness constraints to reduce bias in AI models. Conduct regular reviews for bias and take corrective actions when biases are identified to ensure that models remain unbiased over time.
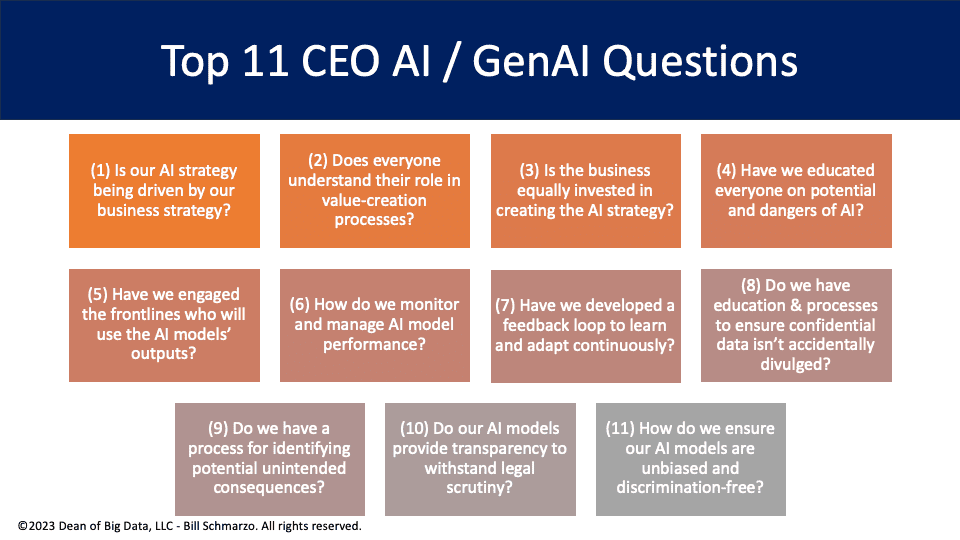
Figure 2: Top 11 CEO AI /GenAI Questions
Top 11 CEO AI / GenAI Questions Summary
“Toto, I’ve a feeling we’re not in Kansas anymore.” – Dorothy in the “Wizard of Oz”
The AI age is upon us, and things aren’t returning to how they were before. A new type of leadership is required to corral and exploit this “Artificial Intelligence” phenomenon. We need strong, stable, inclusive, and accountable leadership with a clear vision for how the organization can leverage AI to deliver meaningful, relevant, responsible, and ethical business and operational outcomes. That is:
- Meaningful: Improves society and people’s lives by reducing poverty, enhancing health, education, and employment opportunities, and protecting the environment.
- Relevant: Pertinent to you and me as individuals in providing individualized solutions, recommendations, and insights that match our unique situations and aspirations.
- Responsible: Considers the potential ramifications for others, especially those who may be harmed or excluded by AI decisions or actions.
- Ethical: Upholds the principles of fairness, justice, and reciprocity by ensuring that AI respects human dignity, diversity, and equality and does not cause harm or discrimination.
It’ll take an empowered organization to uncover, align, and deliver on the value-creation potential of AI. And that transformation starts with the CEO asking hard questions about the organization and enabling culture they are trying to nurture.